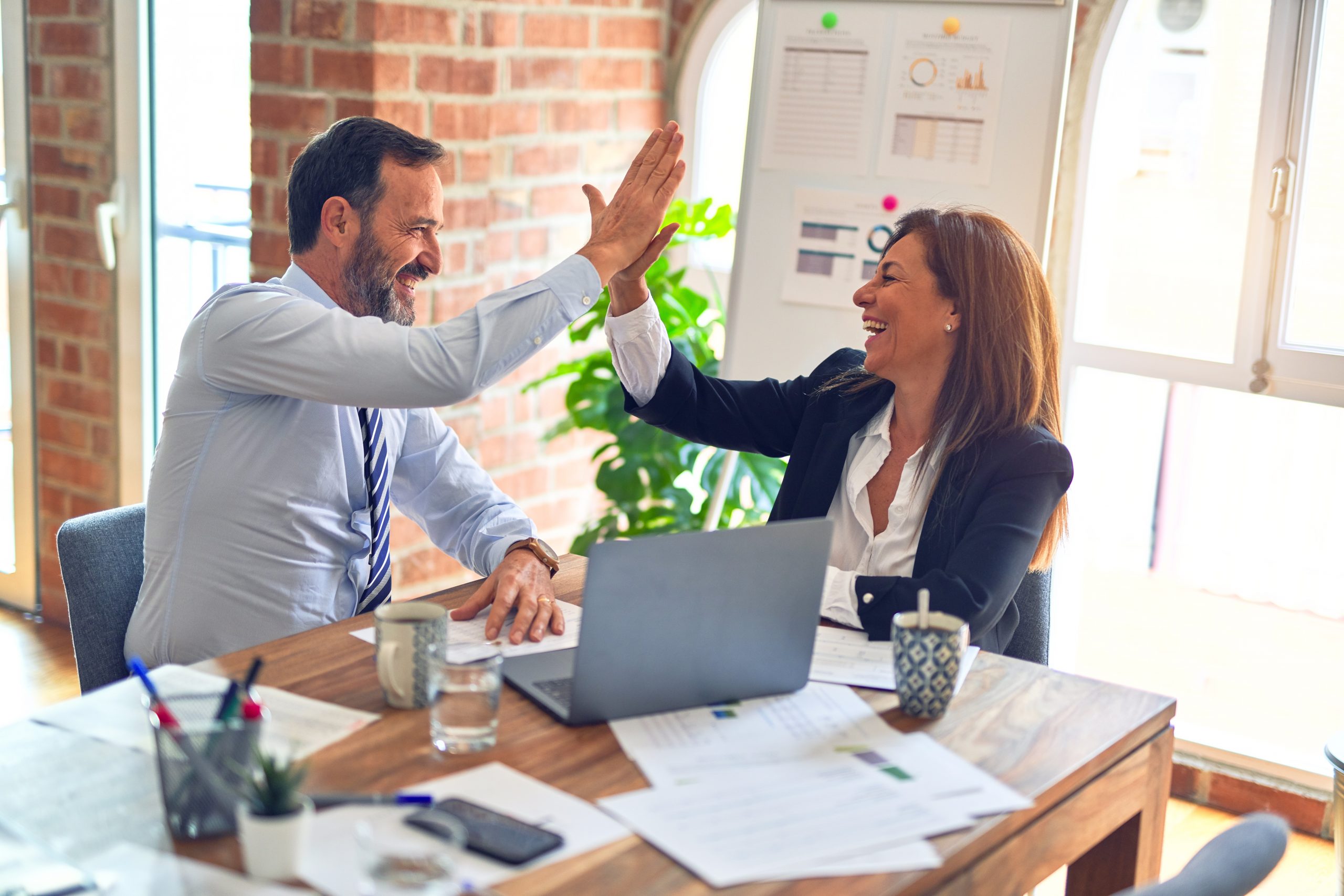
Nearly all medium and large companies spend hundreds of thousands, if not millions, on customer surveys every year. Customer survey results are used to amend strategies, design new products and services, and focus improvement activities. Gathering customer data is only the first step. The second step involves making best use of the data – analyzing it, drawing business relevant conclusions and making important decisions. In this step, practitioners can benefit from Kano analysis, a model for classifying customer responses. The following case study illustrates its use.
