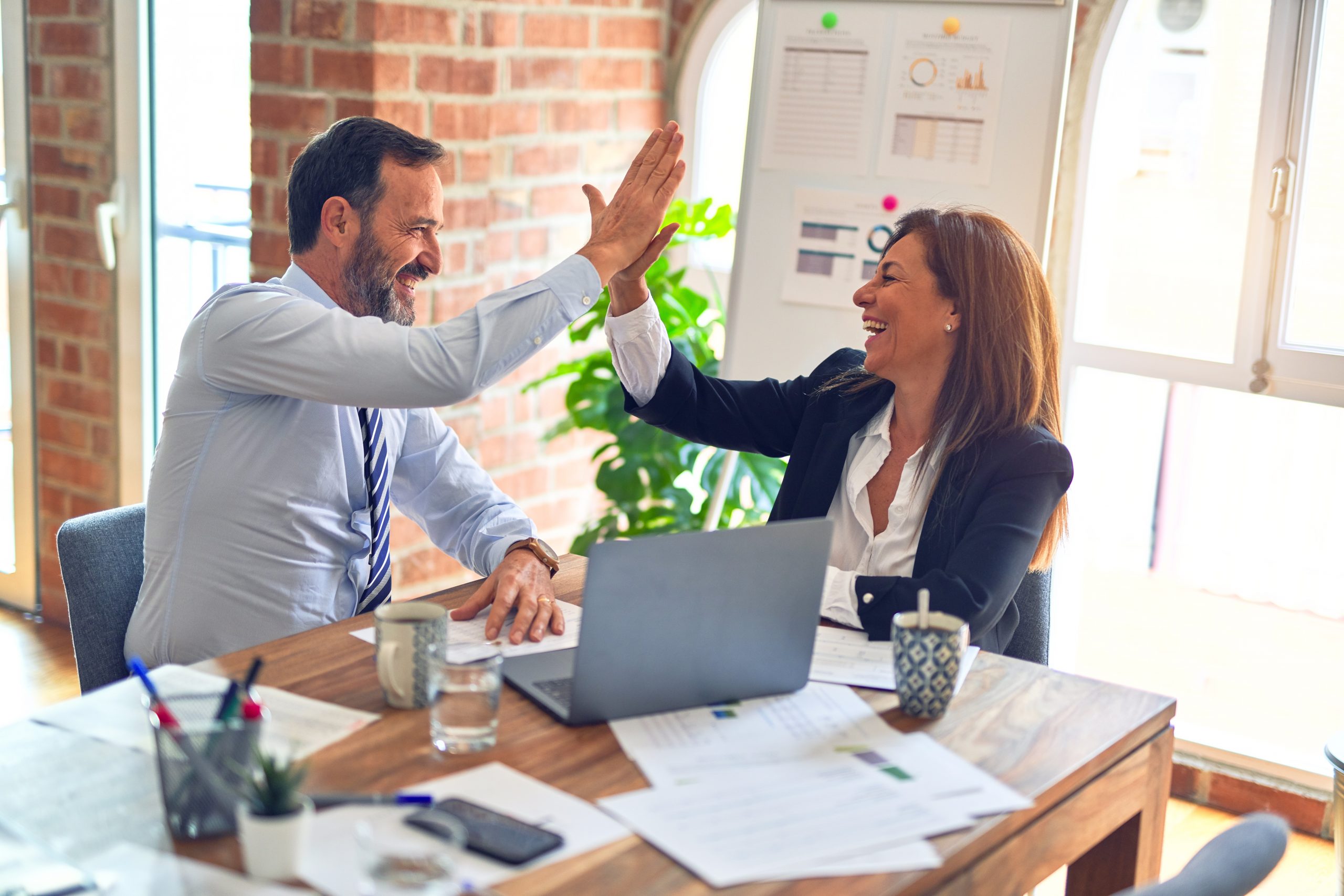
The survey is one of the most important data collection tools in the armament of a Six Sigma practitioner. There is no lack of research literature on the principles and designs of effective surveys. While the surveys conducted by academics and certain research institutes often reflect impeccable design, there are innumerable cases in which the results of survey conducted in haste are not accepted due to poor design. Conducting a survey during a Six Sigma project can be a daunting task. Rigid timelines often lead to poorly designed surveys, which lead to rejection of the results.
This article provides a brief overview of the intricacies involved in a survey design – without getting into complex statistical theories.
Designing a survey is an iterative process as shown in Figure 1.

Measuring the Construct
The critical aspects of any survey design are the underlying construct, framing of questions, validity and reliability, and the sampling methodology. A survey is done to measure a construct – an abstract concept. Before designing a survey, the construct must be clearly defined. Once the construct is clear, it can be broken down into different dimensions and each dimension can then be measured by a set of questions.
Consider an example of a human resources (HR) department that is trying to study the attitude of employees toward a newly launched appraisal process. Assume that with some research, the HR department finds that the major dimensions of the attitudes toward the appraisal policy are “transparency,” “evaluation criteria,” “workflow” and “growth potential.”
