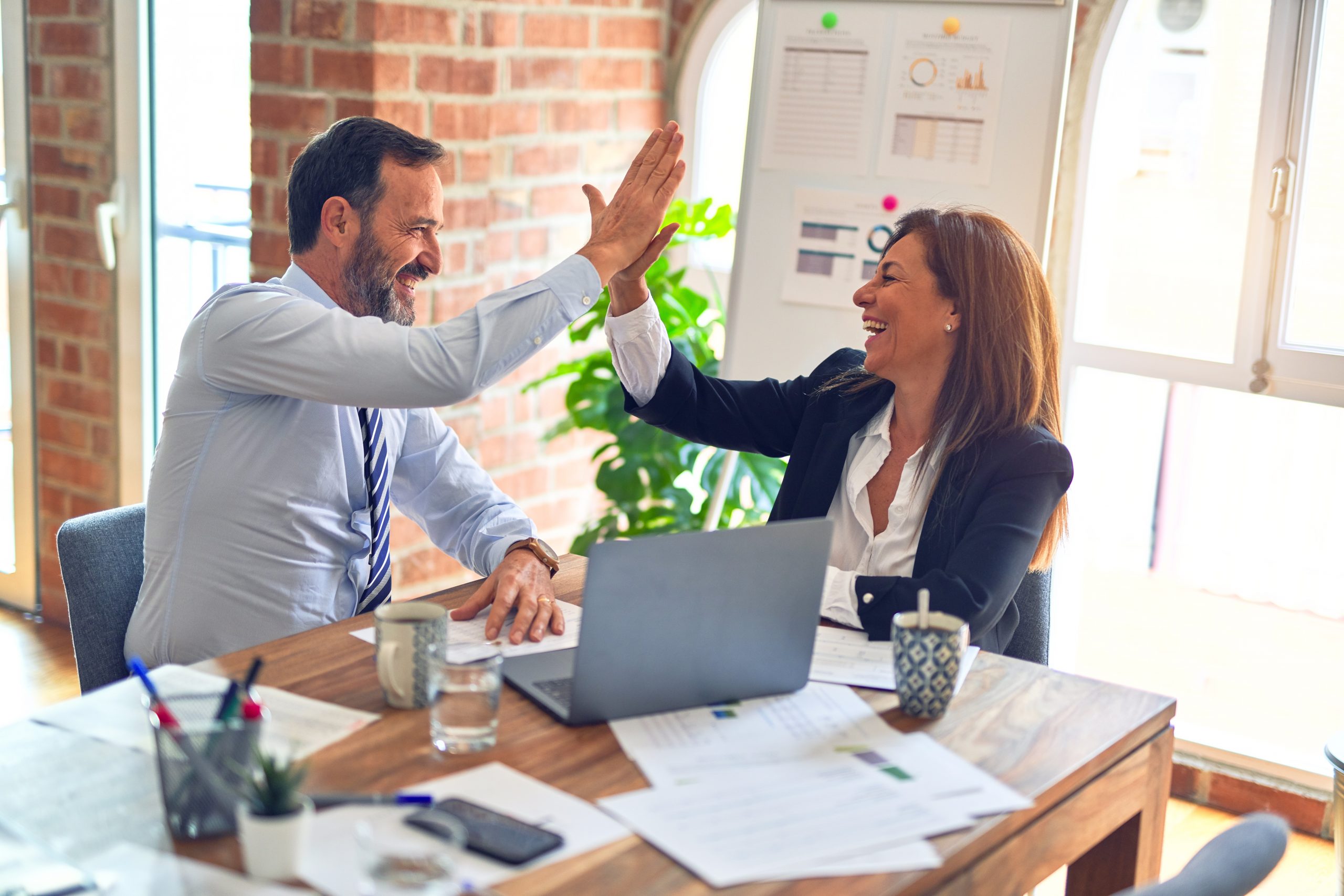
Statistical process control (SPC), despite sounding esoteric, is a subject that every process owner and worker should – and can – understand, at least at a high level. Knowing whether a process is in control and stable is paramount to producing a product or service that meets customer needs.
In this hour-long Minitab training course that took place in Seattle, Eduardo Santiago covers many useful topics related to SPC, including:
- The difference between common-cause and special-cause variation (1:28)
- Rational subgroups and why they are necessary when collecting data (8:30)
- Two separate phases for running control charts (30:00)
- Determining when a process is in control and out of statistical process control (31:00)
- The eight tests for identifying special causes of variation (36:27)
- Stages of statistical process control (56:47)
About Eduardo Santiago
Eduardo Santiago is a technical training specialist with Minitab.
Eduardo earned a bachelor’s degree in industrial engineering from CETYS-Universidad, one of Mexico’s premier educational institutions, and later earned an M.Eng. and a Ph.D. in industrial engineering and operations research from Penn State University, focusing on optimal design theory and how to collect the greatest amount of useful data in the fewest number of runs in expensive testing situations.
He earned his Six Sigma Green Belt certification from Sony while working as a quality improvement consultant for clients such as Plantronics, RCA Display, URBI Homes and Markofoam, a Sony supplier. Before becoming a consultant, Eduardo spent five years teaching mathematics, statistics, chemistry and physics. Eduardo also taught an undergraduate engineering economics course at CETYS University.
