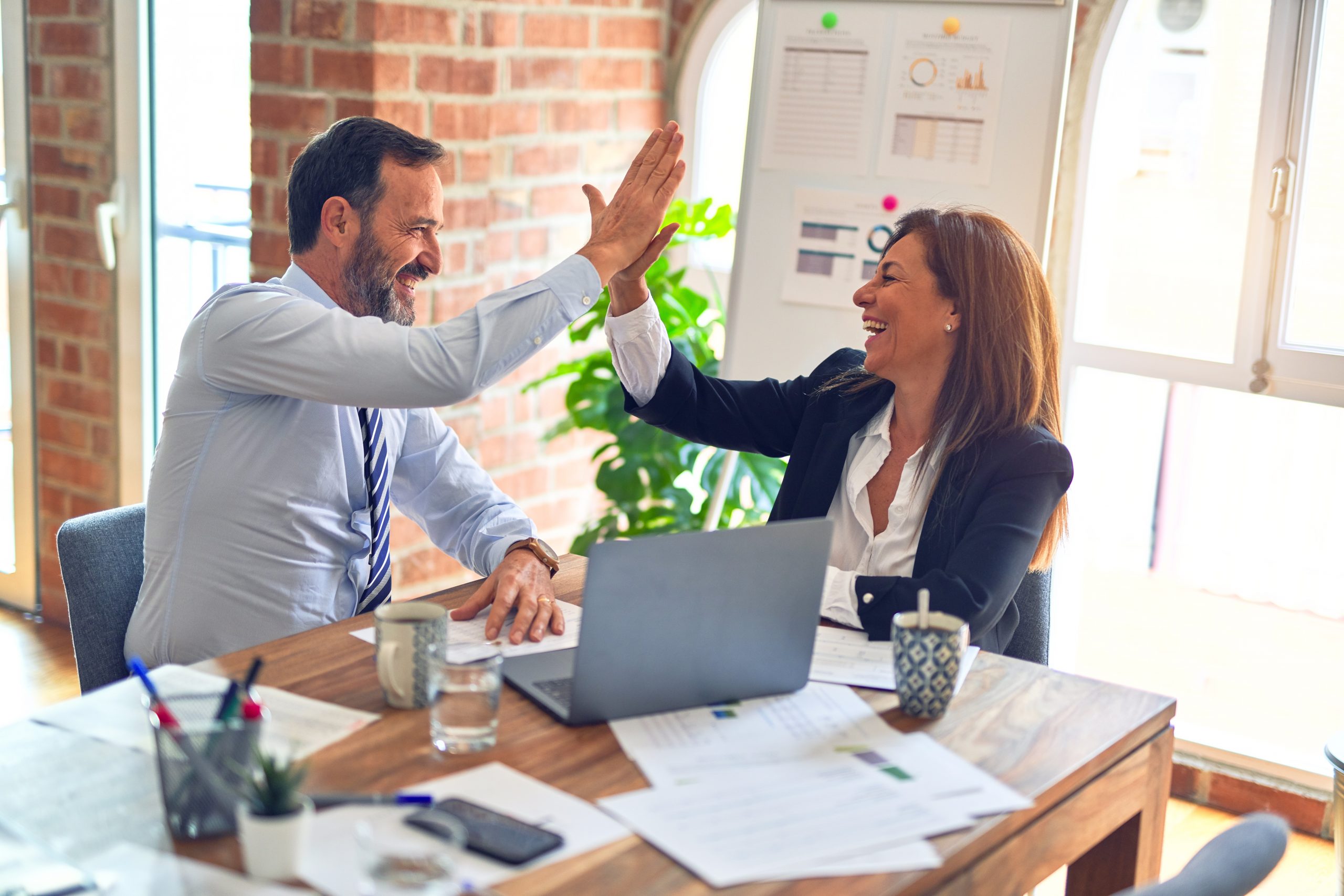
Measurement system analysis (MSA)Â can be fun, always impresses the customer, and can often result in an important surprise or two. Learn why gage R&R is only one part of the equation for achieving near risk free measurements of all types.
Characterizing measurement error is one of the most important yet overlooked and misunderstood aspects of any measurement process. Gage R&R is used in many forms to assess the measurement precision (spread). Determining measurement accuracy (central location) requires an understanding of stability, bias and linearity. Indeterminate measurements (statistical) may also require consideration of response-to-control (RtC) variable correlation and autocorrelation. When precision and accuracy measurements are assessed in combination, the analysis is referred to as measurement capability analysis (MCA) or MSA.
Process Accuracy Measurements
Stability
Stable processes are those that are free from special cause variation. Statistical process control (SPC), scatter plots, or other forms of statistical analysis are used to measure process stability. Stability determination requires enough data sampled to cover a wide range of possible variation contributors that apply to the process being measured. Possible contributors include:
- Part variation: piece-to-piece, raw material lot-to-lot, piece area-to-area, etc.