Framing the Questions
After determining the dimensions, a set of questions needs to be written to measure said dimensions. Questions can be categorized into two groups: classification and target. Classification questions cover the demographic details of the respondent, which can be used for grouping and identification of patterns during analysis. Target questions refer to the construct of the survey. Table 1 includes tips for avoiding common mistakes while wording the questions and selecting their order.
Table 1: Tips for Question Development and Ordering | ||
Content | Wording | Sequencing |
1. The question must be linked to a dimension of the construct.
2. The question should be necessary in the manner that it helps decision maker in making a decision. 3. The question should be precise and should not seek multiple responses. 4. The question should contain all information to elicit an unbiased response. 5. The question should not force the participant to respond regardless of knowledge and experience. 6. The question should not lead the respondent to generalize or summarize something inappropriately. 7. The question should not ask something sensitive and personal that the respondent may not wish to reveal. |
1. The question should not include jargons, technical words, abbreviations, symbols, etc. Use simple language with shared vocabulary.
2. The question should be worded from respondent’s perspective and not researcher’s perspective. 3. The question should not assume prior knowledge or experience inappropriate for the given situation. 4. The question should not lead the respondent to provide a biased response. 5. The question should not instigate the respondent by using critical words. |
1. The target questions should be asked at the beginning followed by classification questions at the end.
2. The questions should be grouped logically. Under each group, the wording and scale should be similar. 3. Complex, sensitive and personal questions should not be asked at the beginning. |
Response Format
Another important aspect of a survey questionnaire is the response format. There are two levels at which the questions can be classified with regard to response format: structured and unstructured (Figure 2).

Structured questions provide close-ended options for the respondent to choose from, while unstructured questions provide a free choice of words to the respondent. While structured questions are easy to analyze, the provided choices must be mutually exclusive and collectively exhaustive. Unstructured questions, on the contrary, are difficult to analyze – limit them in the questionnaire. Structured questions can further be classified based upon a measurement scale. The choice of the measurement scale depends upon the objective of asking the question and, in turn, influences the analysis and interpretation of response. Table 2 describes difference types of measurement scales, characteristics of data generated through them, their purpose and their impact on analysis.
Table 2: Questions Defined by Measurement Scale | |||
Scale | Characteristics of Data | Purpose (When to Use?) | Implications on Analysis (Limitations) |
Nominal | Discrete data with no sense of magnitude (can be binary or multinomial) | · Classification of certain characteristic, event or object
· Can be dichotomous (only two choices) or multiple choice |
· Only mode can be calculated as measure of central tendency
· Cross tabulation and Chi Square can be used for analysis · Arithmetic operations are not possible on nominal scale |
Ordinal | Discrete data with a sense or order/rank | Involves rating or ranking a particular factor on a numeric or verbal scale where distance between various alternatives is immaterial | · Median, quartile, percentile, etc. can be used for central tendency
· Various nonparametric tests can be used for analysis · Arithmetic operations are not possible |
Interval | Continuous data with a sense or order, and distance | Involves rating a particular factor on a numeric or verbal scale where distance between various alternatives is important
· A Likert scale is a type of interval scale with a neutral value in between and extreme values at both end |
· Mean or median can be used as measure of central tendency depending upon the skewness of the data
· Parametric tests such as t-test, F-test, ANOVA, etc., can be used · All arithmetic operations are possible except multiplication and division |
Ratio | Continuous data with a sense or order, distance and origin | Involves questions pertaining to specific measurements such as “number of incidents per month” | · All statistical techniques are possible on data generated by ratio scale
· All arithmetic operations are possible |
Evaluating Validity and Reliability
After designing the questionnaire, the next step is to establish its validity and reliability. Validity is the degree to which a survey measures the chosen construct whereas reliability refers to the precision of the results obtained. Table 3 provides a brief description of the considerations of validity and reliability, and how they can be evaluated. The table also states when a particular evaluation technique can be applied.
Table 3: Validity and Reliability | |||
Design Aspect | What and Why? | How? (Action Items) | When? |
Validity | Representational validity – the degree to which a construct is adequately and clearly defined in terms of underlying dimensions and their corresponding operational definitions. | Consider again the attitudes to the newly launched appraisal process; the representational validity can be established by getting the questionnaire objectively evaluated by HR experts. Face validity can be established by assessing the suitability of questions, their wording, order and measurement scale. Content validity can be established by assessing the adequacy of the dimensions and corresponding questions in measuring the attitude. | Before administering the survey |
Criterion validity – measure of correlation between the result of a survey and a standard outcome of the same construct | Criterion validity in the example can be established by comparing the score of attitude of an employee with his or her performance rating.