Follow Minitab on Twitter
Follow iSixSigma on Twitter
Minitab Data Sets
Color1 Data Set
Color2 Data Set
FilmDens Data Set
Improve Data Set
Eduardo Santiago Interview Raw (Non-Edited) Transcript
Eduardo Santiago Interview Transcript in PDF Format (Right-click to Save As…) [View in Google Docs]
Watch the full video at:
https://www.isixsigma.com/tools-templates/statistical-analysis/understanding-spc/
Hi everyone. My name is Michael Cyger and I’m the founder and publisher of iSixSigma.com – the largest community of Lean and Six Sigma professionals in the world and the resource for learning to drive breakthrough improvement.
Here’s what we do here: We bring on successful lean and six sigma business leaders; we learn from their experiences; and they share their strategies and tactics with you. Then, when you have a success to share, you can come on the show and give back as today’s guest is going to do.
Now, today’s show is a bit different. Instead of bringing on a statistical genius and watching me stammer and stutter trying to ask insightful questions, I decided to head over to Seattle and join part of a week-long training session provided by Minitab.
The instructor is Eduardo Santiago, and I thank Eduardo for welcoming me into his classroom for an hour of the course and allowing me to video tape it. I also want to thank David Costlow at Minitab’s headquarters for helping facilitate the session.
Whether you’re learning about statistical process control for the first time or, like me, you’re using this video as a refresher, I’m sure you’ll agree it’s packed full of information and a tremendous resource.
Here’s your program.
Eduardo Santiago: The next topic is Statistical Process Control. So what we’re going to be talking about here – we have a few concepts on the right hand side. We’re going to be talking about something called common cause variation versus special cause variation. We’re also going to talk about the concept of rational subgroups and why do we need to collect the data in rational subgroups. We’re going to try to motivate that. We’ll talk about something that is typically known as phase one and phase two for control charts. Then later on, the obvious concepts of when is a process in control and when is the process out of statistical control. Then after that, we’re going to discuss the eight different tests for identifying special causes of variation. And finally, later on in this chapter, we will talk about stages, okay? It’s a very important concept. Okay?
So, the father – a lot of people actually say that the father of modern statistical quality control – is Walter Shewhart. And he’s the one who actually created these tools to monitor the quality of a process. He was working at Western Electric Company; so they were the manufacturing arm of AT&T. And manufacturing was a little different back then – in the twenties and thirties. So sometimes – just to know what is a typical situation; what is a typical scenario in which we can actually apply – the control chart, we have to think about the manufacturing back in the twenties and thirties. Okay? It doesn’t mean that it is no longer applicable. But you have to think about what were the assumptions to actually apply these tools. Okay?
So, before that. Before Shewhart actually came up with Statistical Quality Control Charts, what were people doing? What were quality departments? Well, quality was limited to, basically, someone in final inspection doing a hundred percent inspection. So before control charts were established as a tool for monitoring a process, quality was forced. Okay? You basically did a hundred percent inspection and separated – hopefully – all the product that was actually non-conformant to customer specifications.
And one of the things Shewhart realized is that companies didn’t really understand variation. Customers did not actually understand what affects variation. And that’s where the concept of common cause versus special cause variation comes into play. Okay?
So, what is a control chart? Let’s go to page 3-3 on your books. So the agenda for this section is going to be very simple. We’re going to be talking about control charts for continuous data and control charts for attribute data. So, I think you’ve all seen – and just raise your hand. Who has already used, or seen, control charts? So the majority, I assume. Okay.
So, what is a control chart? Well, what we got here is something that we call the centerline. And then we have two other limits here that we typically refer to them as the upper control limit and the lower control limit. Not to be confused with specification limits, okay? These are different, okay? Typically, a specification is provided by the customer. A control limit is determined by the process itself. Okay? And a lot of times people struggling understand this: that control limits are not your specs. A specification limit is determined, always, by the customer. While a control limit is really determined by the process itself.
So, what are control limits really doing? Well, what Shewhart did. He said, ‘Well, I’m going to assume, here, the process behaves, maybe, normally distributed. And I’m going to create a graphical tool that allows me to monitor this stability of a process parameter’. So the simplest case; just to keep it very, very simple – let’s think about the mean. Let’s think about the mean of the process. So the idea of these control charts is to determine limits, which we call the lower control limit and the upper control limit, such that if the mean does not change – if a mean of a process is steady over time – most of the points recorded will be well contained within the control limits. You may have something like this – some randomness. Something like that, right? So what is the percentage? 99.73%. In other words, if the mean of the process does not change, you would expect most of the points – 99.73% of the points – to fall within the control limits. And that’s the way we calculate the control limits.
So, for instance, here – one simple mechanism. You’ve probably seen it before. It’s X-double bar is the average of all the subgroup averages. It’s the average of all these averages. And here, for the upper control limit, a simple formula would be X-double bar plus three times the standard deviation estimated from the data divided by, something that we call, the square root of the subgroup size. I’ll talk about that in just a second. And here it would be kind of the same idea. X-double bar minus three times the estimate of the standard deviation, which would be divided by the square root of the subgroup size.
