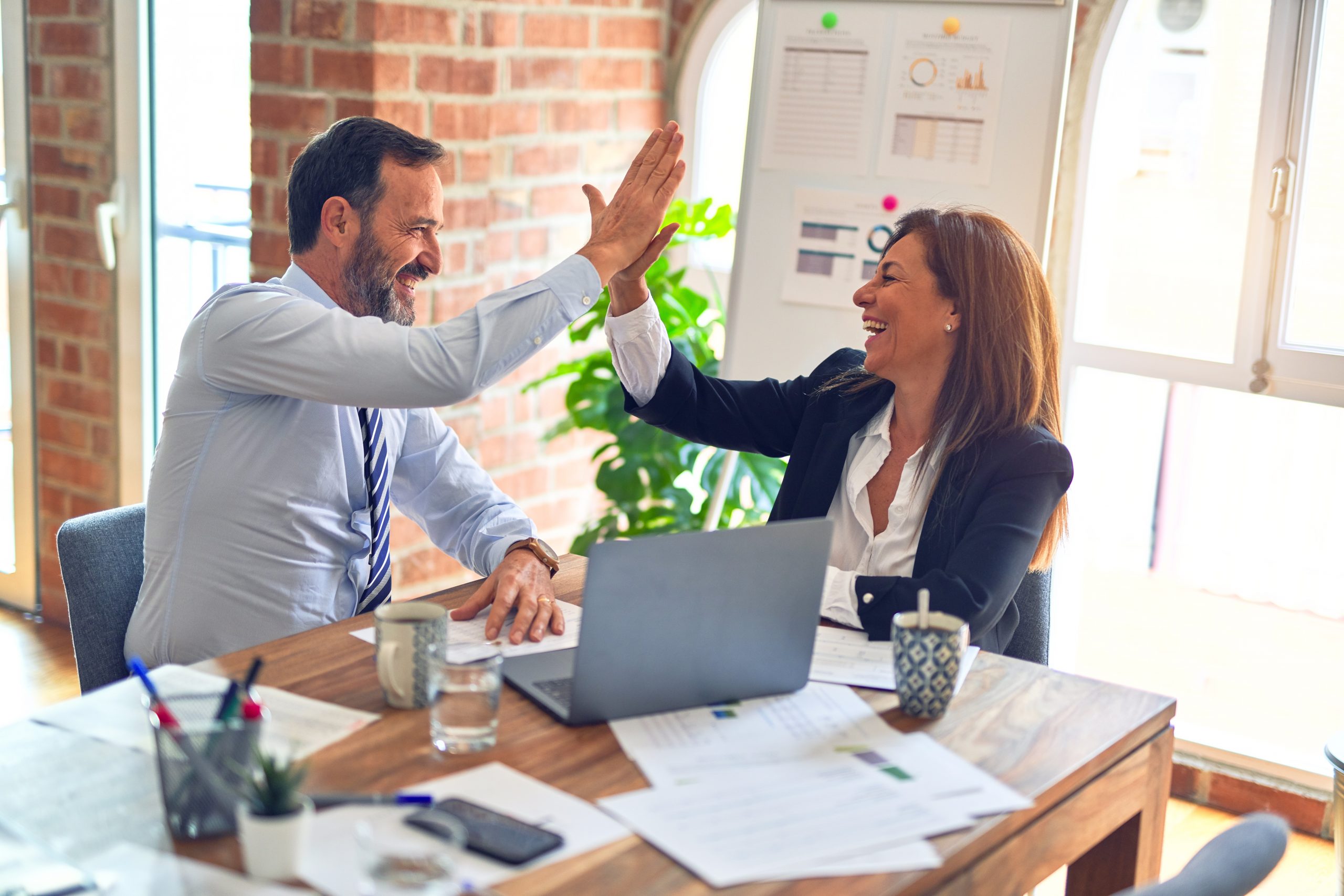
A number of useful ways exist for processing and using language data, among them pre-processing raw language data for specific uses, focusing on an efficient data sample and distilling the data for particular purposes using tools like net-touch, affinity diagrams or KJ analysis.
Pre-processing Raw Language Data
One problem inherent in language data is the volume of raw material necessary to mine the most useful information. When capturing voice of the customer (VOC) data it is helpful to first pre-process information to highlight context and needs data, as discussed in Part 1. Figure 1 illustrates this for a simple case. Extracting key phrases, while maintaining a clear and traceable link to their sources, is the first step in preparing the data for further distillation.
Figure 1: Pre-Processing Transcripts to Highlight Context and Needs Data |
Legend:
|
Focusing on an Efficient Data Sample
Even after pre-processing, there still may be too much data to readily distill. In these cases, further pruning of the data to an appropriate representative sample can save time and energy. For example, the KJ analysis method uses a high level of discipline that requires considerable time be spent on each language data element.
A Multi-Pick Method
What if pre-processing has yielded 100 or more remaining language data groups? A simple approach that works very well in practice is illustrated in Figure 2. The steps to this multi-pick approach include the following:
- On an empty wall chart, post the theme question that the team is trying to answer with language data. This provides an important focal point for deciding what’s most useful.
- Place each element of the data set on a separate self-stick note, arranging the notes on the empty chart.
- Team members read each note, considering whether or not that element should remain as part of the critical sample.