If the scores are compared to a current performance rating, concurrent validity has been established. Another part of criterion validity is predictive validity, which can be established only in the long run by comparing the survey results with long-term performance of employees. |
After the results are obtained | |
Construct validity – measure of extent to which the questionnaire is consistent with the existing ideas and hypothesis on the construct being studied | In the HR example, construct validity can be established by two means: 1) correlate the scores obtained with that of another survey on attitude toward appraisal (convergent validity) and 2) correlate the scores obtained with that of another survey on attitudes of employees toward the earlier appraisal policy (discriminant validity). By factor analysis, it can also be verified by whether the results support the theory-based selection of dimensions and the corresponding questions. | After the results are obtained | |
Reliability | Stability – measure of consistency of results obtained by administering the survey to same respondents repeatedly | In practice, it is difficult to establish stability. The main problem is the choice of interval for administering the survey again. It may result in respondents answering based on memory or confounding of results with actual change in the construct. It is recommended to decide on the choice of interval, taking into consideration all the factors that influence the construct. | After the results are obtained |
Equivalence – degree of consistency among the observers or interviewers | This form of reliability is more appropriate in interviews and telephone surveys. | After the results are obtained | |
Internal consistency – measure of consistency in the questions used to measure the construct | Internal consistency can be measured by dividing the questionnaire into two equal halves and measuring the correlation between their scores. | After the results are obtained |
Sampling Methodology
After the questionnaire is designed, it is time to determine the appropriate sampling methodology. Sampling is done to save time and costs in situations where it is not feasible to reach out to the entire population. It is a part of both analytical and enumerative studies. An enumerative study is done to measure the characteristics of population under study while an analytical study aims at revealing the root cause of the pattern observed. In other words, an enumerative study asks the question, “How many?” while an analytical study asks, “Why?” This distinction influences the sample design as well as the interpretation of the results. For an enumerative study, a random sample is repeatedly taken from a population while for an analytical study a random sampling frame is selected repeatedly from a population and then a sample is selected randomly from the sampling frame. During analysis and interpretation of results, scores of analytical studies include standard errors while those of enumerative studies do not.
Sampling can be broadly classified into two categories: random probability and non-probability. Random probability sampling means that each element of the population or sampling frame has an equal and non-zero probability of getting selected in the sample. It is only within the scope of random probability sampling that standard error (the measure of variation in the sample due to sampling error) can be estimated. Only in the case of random probability sampling can an estimate of confidence be made for a sample statistic. Here it is also noteworthy that as sample size increases, standard error reduces as the calculation of standard error uses sample size in the denominator. Non-probability sampling means that the sample is selected based upon judgement or convenience. It is important to keep the difference between random probability sampling and non-probability sampling in mind while selecting and implementing a survey methodology. Sometimes a random probability sampling methodology used to select elements of a population inadvertently leads to a selection of elements on a non-probability basis. Assume that a survey is conducted by a marketing team wherein the team members select people in various locations and ask them to complete the questionnaire. There can be bias resulting from the selection of respondents by the team members and respondents choosing not to respond. It is imperative that these situations are thought through while planning data collection before it comes to interpreting the results.
Refining and Administering the Survey
After deciding on the sampling methodology, the survey is administered. The survey is also reassessed in terms of validity and reliability and is further refined. Often, a survey is pre-tested before being rolled out on a large scale. This helps in refining the survey before it is launched. This, however, depends upon the available time and budget. In general, it is recommended to pre-test a survey. Once the results are received, the data is analysed and interpreted using various descriptive and inferential statistical techniques.
Conclusion
It is highly recommended for a practitioner to understand the nuances of surveys before embarking upon any such assignment. Refer to this article to help refine survey design in shorter and shorter spans of time.
Resources
- W. Edwards Deming. On the distinction between enumerative and analytical surveys. Journal of the American Statistical Association. 1953(48):244-255.
- José Linares Fontela. A Guide to Designing Surveys. Available at: https://www.woccu.org/documents/Tool10. Accessed September 4, 2017.
- W. Edwards Deming. Some Theory of Sampling. 1966. Dover Publications.
- Office of Quality Improvement, University of Wisconsin-Madison. Survey Fundamentals: A Guide to Designing and Implementing Surveys. Available at: https://oqi.wisc.edu/resourcelibrary/uploads/resources/Survey_Guide.pdf. Accessed September 4, 2017.
- Jon A. Krosnick and Stanley Presser. Questionnaire Design in Handbook of Survey Research, (Second Edition). 2010.
- Carole L. Kimberlin and Almut G. Winterstein. Validity and reliability of measurement instruments used in research. American Journal of Health-System Pharmacy, 2008(65);23: 2276-2284.
- Sofia D. Anastasiadou. Reliability and Validity Testing of a New Scale for Mesuring Attitudes Toward Learning Statistics with Technology. Acta Didactica Naposencia. 2011(4).
- Linda Del Greco, Wikke Walop and Richard H. McCarthy. Questionnaire development: 2. Validity and reliability, CMAJ. 1987(136);699-700.
- Wai-Ching Leung. How to design a questionnaire, STUDENT BMJ. 2001(9):187-189.