Now, in essence, all it is, is the centerline plus or minus three times some standard deviation. Just to keep it in very simple terms, all it is – the control limits is the centerline. The centerline plus or minus three times the standard deviation.
Okay. So one of the things Shewhart noticed is that in practice in manufacturing a lot of times, even though we assume the data follows a normal distribution, it doesn’t quite happen like that. When you start collecting data from a real manufacturing process, the data does not behave normally distributed. Most of these control charts work under the assumption that there is some underlying normal distribution. So what he did – he basically came up with the concept of rational subgroups. Anyone familiar with that? What is a rational subgroup? A rational subgroup is, essentially, a point in time in which are going to go to the process, right? And the conditions will be fairly similar that allow you to actually obtain not just one sample, but more than one sample at the same time. Not at the same time. Say, you go to to the process from 8AM to 9AM and you get six samples completely at random. You’re assuming that the characteristics of those six observations will be fairly similar. And then you might go to the process again from 12PM to 1PM and get six more samples. So the idea of sampling more than one part at a time is what actually is called rational subgroups. And sometimes this is not feasible.
Now, why do you think he recommended the use of rational subgroups? Why do you think he actually said it’s a good idea that whenever you sample from the process, you get more than one part a time? You get, maybe, five. You may get ten, fifteen parts. Why do you think?
Student: More than 100?
Eduardo: That’s right. Exactly. So he knew that a lot of times data in manufacturing was not going to be normally distributed. Well, it turns out if the data is not normally distributed, but you collect the data in subgroups, the averages will tend to behave like a normal distribution. And that’s the result known as the Central Limit Theorem.
So let’s go to page 3-5. This is example one – color consistency. And the problem goes like this. A company uses plastic pellets to manufacture outer cases for computer monitors. They want to evaluate whether the color of the cases is consistent over time.
Data Collection. Quality inspectors randomly collect five cases every four hours over an eight-day period. The color of each case is assessed using the L-star Valley of the LAB Color Scale.
Let’s go ahead and open color one. So everyone, please go ahead and click on open project. The name of the data set is Color Number One. Just beware there’s a couple of them. The one we want is color number one. So let’s talk about how the data was collected over time. So here we’re measuring the color of these plastic pellets that will be used for the outer case of a computer. So it’s really important that there’s consistency, right? That the color is consistently the same. So that when you use two different units, they look alike. So the way we collect the data is by going to the process every four hours and collecting five samples. So here, are we using rational subgroups? The answer is yes, we are using rational subgroups. And what is the subgroup size? It’s five. That’s in terms of my notation here. That’s what I have denoted as M. So in this particular case, M equals five. And then I would go to the process again at noon. I would actually also collect five random samples and then I would proceed to do that at 4PM and 8PM – every four hours during a period of eight days. So this is a data. This is all we really need.
So, how do you know which control chart to use? Well, I would like to actually use the assistant menu here. Just to showing something here really quickly. If you go to assistant choose control charts. I want to show you the following flowchart just to make a decision on which control chart we need to use. So the first question you need to answer is: what type of data you’re collecting. Is it continuous or is it attribute? In this particular case, the L value is a continuous number; so we’re going to go to continuous. Was the data collected in subgroups? The answer is yes. Was the subgroup size eight or less? Yes, it was five. So therefore, we’re going to be using the X-bar R Chart. And if you’re wondering why eight or less; why more than eight – where are these rules coming from? Well, the AIAG – Automotive Industry Action Group – has an SPC – Statistical Process Control – manual that actually establishes all those rules. So we’re closely just following the same rules of thumb here.
So let’s go ahead and create an X-bar Chart. But not from here. Let’s go to the Classic Minitab Menu, not the Assistant. Later on, we’re going to come back and use the assistant. For now, let’s use the Classic Minitab Menus. So let’s go into Stat Control Charts. These are variables charts for subgroups. And let’s go ahead and select the X-bar and R. So it’s the Stat Menu. Control Charts. Variable Charts for Subgroups, not for individuals. And choose the X-bar R.
So here, all the observations for the chart are actually in one column. All the observations are indeed in column C1. So just click on that field underneath the drop-down menu. So just click on here, and you should be able to select readings. Then the second input that’s required here is subgroup sizes. So there’s two options. You can either put a number five; because we know the subgroup size is five. Or we can also tell Minitab to figure out what the subgroup size is by just looking at this – the second column. Using a column like this to track the subgroup size gives us the flexibility to have unequal subgroups sizes. Sometimes it may not be feasible to get five parts all the time. So you would need a second column to keep track of that. So in this particular case the subgroup size was exactly five. So it doesn’t matter if you put a number five here, or you choice the date/time. The result will be exactly the same.
Now, remember Minitabs dialogue box has the minimum requirements to run this command. If you click okay, that would generate a control chart. However, I want to customize something about the control chart. I want to make sure that the X-axis has a timestamp. I want to make sure it actually tells me what day of the week, what time of the day the information was collected. So go into Scale, click on the Scale button, choose the Stamp Radio button, click on this field underneath Stamp Columns, and select the date/time column C2. Let’s go ahead and click okay.