Case Study: Appliance Customer Data
A home appliances manufacturing company called on its customer care staff to analyze new satisfaction data and to suggest actions to the management team. All data was gathered using a four-point Likert scale based on whether customers were satisfied enough to buy the product again and a five-point Likert scale for how likely they would be to recommend the product to others (Figure 1).
Some conclusions were drawn immediately:
- Overall satisfaction seemed to have dropped from 2006 to 2007.
- Tendency to buy again and to recommend had gone down as well.
- Product quality seemed to be stable at a high level, whereas sales quality levels were also stable but left more room for improvement. Delivery quality had dropped.
- The major driver for satisfaction seemed to be product quality, with delivery quality following.

Calculating confidence intervals for all survey results proved the significance of all changes. The team decided to conduct more detailed analyses to find the culprit for the drop in delivery quality. Looking into the four major drivers for delivery quality revealed that cleanliness and punctuality left room for action (Figure 2).
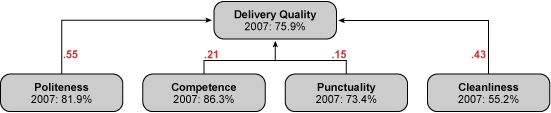
But would improving cleanliness and punctuality really drive the ultimate goal of repetitive business and recommendations from existing customers? The data seemed to suggest it, but practitioners wanted to be sure about the impact.
Time for Kano
Kano analysis is a tool – often mentioned in Six Sigma training, but not so often applied in projects – that can greatly help to structure customer needs based on feedback given. It divides customer needs into four categories:
- Must – This need must be fulfilled. However, fulfilling this need does not result in cus-tomer satisfaction, it simply avoids dissatisfaction. Example: Your new car comes with air conditioning – a must if you live in a hot climate.
- More the Better – Not meeting this need results in dissatisfaction. Delivering on this requirement generates satisfaction – the more the better. Example: Fuel efficiency of a car is of great interest for most customers.
- Delighter – Characteristic that is not expected by customers, hence it does not result in dissatisfaction if not present. However, this characteristic can be used to differentiate a product or service in the market to form a unique selling point. Example: Receiving a new car with a 10-year free warranty would be far beyond customer expectations.
- Indifferent – Customer does not perceive this characteristic as necessary, nor does it cause satisfaction if present. Example: Car manual printed in an additional foreign language the customer does not speak.
Information about these categories of customer perception for a product and service are of enormous value for improving performance and gaining market share. Practitioners can use customer satisfaction data to establish a Kano analysis with help from the Jaccard index of similarity. Paul Jaccard developed an algorithm that enables regression-like comparison of non-continuous data such as that collected through a Likert Scale. Additionally, this algorithm can filter Musts, More The Better and Delighter characteristics out of the data.
In this case study, use of Kano analysis and the Jaccard index enabled the following conclusions:
- Cleanliness turned out to be a Must, together with punctuality. These basic characteristics were expected. Fulfilling cleanliness and punctuality indicators would not lead to higher customer retention or recommendations. Working on these characteristics alone would not be not enough.
- Product quality and competence of staff during delivery and installation were seen as satisfiers: the more they were offered, the happier the customer would be. Working on these characteristics would be essential to driving sales.
- None of the indicators was able to “delight” the company’s customers (Figure 3). There was no unique selling point. As soon as its competitors came out with an iPod-compatible dishwasher, or some other unexpected feature, the company would lose market share.
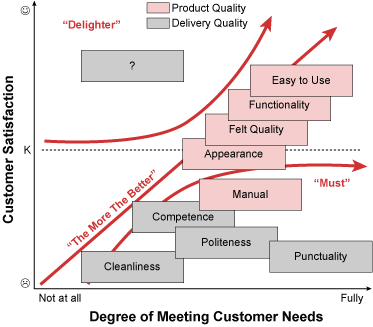
Put Belts to Work
Customer satisfaction data is not easy to come by. Therefore, it should be used to the fullest. Some basic mistakes can be avoided by appointing Belts with experience in discrete Likert data to complete the analysis. Additional value can be added with tools beyond the standard Six Sigma toolbox, such as the Jaccard index. Instead of relying on customer survey data providers for this analysis, Black Belts should be trained on additional methods to gain flexibility and save costs. Remember: Attaining the data is expensive, analyzing it can be cheap.