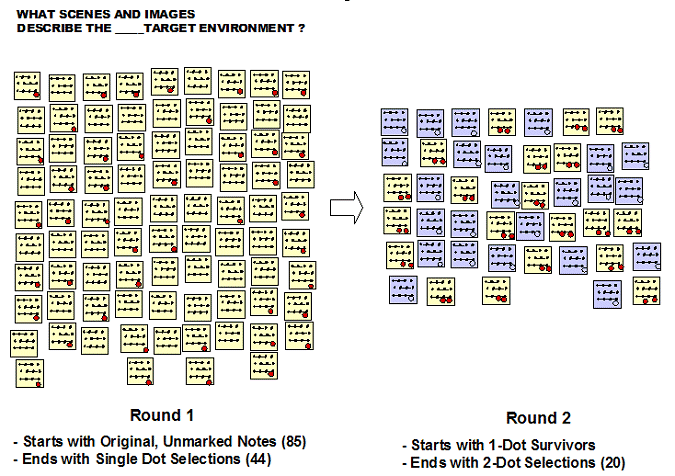
Net-touchÂ
Net-touch, a tool for distilling the data, uses a simple affinity process with a useful twist. In a routine affinity, everyone’s self-stick notes are posted on a wall and each is read by enough members of the team to begin the grouping process. In net-touch, everyone holds onto their own notes and watches the facilitator for cues to offer a note for grouping. A practical use for this process is during the building of an interview discussion guide. The process includes the following steps:
- Everyone in the group writes open-ended questions that address the VOC learning objectives
- People hold onto their own notes.
- A facilitator takes one note from the group, at random.
- Reading that note they ask, “Does anyone have a question that belongs with this one?”
- Because everyone is familiar with his/her own notes, this provides efficiency.
- The facilitator collects all the notes that are offered, forming a cluster with the original seed note.
- Repeat steps 3-6 until all the notes have found their way into a cluster.
- Now the team can work together to subdivide large clusters into smaller groups and title them.
KJ Analysis
KJ analysis is a powerful tool that is not widely used. Its founder, Jiro Kawakita, realized the simple yet profound value in the way that abstraction distills meaning, even in language and observational data that is incomplete. There are many kinds of KJs each distinguished by the theme question posed in its upper left corner (see Figure 3 and the example in Figure 4). It’s important to note that all KJs seek facts that answer the theme question. For that reason, you won’t typically see this method used for brainstorming. Part of the KJ discipline is the use of report language and the verification source data.
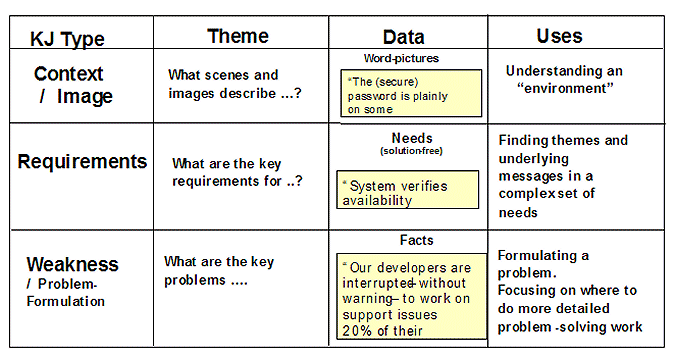
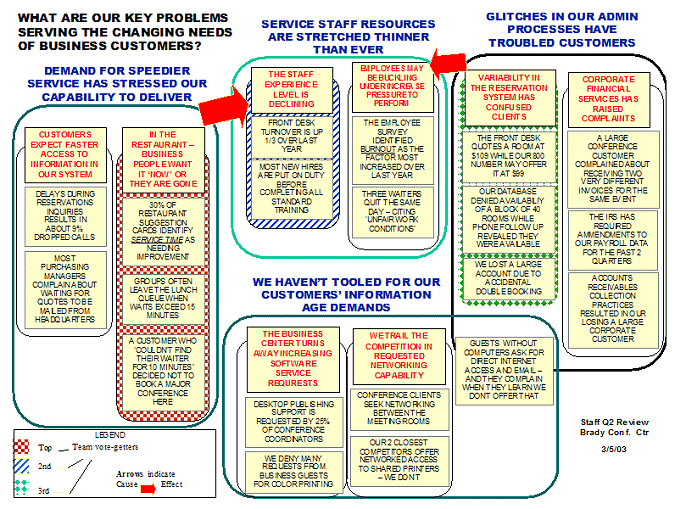
 KJ Analysis Steps
The best way to learn the KJ analysis method is to participate under the guidance of someone who knows and understands the practice. The steps outlined here provide some insight into the process.
- State the theme as a question (Figure 4). The theme carefully states what the team seeks to learn through the KJ analysis and facilitates the collection of factual answers.
- Gather facts that answer the theme question. A color-code convention suggests that these facts be printed on self-stick notes.
- Assemble the facts, reducing them to the key 20 to 30 if necessary. See the multi-pick method above and in Figure 2.
- Gain team understanding of each fact, scrubbing its language for clarity. This is a powerful step in the process when each fact is explained by the person offering it to create team understanding of the story behind the fact. Even if they stopped here, a team would probably have derived significant benefit by having come to understand one another’s facts and perspective on a complex issue.
- Group the facts that really belong together (3 per group, maximum). This is an important part of the discipline where notes are not grouped by keyword or logical grouping, but by the story they tell.
- Title the groups. Each piece of data in a KJ analysis is a complete sentence answer to the theme question. This is true of the titles created to describe each group at each level. Develop titles that are as specific as possible, while generalizing the stories being told by each of the notes. This follows the precise generalization mode of abstraction. In object-oriented design a common test of an abstraction hierarchy is that any item below is a reasonable substitute for an item above it.
- Encapsulate lower level facts under higher level titles. Simply hide the lesser notes under the more prominent items to simplify next-level grouping.
- Group and then encapsulate the titles again. Repeat the logic and operations of steps 5 and 7 and then use arrow and opposition symbols to identify cause and affect relationships.
Conclusion
The importance of understanding VOC through effective application of language data is clear. Six Sigma practitioners who use this data reap the rewards of combining the benefits of language data with number data to find project success.