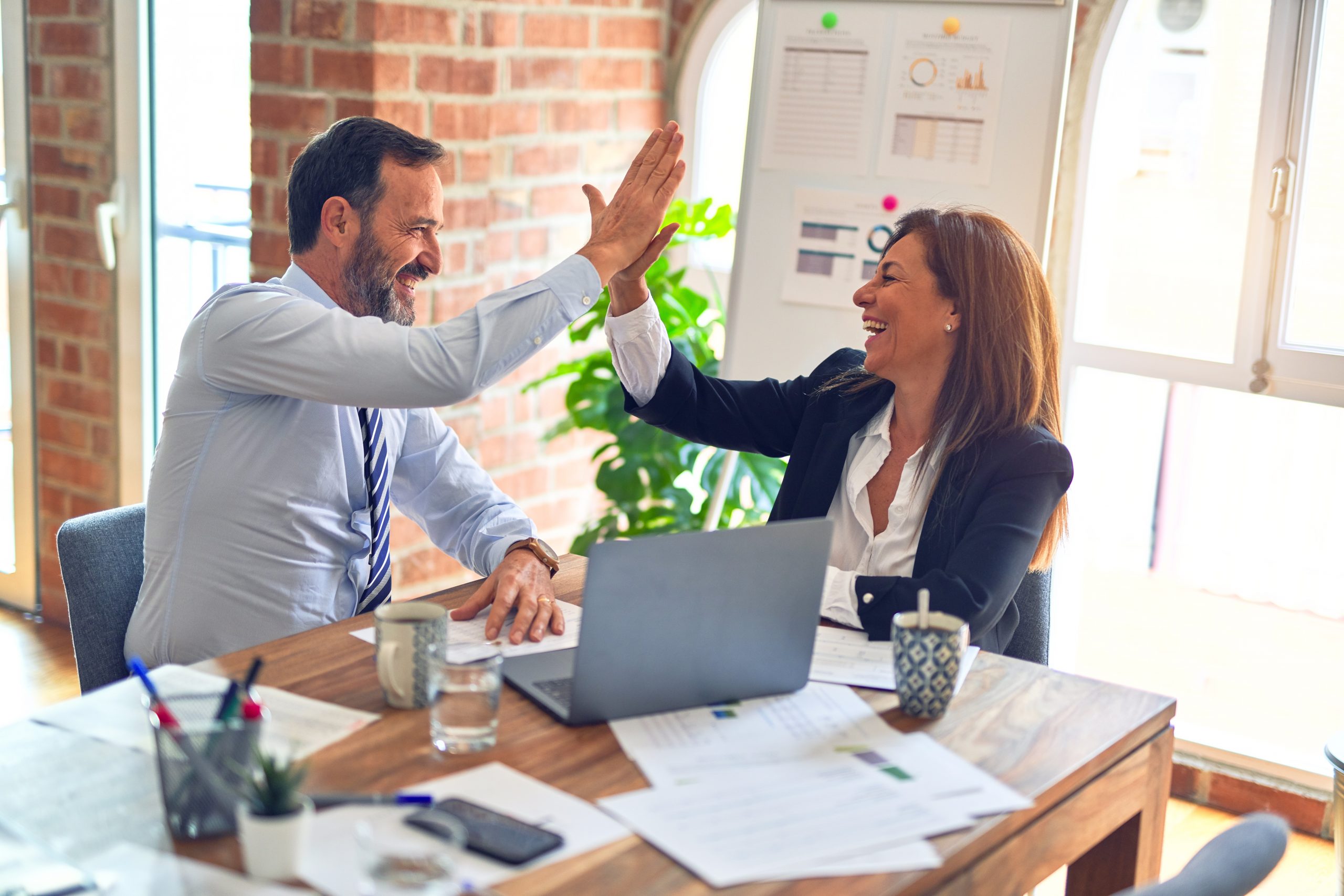
One of the most memorable quotes from the movie The Graduate (1967), staring Dustin Hoffman, is the brief exchange between young Benjamin and a family friend, who offers the following advice:
Mr. McGuire: I want to say one word to you. Just one word.
Benjamin: Yes, sir.
Mr. McGuire: Are you listening?
Benjamin: Yes, I am.
Mr. McGuire: Plastics.
Benjamin: Just how do you mean that, sir?