There’s two control charts. What is the X-bar R Chart? Well, we got a control chart for the ranges. For each subgroup, I’m going to have a range. And for each subgroup I’m also going to be tracking a subgroup mean. So this is the X-bar Chart. This is the Range Chart. And you can see that the information about the day/time was actually stamped on the X-axis just like we requested. Why do we have two control charts? Well, we use two control charts because they do different things. The graph at the bottom monitors the variation of the process – the ranges. The purpose of the R Chart is to monitor the variation of the process. That means Sigma. What about the one above? Well, this one, on the other hand, tracks or monitors the stability of the average of your process.
So, typically, we go to the Range Chart first. We look at that one. And we ask ourselves the question: Is the variation in statistical control? Well, all the points are actually contained within the control limits. So our conclusion is: the variation is steady over a long period of time. Is the variation changing? Well, I mean the estimates are changing. But there is no evidence to suggest that Sigma has changed over the eight day period in which we collected data.
So the variation seems to be steady – seems to be stable – over time. Then we move up and look at the means. And what do we see there? Well, we see one, two, three, four, five, six, seven. Seven points out of company subgroups? Well, we collected samples every four hours during eight days. It’s like forty-eight subgroups. So, is that just a fluke? To see seven points out of control from forty-eight? Can that just be a fluke? No. There is something systematically happening in the process that’s making your process go out of control. And then here’s where you need to do some investigation.
Well, what is causing the process to go out of control? Is it raw materials? Is my operator changing the parameters of the machine? What’s going on? We need to do some investigation and figure out what is causing these processes – these means – to go outside the control limits. If we find the actual root cause, we can prevent it from happening again. Take corrective actions. And that’s really what Shewhart referred to as common cause in special cause variation. And this something that we’ve been talking about this week. We tend to react to changes from just sample to sample. Like here, for instance, we see the range is 2.5. And then the second subgroup, the range was about 1.8. We tend to overreact to those small changes.
Variation is natural. Variation is something that we have to deal with no matter. Things will have a common cause variation that will make things fluctuate. But hopefully it’s only common cause. And every now and then, there will be a signable causes. Things that actually make your process go outside of control. And Shewhart referred to those as special cause variation. So, really bad quality of a new batch of raw material could be special cause of variation. Your operator actually changing the machine parameters is another special cause of variation. There’s a lot of different things that could actually occur. Maybe all of these can be assigned to one of the ships. I actually seen control charts where all the points that were out of control belonged to ship number three. And when we did some further investigation, we concluded that well, we didn’t have supervisors in the night shift. So something else was going on.
Okay, very good. Are there any questions up to this point?
Student Question: So you can’t brush the data sets?
Eduardo: This one you can’t. You remember Minitab allows you to brush points when the point represents an individual row. Here, what is this representing? This first point. It’s representing five rows. So you can’t really brush. That’s why. But if were actually doing an individual’s graph, like an IMR, we will be able to brush. But not for this one.
Let’s actually move on here. Let’s go to page 3-15.
So, we’re basically done here. We concluded that the variation of the process is stable and under statistical control. However, the mean is not. We need to do further investigation and fix those issues. Because this, as Robert said, it’s not just a fluke. It’s not a fluke. Something systematically is systematically occurring in the process that’s causing the mean to vary way more than expected. So this – the mean of the process – is not under statistical control.
So let’s go to page 3-15.
A process that is out of statistical control exhibits unusual variation, which may be due to special causes. Because several points are out of control on this chart, you should investigate what special causes may be affecting the mean color. Now, on the right hand side – under additional consideration -, I want you to focus on the third bullet. It says, ‘assuming normality’ – if the data really follow a normal distribution-; if the mean is not changing you would expect the process to say within the control limits with the probability of 99.73%. So that means that there’s a probability of having a false alarm of .27%.
What is a false alarm? It’s a point that is actually outside of the control limits when, in fact, the mean has not changed. Now, that’s going to be a rare event. It’s only going to happen about .27% of the time. So that’s why I was asking the question: Would seven out of forty-eight subgroups seem like a fluke? No. It seems like something is really going on. Now, if you actually had one thousand subgroups and you see about three points be outside of the control limits. What’s three out of one thousand? It’s about .27% roughly.
And then the fourth bullet here says, ‘the data do not need to follow up the normal distribution for you to use the X-bar Chart’. Even if the data are not normally distributed, the probability of a false alarm is low. So that’s really one of the advantages. So just remember Shewhart noticed that a lot of real data sets do not follow a normal distribution. So when you suspect that that’s the fact for your process, it’s important that you try to do your best to actually get as many samples as you can – as many subgroups.
Are there any questions?
Student Question: Can your subgroup size be too large?
Eduardo: No. It’s a waste of time. It’s a waste of resourced probably. Like using forty or fifty every single time you go do your process. It might be too many. But is that negatively affecting the performance of these control charts? It doesn’t. It’s just probably not necessary. Just think about how many samples should be good enough to really estimate Sigma. Twenty; thirty; forty – I mean, ideally. Do you need to go way beyond forty? You don’t. But can you get away with only five? And the answer is yes, you can also get away with five.
Student Question: How do we choose control limits?
