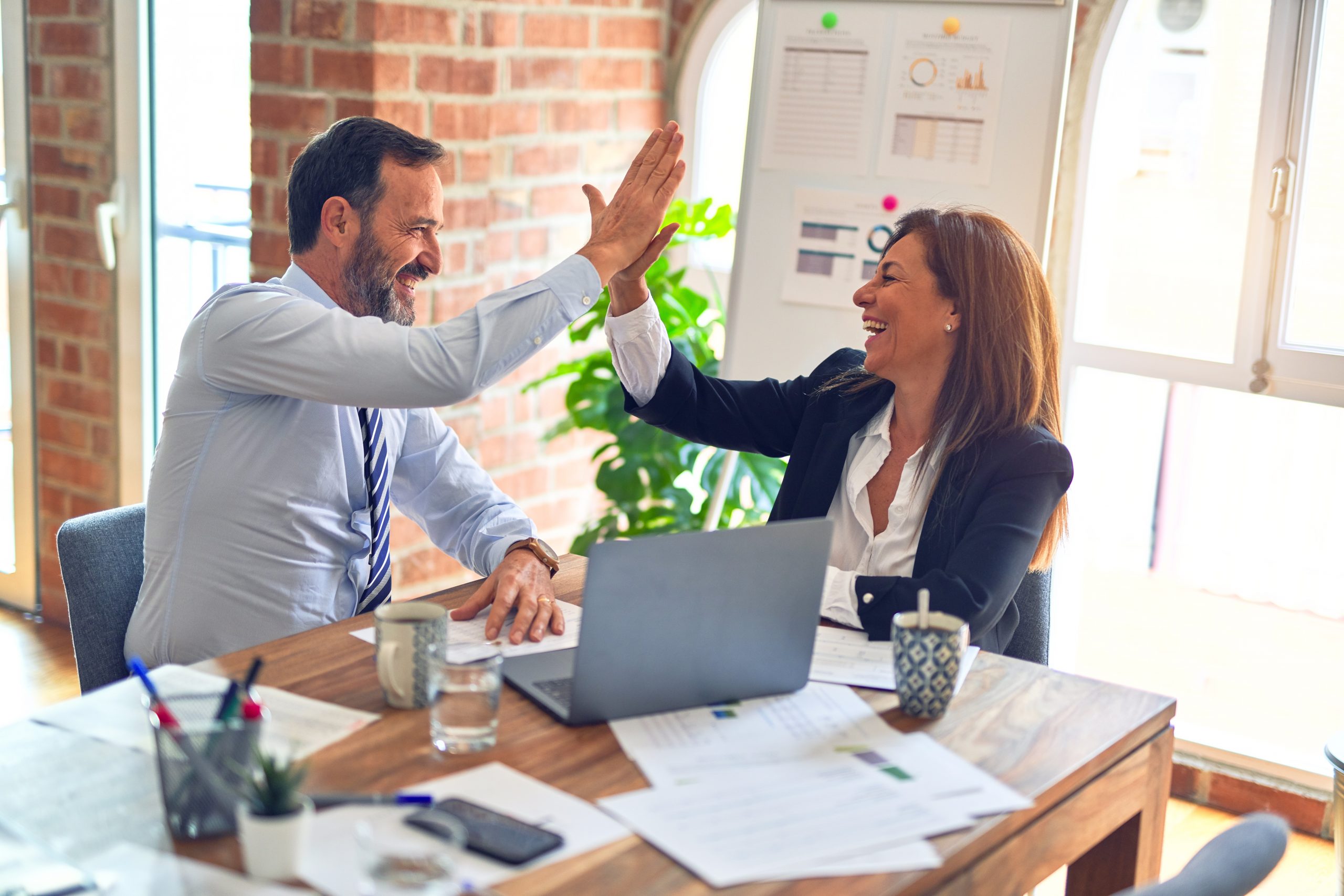
Let me end the suspense: not to p. At least for me. Also not to F. And not to t.
I got thinking about this topic after reading an article in the Wall Street Journal about “sloppy analysis” in scientific studies. That article is here, but you’ll have to pay to see it. However, the primary source for the article, available here, is an open access study by John Ioannidis. What originally caught my eye in the Wall Street Journal article was this zinger:
Statistically speaking, science suffers from an excess of significance. Overeager researchers often tinker too much with the statistical variables of their analysis to coax any meaningful insight from their data sets. “People are messing around with the data to find anything that seems significant, to show they have found something that is new and unusual,” Dr. Ioannidis said.
This situation sounded very familiar to me. In fact, substitute “Six Sigma” for “science” in the first sentence and I think the passage becomes even more true. We, as a Six Sigma community, rely far too much on formal tests of statistical significance to tell us what to do.
Statistical significance is nothing more and nothing less than a comparison of one thing to another. A comparison of a supposed “signal” to observed “noise” is the classic example. What gets forgotten is that when we experiment or otherwise collect data, we have complete and total control over what goes in both buckets. We decide what gets counted as signal, and what gets counted as noise. So the results depend entirely on how we sample. And there is no statistical test that can assess significance with this in mind, because it’s not a statistical question. It’s a practical one. Want better p-values? Sample differently. Want to make your F-test look good? Or bad? Change how you collect the data. Want that t-test to have a different result? Run the study again. Go ahead. Try. It’s really easy.
Don’t believe me? Check out Ioannidis’ study, which goes into much greater depth on this topic than I have the time (or admittedly, the intellect) to do. And note in particular the comments about lack of replicability of most studies, regardless of p-values. In other words, a study with a high p-value is no more likely to repeat than one with a low p-value. And the standard of p must be less than 0.05 has no practical relevance at all. To anything. Scary, right?
Now don’t get me wrong; I certainly don’t intend to condone manipulation of data to make things “look good” or support some pre-determined outcome when they really don’t. But on the other hand, I want to be very clear that blind faith in statistical test is just as bad. If you are letting software make business decisions for you on the basis of a p-value or F-test, you are behaving foolishly. After all, who made up the rules about what does and doesn’t constitute statistical significance? What were the circumstances, and what were they trying to do? Unless the circumstances were the same as yours and they were trying to do the same thing you are, you should make up your own mind. What your software package happens to think about statistical significance out to be immaterial.
So what good are these tests of statistical significance? Well, for enumerative work on historical datasets they can be useful. But in the world of Six Sigma where we are charged with predicting the future behavior of a process, let me be clear: they aren’t much good at all. You should be making your own decisions on what is and isn’t significant in your data. This will be based on tolerance for risk and how well you have sampled the process, among other things. You need to fully understand the level of knowledge you have based on your sampling strategy, assess your confidence in your conclusions accordingly, and make the best decision you can about how to proceed based on the particular situation you are in. Beyond some basic number-crunching, these are practical questions and concerns, not statistical ones.