Fast-forward to 2010 and that one word may just be statistics. As Hal Varian, chief economist at Google, once said, “I keep saying that the sexy job in the next 10 years will be statisticians. And I’m not kidding.”
The rising stature of statisticians, who can earn $125,000 at top companies, is a by-product of the recent explosion of digital data. Six Sigma professionals should take particular notice because they work in the world of data analysis. They are trained in the use and application of statistics – including basic descriptive statistics and more advanced inferential statistics, such as hypothesis testing, ANOVA (analysis of variance), regression analysis and design of experiments (DOE) – to make better business decisions. Part 1 of this article will help practitioners understand the key drivers for the growth of statistics and introduce some leading analytics competitors. Part 2 will focus on choosing the right kind of statistical analytics, and offer some statistical tools practitioners can use for predictive decision making.
The Drivers
The confluence of technology, advanced computing and the Internet is creating an explosion of digital data. Understanding the digital universe is a little like contemplating Avogadro’s number – it is huge. According to International Data Corp. (IDC), the volume of data created by individuals and businesses continues to multiply, doubling every 18 months (“As the Economy Contracts, the Digital Universe Expands,” May 2009). At its current rate, the universe of content created across the globe will grow fivefold from 486 exabytes (note: 1 exabite is the equivalent of 1 billion gigabytes) to more than 2,500 exabytes by the end of 2012.
While this is great news for information seekers and data storage companies, it is even more important for Six Sigma practitioners. This explosion is opening new realms of data that will improve people’s quality of life and create meaningful, high-wage jobs. Consider how the world of digital data has made possible the ability to:
- Predict how and when components (products, infrastructure) will fail, before they’re even built. (“America’s Most Promising Companies,” Forbes, October 5, 2009)
- Anticipate and manage the flow of traffic to prevent the buildup of congestion. (IBM Business Analytics and Optimization)
- Detect influenza outbreaks around the world earlier. (Google Flu Trends)
- Get breakthrough pharmaceuticals to market faster by having drug makers share information about compounds they have tried and shelved for reasons like toxicity or inefficacy. (Massachusetts Institute of Technology’s Center for Biomedical Innovation’s New Drug Development Paradigms, launched in May 2009)
- Prevent strokes and illnesses via remote home-based health monitoring. (GE and Intel Home Healthcare Alliance, 2009)
- Detect heatstroke in sports players through sensors in equipment. (Hothead Technologies, Heat Observation Technology system)
- Seed the planet with trillions of sensing stations (each the size of a shirt pin and carrying 10 to 20 sensors) for measuring the earth’s vital signs – such as light, temperature and vibration – plus radio gear to send the information to a central location for earlier prediction of earthquakes, tsunamis and warming trends. (“HP’s Million Sensitive Spots,” Forbes, May 25, 2009)
Yet data is merely the raw material of knowledge. According to Erik Byrnjolfsson, economist and director of the Massachusetts Institute of Technology’s Center for Digital Business, “We’re rapidly entering a world where everything can be monitored and measured. But the big problem is going to be the ability of humans to use, analyze and make sense of data.”
The new breed of statisticians is unlike statisticians of the past. These data sleuths use sophisticated mathematical models and powerful computers to hunt for meaningful patterns and insights within vast troves of data. The good news is that Six Sigma experts are trained to analyze data and are familiar with statistical tools to help make sense of all this information.
Leading Analytics Competitors
Most companies generate simple descriptive statistics about aspects of the business, such as average revenue per employee or average cycle time per order. Leading analytics competitors, however, make analytics a core component of their business strategy (Davenport, Thomas H., “Competing on Analytics,” Harvard Business Review, January 2006).
These organizations regularly perform data mining and predictive modeling to concentrate on the most profitable customers, promote the right products and services to their customers, and even identify those customers most likely to cancel their accounts. They employ squadrons of analytical people with diverse backgrounds, including statistics, economics, computer science, mathematics and Six Sigma. Equally important, these organizations are generally managing analytical activity at the enterprise (not departmental) level across the value chain. As a result, they make the best decisions consistently over the long haul.