Eduardo: The control limits are automatically computed based on the data. So, for instance, just to keep it simple, this one here. This is the average of all these subgroups means. X-double bars. The average of all the subgroups means. Forty. The control limits are populated in such a way that the probability of the data staying within those control limits is 99.73%. So, mathematically, those control limits will be X-double bar plus or minus three times some measurement for Sigma.
Student Question: Is there a reason somebody would say means can be higher probability than 99.73 (Unclear 25:40.1) control limit?
Eduardo: Typically, that’s not the case. I mean .27% – three out of every one thousand subgroups – being false alarms; that’s pretty low.
Student Response: Right.
Eduardo: That’s pretty low.
Student Question: Is there any special consideration for processes that are like low volume, long leap time or complex, where this seems like?
Eduardo: A stable process. That you’re doing, sort of, mass production. And then that’s right. And that’s why I was actually bringing up the comment earlier today about think about how manufacturing was in the twenties and the thirties. It was mass production. So if these guys at Western Electric Company were producing phones, there were probably only two types of phones. White phones and black phones. And that was it. Right? Ford truly believed that everyone just needed a black car. It didn’t think that color was important. So it was just mass-produced. So, you’re right. These techniques assume that the product will be produced on a long term basis. Like you’re not doing it in short batches – in small batches – and then changing to a different product, and then changing to something else. Right? You need really a lot of data. And this is just something that you want to monitor from this point on. And see if your process has remained the same. Is my variation the same? Is my mean the same?
Now, there are special topics on control charts. And I’ll only briefly mention this. I won’t get into details. But there is something called Short Run SPC. And if you’re really interested in that topic. Like say, you’re manufacturing screws. And you got the quarter inch, a one inch, a half inch, and so on and so forth. The process is almost the same, but what you’re measuring is different. It’s actually different because the specification is changing. The target is changing. The nominal value is changing. So if you produce screws of different dimensions in small batches, but it’s still the same family of products, you can group together information. And that’s what we call a Short Run SPC. So even though we’re not producing the same product for a long period of time, we can get information together using a control chart known as ZMR. If you are really interested in this topic, just send me an e-mail and we can follow up on this.
Okay. So before we move on to the second example, I want to talk about something that’s not in the book. It’s not explicitly described in the book. But Shewhart referred to something called phase one and phase two for control charts. So, he though that ever single process should be monitored in two different stages – in two different phases. Phase one is a learning phase.
So what do you do in that phase? Well, you’re collecting data from the process. And with the purpose of doing what? The purpose of understanding what is the true variation of your process and what is the mean of your process. So as you collect more, and more, and more data, you gather information about Sigma and MU. And there are different rules of thumb. You’re supposed to collect about twenty-five subgroups with at least a hundred observations total. So if maybe there’s twenty-five subgroups of size four, that’s actually enough. Or if you actually collect thirty subgroups of size five, that’s more than plenty. Now, if you collect such number of samples and there is nothing out of control, you can calculate what the true estimate. Well, you’re going to accurately estimate what the true value of Sigma and the true value of the mean may be. And that’s phase one. Phase one is a learning phase. The purpose is just basically collect data from the process when it’s in control. There should be nothing going out of control. And that should allow you to estimate MU and Sigma.
Now, notice what happens as I start collecting new information. Look at this. So allow me to go into the data set. You don’t have to do anything. Just watch me do this here. I’m going to remove this data set. I’m going to remove this chunk of the data set – the last three subgroups. So if I go back to the control chart, it says what? It says that the data has changed and it’s not longer reflecting the data set. I’m going to update this graph right now, and just look at this control limits. What happened to them? They changed, but just slightly, right? They change. Well, in phase one, as you collect one more subgroup, and another subgroup, and another subgroup, the control limits will continuously be getting updated. But it reaches a point where enough samples have been collected. You know what MU and Sigma should be. And in that point you move on to the second phase. In the second phase what you is you fix the control limits and you don’t let them change based on the data. That’s phase two.
So what is the purpose of phase one? How would you put it in your own words? What’s the purpose of the first phase?
Student Response: Understand the capabilities of the process.
Eduardo: Not the capabilities. Because that usually involves like the specs. I know what you mean. So, understanding the parameters of the process. Its mean and its standard deviation. Once you gather data to actually know, and “you never really know”; but once you collect enough data, you have a pretty good idea. You can well, this is what MU is. This is what Sigma is. Let’s use those to fix the control limits and continue to monitor the process for this point on.
So if the process changed – if the mean changes – what’s going to happen?
Student Response: The graph will shift up or down.
Eduardo: Perfect. Allow me to actually create a picture that graphically depicts what you just said.
So this is where we think the process should be. So the assumption is that the process is, right now, here. We’ve estimated Sigma and MU from the data in phase one, and this is what MU-zero is, and has some standard deviation. But suppose that something has happened in the process. Something has truly happened in the process that has made this distribution actually shift to the right. So the mean is no longer MU-zero. Now it’s MU-one. What do you think is going to happen? Exactly what John said. It’s going to be now not just a little likely. It’s going to be a lot more likely to see points above the control limit. And that’s something we need to investigate. What actually produced this shift? So that’s what we do in phase two.