- Tooling variation: cavity-to-cavity, tool-to-tool, tool wear over time, etc.
- Human variation: operator-to-operator, supervisor-to-supervisor, set-up lead to lead, number of other tasks performed at the same time, ergonomic conditions, etc.
- Time variation: Sample time-to-time, hour-to-hour, shift-to-shift, day-to-day, week-to-week, month-to-month, season-to-season, year-to-year, lunch and other break times, etc.
- Location variation: machine to machine, building-to-building, plant-to-plant, state-to-state, country to country, etc.
Bias
Bias in a sample is the presence or influence of any factor that causes the data population or process being sampled to appear different from what it actually is. To measure process measurement bias, a higher measurement authority is compared to the data average. For determinate measurements this process is referred to as calibration. For indeterminate measurements, average values are compared to a target or specification value.
Linearity
Linearity refers to measurements being statistically different from one end to the other of the measurement space. A measurement process may be very capable of measuring small parts but much less accurate measuring large parts or one end of a long part can be measured more accurately than the other end. Linearity is measured using measurement standards calibrated to higher authorities, traceable to NIST. Indeterminate measurement linearity is measured in the form of interaction effects.
If equipment demonstrates non-linearity, one or more of these conditions may exist:
- Equipment not calibrated at the upper and lower end of the operating range
- Error in the minimum or maximum master
- Worn equipment
- Possible poor internal equipment design characters
Response-to-Control (RtC) Variable Correlation and Autocorrelation
Measurements are often used to control processes that in turn affect subsequent measurements. When indeterminate measurements are taken at a slower rate than the time it takes for process changes to be fully implemented then measurement adequacy is not an issue. However, low control variable (used to control the process) to response variable (being measured) correlation can mask the time it appears to take for a process change to be implemented. Using SPC to control a process where data is taken and process changes are made every hour yet where poor RtC correlation masks changes that take a full day to implement would not be adequate. RtC variable correlation is measured using regression analysis. This situation exists when the response variable is not the same as the control variable, which is often the case.
Similarly, autocorrelation affects measurements when correlation between paired values of mathematical functions taken at constant intervals incorrectly indicate the degree of periodicity of the function. Woodall states that much lower levels of autocorrelation than Wheeler’s suggested 0.7 minimum recommended level can have a substantial effect on a control chart’s statistical performance.
Process Precision Measurements
Gage R&R
Gage R&R statistically isolates different types of variation in the measurement process. These types of variation include:
- Repeatability = equipment variation = within variation
- Reproducibility = appraiser variation = between variation
- Residual or pure error
- Variation due to interaction effects. For example, out of several inspectors, one might have a tendency to read one gage differently than others
Gage R&R can be applied to any kind of measurements (attribute or variables, indeterminate or determinate).
There are many overlapping methods outlined in the literature that can be used to perform Gage R&R. A few of these methods are as follows:
- Analysis of variance (ANOVA) method
- Average and range method
- Within part variation (WIV) method
- Automotive industry action group (AIAG, Southfield, MI) method
- Short range method for non-destructive testing
- Short range method for destructive testing
- Long range method for non-destructive testing
- Long range method for destructive testing
- The Instantaneous method (one appraiser for equipment variation only).
The two most common method types used and supported by statistical software are the ANOVA method and the average and range method.
Other MSA Components
Other components that might be included in MSA are precision-to-tolerance ratios, measurement capability indices (Cpk for measurement system capability), procedures, plans or protocol, and summary reports.
Important uncertainty contributors to consider when documenting related contextual information include:
- Environment
- The reference element of the measuring equipment
- The measuring equipment and setup
- Software and calculations
- The metrologist
- The measuring object
- The definition of the measurand (standard or perfect / ideal measurement)
- The measuring procedure
- Physical constants
- Intentional bias out of cost or resource concerns. The “operator model” is a relatively recent concept developed by ISO Technical Committee 213 and accounts for intentional uncertainty. This is probably the most overlooked uncertainty contributor
Conclusion
Characterizing measurement error can be a fun, always impresses the customer, and can often result in an important surprise or two. Measuring how your measurements measure up removes guesswork and results in less erroneous conclusions, lower associated risks, improved efficiency, and more productive employees and companies.
References
- A.I.A.G. (1991). “Statistical Process Control (SPC) Reference Manual,” Automotive Industry Action Group, Troy MI.
- Dave Banerjea. “10 Tips for Choosing Gage R&R Software,” Quality Magazine, March 2000
- Henrik S. Nielsen, Ph.D. “Know Your Uncertainty – Understanding and documenting measurement uncertainty is key to gage calibration,” Quality Magazine, April 2000
- John Raffaldi and Steven Ramsier, Ph.D. “5 Ways to Verify Your Gages,” Quality Magazine, March 2000
- “Stability and linearity: Keys to an effective measurement system”
- William H. Woodall. (2000). “Controversies and Contradictions in Statistical Process Control,”Journal of Quality Technology, vol. 32, No. 4, pp. 341-350.