One of the foremost analytics competitors is Google. Google’s success as an online advertiser is based on its deep understanding of the data it manages. By creating sophisticated analytical models, known as algorithms, Google ensures that ads are as useful to the people who see them as they are to the advertisers who run them. Amazon.com’s ability to dominate online retailing can be largely attributed to their ability to mine existing customer data, which helps Amazon figure out the purchasing habits of certain customers, based on their previous purchases. Priceline.com’s successful niche of locating the lowest prices in the travel and hospitality industry stems from the sophisticated algorithm it uses to trawl the Internet and constantly update its database.
In healthcare, Medco Health Solutions Inc. is becoming a super analytic competitor. It is branching out beyond its core business – mail-order pharmacy – and using its massive database of patient information to mine genetic information to determine how well people metabolize drugs. In the process, it can identify critical medical side effects and pass these findings to physicians, hospitals, and drug manufacturers to improve outcomes and save healthcare providers money. Recently, Medco’s records showed, for example, that 22 percent of patients were hospitalized after receiving the wrong dosage of warfarin, a blood thinner. Using genetic information to determine how much of the drug to give different patients could save healthcare providers about $1.1 billion a year.
Wal-Mart’s supply-chain logistics models enable the company to have the right products in the right amounts at the right time for its more than 5,000 retail stores worldwide. In fact, Wal-Mart goes a step further by insisting that its suppliers use its system to monitor product movement at the individual store level, to plan promotions and layouts within stores, and to reduce stock-outs.
IBM, seeing an opportunity in data-hunting services, created a business analytics and optimization services group in 2009. The unit will tap the expertise of the more than 200 Six Sigma Black Belts, mathematicians, statisticians and other data analysts in its research labs. IBM plans to retrain or hire 4,000 more analysts across the company.
Capital One’s leadership position in the hyper-competitive credit card business originates from its consistent use of DOE to measure the overall impact of intervention strategies and then apply the results to improve subsequent analyses. Capital One conducts more than 30,000 experiments a year, with different interest rates, incentives, direct-mail packaging and other variables. This allows it to maximize the likelihood that potential customers will sign up for its credit cards.
United Parcel Service (UPS) is leveraging its strengths in operations research to track the movement of packages and to anticipate and influence the actions of people – assessing the likelihood of customer attrition. UPS’s Customer Intelligence Group is able to predict customer defections accurately by examining usage patterns and complaints. When the data points to a potential defector, a salesperson contacts that customer to review and resolve the problem, which can reduce the loss of accounts dramatically.
Profit Roadmap
Before diving into the tools, it helps to have a strategic business framework and an understanding of some key terms. Just as Six Sigma professionals use the DMAIC (Define, Measure, Analyze, Improve, Control) or DMADV (Define, Measure, Analyze, Design, Verify) roadmaps, businesses also need a roadmap for channeling analytics.
Start with the most basic challenge all companies face: How can we improve profitability? One reason companies focus on profitability is that profit is the “language of management.” A second reason is that profit can be impacted externally (increasing revenues) and internally (reducing costs). Thus, it is important to capture both sides of the business equation. In financial terms, this relationship can be expressed as:
Profit = (P – C) x V
Where:
P = selling price
C = cost
V = sales volume
In statistical terms, these three variables are considered response variables because they “respond” to various inputs. Response variables are also known as dependent variables or outputs, or can be denoted as Y. Figure 1 displays such a high-level profit roadmap.
Figure 1: Profit Roadmap
Source: Ohmae Kenichi’s The Mind of the Strategist (McGraw-Hill, Inc. 1982)
Starting with this high-level profit roadmap allows companies to focus their analytical energies and save time. When examining the “increase sales volume (V)” route, practitioners should start to ask some basic questions, like “Can market share be increased for Product X?” or “Can the market segment for Product X expand?” These key questions result in a series of actions, each with its own set of possible predictor variables. Predictor variables, or independent or input variables, can be used to predict the outputs. Figure 2 depicts the expanded decision tree for increasing V with actions and suggested predictor variables.
Figure 2: Expanded Decision Tree for Increasing V
Figure 3 depicts a similar decision tree for lowering costs (C). Practitioners start by asking questions, such as “Are the design specifications too excessive?” or “Are the fixed costs too high?” or “Are the variable costs too high?” Ultimately, these questions lead to actions and a set of possible predictor variables.
Figure 3: Expanded Decision Tree for Lowering C
Once practitioners understand the key terms and concepts behind the high-level profit roadmap, they are ready to begin using analytics tools, which are described in Part 2 of this article.