Phase one we learn about the process parameters. We fix the control limits. And we move on to phase two, where we just continue to monitor the process. If something changes, we should be able to identify it.
Student Question: What if the shift is not dramatic? Like it happens gently over time and you need to know where that shift started.
Eduardo: Very good question. So the question – if I clearly understand. A large shift can easily be identified with these control charts. And that is very true. So what do you when the shift is very gradual? It’s slowly moving. So, control charts may be a little too slow to react and identify that. But if you go to page 3-15, we actually talk about that concept.
The first bullet says, control charts for subgroup data are sensitive to “large process changes”. If you want to detect small change in the mean, use other charge such as QSum or EWMA – Exponentially Weighted Moving Average -. So there are other control charts that are specifically designed for detecting smaller shifts. Now, if you don’t want to use QSum or EWMA because you’re not comfortable with the interpretation, you still want to stick to these typical classic control charts, there’s one way we can actually detect even smaller shifts. We could increase the sensitivity, or the power of the test, by adding other tests for special causes. The only test for special causes we have actually discussed at this point is if the point is outside of the control limits, there might be a special cause. There are seven additional tests that you can include in order to increase the sensitivity and make it more likely for you to detect those smaller shifts.
Student Question: Did you say you have to get your process in control before you can go on to phase two? Is that correct?
Eduardo: Yes. That is correct. The assumption is, like, you’re trying to estimate the mean and the standard deviation. If during phase one there are things happening to your process, are you really getting the trust estimate of MU and Sigma? You’re not. So that’s why you have to make sure that the process is stable during that process – during phase one. If it’s not stable, you got to continuously fix the process, fix the process, and eliminate as much as you can those special causes of variation.
Student Question: Is there, kind of, a general rule for how much change you see in your control limits, but you can say that my process is stable? You’re saying during phase one you’re looking for it to move. And as you take more measurements, it should stop moving to a point where you feel that’s it, now we understand.
Eduardo: That’s hard to say, because it’s always going to relative to the magnitude – the scale – of whatever you’re measuring. So that will be difficult for me to say, oh, if you see a change of ten percent, or five units; it really depends. Now, once you start gathering more data, you’re going to see that even if I continue to add more, and more, and more data, these control limits will not change. Where you see a lot of change is in the beginning. When you only have like five subgroups. You collect the sixth one. Yeah, they can dramatically change. Because you haven’t collected enough information about the process. But once you gather twenty or more subgroups, you should start to converge.
Student Response: Okay.
Eduardo: If at that point you see something completely different, something’s wrong.
Okay. So let’s go to page 3-16. This is example number two. It’s a continuation of the same process – color consistency. But specifying historical parameters. The X-bar chart revealed that the process is out of statistical control. Rather than rely on the more recent sample data to estimate the parameters for the control chart centerline and control limits, you sample data, record it when the process was in statistical control. Specify a process mean of forty and a standard deviation of .96. Then use the X-bar in our charts to assess the control status of the process.
So, let’s open the data set Color Number Two. Color -> Color Number Two.
Okay. So this is just additional information about the same process. Now, what it’s actually saying is: we know that the X-bar chart was out of control. So instead of actually trying to estimate what MU and Sigma should be based on that data set, why don’t we use historical data from a point in time in the past where we did not observe any anomalies? So the parameters based on that process data was a mean of forty and a standard deviation of .96.
So, let’s create an X-bar R assuming that we are in phase number two. So when you assume. When you fix the control limits, you’re assuming that you’re in that second stage – that second phase. So let’s assign the readings here as all observations for our chart are in one column. The subgroup sizes – you can use a number five, or the day/time column. Now, where do you go to specify the parameter of the process? You go under options. You click on this button, and X-bar and R options. And here, for the historical mean, we’re going to be using the value of forty. And for the standard deviation we’re going to be using the value of .96. Once you’re ready, go to tests.
Now, allow me to focus this for just a second. So remember, if you want to increase the sensitivity of your control chart to identify smaller shifts in the process, you got to include additional tests. That are a total of eights tests here. And the only one – the default – is just outside of the control limits. That’s the easiest one. Now, there are others. Usually, the most commonly used are test number one, two, and seven. So let’s see what some of these rules actually do.
So let’s focus on test number two. Let’s see if we can figure out what test number two does. It says, ‘K points in a row on the same side of the centerline, where K is 9’. So that means that test number two raises a flag when nine points in a row are on the same side of the centerline. You think that that’s very likely? If the process has not changed, do you think it’s very common to see one, two, three, four, five, six, seven, eight, nine on the same side? No. It probably means that what has happened? That a shift has occurred towards that side. So that’s a very common test used to detect even smaller shifts.
Now here, for instance, this is another one. This for a trend. So, ‘K points’. So there’s degradation or some increasing trend. If there’s six points in a row all increasing, or all decreasing, that’s usually another indication that there’s something going on in the process. There has been a systematic change. And there are others that basically say, well, two out of three points outside or beyond two standard deviations from the centerline. Four out of five points outside one standard deviation from the centerline. Or here, this one. Fifteen points in a row within one standard deviation of centerline. What does that mean? Well, all of a sudden you’re supposed to see variation all across the board. If for some reason you just see one, two, three, four, five, six, seven, eight, nine, ten, eleven, twelve, thirteen, fourteen, fifteen very close to the centerline, something’s going on. Usually it’s the operator. When that happens, it’s very common that maybe the data is being modified by the operators. It could also be that something actually affected the variation and made the variation smaller, which would be great news. Because why would actually the process go from exhibiting larger variation in the means to just basically have smaller variation? So it’s actually a positive change in the process.
Okay. Now, you can choose to perform all tests for special causes. You can choose it from the drop-down menu. You can tell Minitab I want to perform all eight tests for special causes. But that has a disadvantage. What happens when you actually select all these tests is that you’re also negatively affecting the false alarm rate. So remember that for test number one, the false alarm rate was .27%. Now, if you use eight tests, you’re increasing the sensitivity, but you’re also increasing the number of false alarms. And you’re supposed to do investigation. You’re supposed to maybe even stop the process and try to figure out what’s going on. So if you want to use all eight tests, you have to be willing to accept also a higher false alarm rate.
Now, what is a false alarm if you go from one test to eight? There’s a famous paper by this professor in Virgina Tech. His name is William Wardell, or Bill Wardell. He actually has a paper that explains what the false alarm rates go up to when you use multiple tests simultaneously. We – Minitab – has done a lot of research for the assistant menu, and we actually figure out that to get a good protection – good detecting capability – without dramatically increasing the false alarm rate, for phase one you use being using test one, two, and seven. And for phase two it’s just test one and two. And those are the rules that are implicitly assumed by the assistant menu when you use our control charts.
So let’s go ahead and click okay here. I’m going to assume I want to run all eight tests. So let’s go ahead and click okay.
Student Question: You said phase one was one, two and seven? And phase two was?
Eduardo: One and two. Just one and two. You drop out seven.
Student Question: Did you ever change the numbers (Unclear 45:17.5)?
Eduardo: You could. If you understand what the rule’s really trying to identify and you know that something in your process might be very likely to occur. Well, you want to actually increase the sensitivity to that particular case by maybe either reducing or increasing K. Depending on what the test is.
Student Question: Do you ever change the numbers within the tests in the Minitab software?
Eduardo: Yes. But you can change it. You can definitely change it.
So, as we said, we typically look at Sigma. Is the standard deviation still .96? No. Probably not. Why? Because there’s four points out of control. That means a standard deviation of the process has changed. Is the mean the same? No. So, those historical parameters that we used have changed – are not the same anymore. And actually look at this; just to illustrate what I was saying earlier, I’m going to go here – all the way to the bottom – and just assume. Well, ten; twenty. Here you don’t have to do anything. And this is just for illustration purposes. I’m going to create, here, just a little more data. But I’m going to, basically, make it a very extreme subgroup here. So you would that the control chart would be able to detect that without affecting the control limit.
So why will the control limits not be affected? Because I’m using historical parameters. Yes, and it did actually pick it up. [Just undo here].
Okay. Let’s work on just a little exercise. This is on page 3-20. This is Exercise F – Stability of Photographic Film Density Measurements.
So go ahead and work on it individually. I’ll give you a couple of minutes. It’s just basically going over the process of fixing parameters. This is Film Density. We got data for three different control charts.
Do we have rational subgroups here? Yes. I don’t know if you noticed, but you can actually enter more than one variable at a time. And this will just actually produce all three control charts at the same time. So you don’t have to go back and change the variable name. Then under X-bar R options, the means, right? We do have a target for each one of these. So they should be 1.53 and 4.5.
Now, you might be asking yourself the question: How will Minitab know the correspondence? Well, it does it by association. Just like everything else. All the other dialog boxes where we have specified some values. It’s always by association. So the first mean will be actually for the first column that you enter. Second one for the second column. So on and so forth.
Let’s go ahead and click okay. And we can only fix the mean; and not fix the standard deviation. We can do that. So Film Density at one hundred and fifty in statistical control? Yes. Is the standard deviation stable over time? Yes. What about the mean? Same. It is stable over time. What about three hundred? Same story. Both parameters – mean and standard deviation – are stable over time. However, that’s not the case for four hundred and fifty. And the purpose of a control chart is to prevent from this situation from happening. If only we could’ve actually identified the problem right here. We could’ve, in theory, prevented the process from actually deteriorating furthermore.
Remember when we talked about the coding thing in this example with adjust versus no-adjust on Monday. So remember that second process with the no-adjustment. It was a very, very similar situation. And I said control charts are the tools that we use to monitor the process and prevent situations like this from happening. Would one point be out of control? Yes. But if we detect what’s going on – the special cause of variation -, we could’ve actually prevented what happened afterwards.
Okay. Are there any questions about control charts?
So let’s just quickly go over the concepts that we covered here. I’m going to go here to your right side. So, common cause versus special cause. So, variation is a natural thing. Variation will occur. And there are common causes for variation. Right? Different batches of raw material. Maybe even different suppliers, but the suppliers are similar in performance. Maybe the ambient conditions may differ from day to day. Some days might be a little more humid than others. So on and so forth. But that’s what we call common cause variation. Now, every now and then, there will be special causes that will produce changes that are not what you would expect. And that’s what we call special cause of variation. So the purpose of a control chart is to assess whether or not the process is stable in statistical control. And when it’s not in statistical control, the purpose is identifying where special causes may exist.
Now, the idea of national subgroups. Why are rational subgroups recommended? Because they protect you against data that is not normally distributed. If the data is not normally distributed and you can’t do subgroups, you might be in trouble. The control charts work assuming the data behaves like a normal distribution. So if that’s not the case, your control chart for non-normal data may not be accurate.
So, phase one and phase two is basically just the two stages. First we actually collect data from the process. Once you collect enough data from the process under stability, you can estimate the parameters – MU and Sigma -, fix the control limits, and continue to monitor the process under phase two.
We talked. Heidi was asking the question. How do you increase the sensitivity of a control chart for small shifts? You use more tests. You use some of the additional tests.
Stages. That’s the new topic.
Let’s go to the following example.
This is on page 3-22. Shrinkage in an Injection Molding Process. Parts that are manufacturing an injection molding process are shrinking excessively. The average shrinkage of five percent is unacceptable, and the process has too much variability. A quality improvement team uses a design experiment to investigate factors that may affect shrinkage in the injection molding process. Based on the results of the experiment, they reduce the mold temperature. After lowering the temperature, the team decides to further reduce shrinkage by modifying the injection molding tool, which is something expensive.
Data collection. The team collects shrinkage data in subgroups of size ten every eight hours. They labeled the initial data benchmark. The data after the first process changed – reduced temperature, and the data after the second process changed – molding tool modification.
Please go ahead and open Improve.mpg.
So, initially, we noticed that there was too much variation in the process. So we decided to run an experiment. And from that experiment, we concluded that reducing the temperature might actually reduce the variation in the shrinkage of parts. Then after that, there was another change to the process, where we used a new molding tool. So, very good.
Now, here, for injection molding processes, you essentially have multiple cavity; so it’s easy to get multiple parts. It’s fairly easy. So, here, don’t be surprised. Why ten? Because it was just there was a lot of data available. So it was something feasible. Okay.
So, this, using the criteria that we saw before for control charts; if you use more than ten observations per subgroup, you should be using the X-bar S. That’s the recommendation. So, let’s go into Stat –> Control Charts –> Variables Charts for Subgroups, and choose the X-bar S. Let’s just choose shrinkage here, and put date as a stamp for the subgroup size. You can also put the number ten. Go ahead and click okay, and answer the question. Is the process stable?
Student Response: (Unclear 58:33.5).
Eduardo: Are you sure?
Student Question: Which process?
Eduardo: Which process. Yeah, that’s the right question to answer to my question. What process? Why did you say that?
Student Response: Because we dealt with three major changes to the process.
Eduardo: Control charts are supposed to monitor stability. By definition, what is stability?
Student Response: Lack of change.
Eduardo: Lack of change. Everything remaining the same. If you consciously change the process, the process is no longer the same. So it’s really not fair to compare the process as if there were no changes. This is a concept that Minitab calls Stages. So you can force Minitab to calculate independent control limits for each unique stage, and then assess if there was stability within each part – within each process.
So let’s see how that is done. Let’s do Control+E. So there’s a couple of ways you can do that. This is probably the easiest way. Just keeping track of the stage on a third column in the worksheet – which we already did that. So, go into Options. If you click on stages, define stages with this variable, choose change, and leave the defaults where we define a new stage with a new value in the column change. So there should only be three stages. Go ahead and click okay, and okay. What do you say? Is the process stable and in statistical control? Yes it is.
So, this is where I was expecting a wow. You guys didn’t remember to say it.
So it’s a very simple tool, right? That even without someone understanding statistics, it’s clear what happened. We were, right here, at 5.25% on average. The goal was to be below five percent. So in order to reduce the variation, which was very large in the beginning, we reduce the temperature. What did that do to the variation?
Student Response: Reduced it.
Eduardo: Reduced it. What happened to shrinkage levels. They were reduced as well under five percent. Then further down the road, we actually modified the molding tool. What did that actually do to variation? Well, not much. I wouldn’t say it actually reduced it. Maybe not significantly. But on the other hand, it actually had a positive effect on shrinkage, which it kept on reducing it. This is a very graphical tool, and probably one of the best tools in Six Sigma to show a before and after effect. If you want to show management what your project has done to a process to improve it, you got to show how the process was before the change and then after the change.
Student Question: Upper and lower control limits are different. Aren’t overlapping for each one of these things. Did you say the mean is changed (Unclear 1:01:57.4), or you have to go back and run an ANOVA?
Eduardo: That would be an ANOVA formally, yeah. And it’s probably going to be the case, I think, if the control limits are non-overlapping. It also depends on the sample size. It’s tricky. I think, here, we’ve gathered enough data where a One-way ANOVA would basically say the means are different. But you’re right. That’s the right analysis.
Any other questions? So, with this, I think we covered the basics for Control Charting. So this is going to be the end of this little section. We’re going to have a ten minute break. Are there any other questions?
Eduardo: Well, thank you so much, and thanks Mike.
Watch the full video at:
https://www.isixsigma.com/tools-templates/statistical-analysis/understanding-spc/