
© Ground Picture/Shutterstock.com
Key Points
- Hypothesis testing provides organizations with a structured approach to evaluate assumptions using data, reducing reliance on intuition and enhancing decision accuracy.
- By validating decisions with statistical evidence, businesses can minimize costly errors and uncertainties, ensuring more reliable outcomes.
- From marketing campaigns and product development to quality control and financial analysis, hypothesis testing helps identify strategies and solutions that yield the best results.
- The method streamlines decision-making processes, making it versatile and applicable across various industries and organizational needs.
In today’s data-driven world, organizations across industries are inundated with information. Transforming this data into actionable insights requires robust analytical tools, one of which is statistical hypothesis testing. This method enables businesses to make decisions based on data rather than intuition, significantly reducing risks and enhancing operational efficiency.
This article explores the concept of statistical hypothesis testing, its process, and how organizations can leverage it to make better business decisions. We’ll also examine real-world examples to illustrate its impact.
What is Statistical Hypothesis Testing?
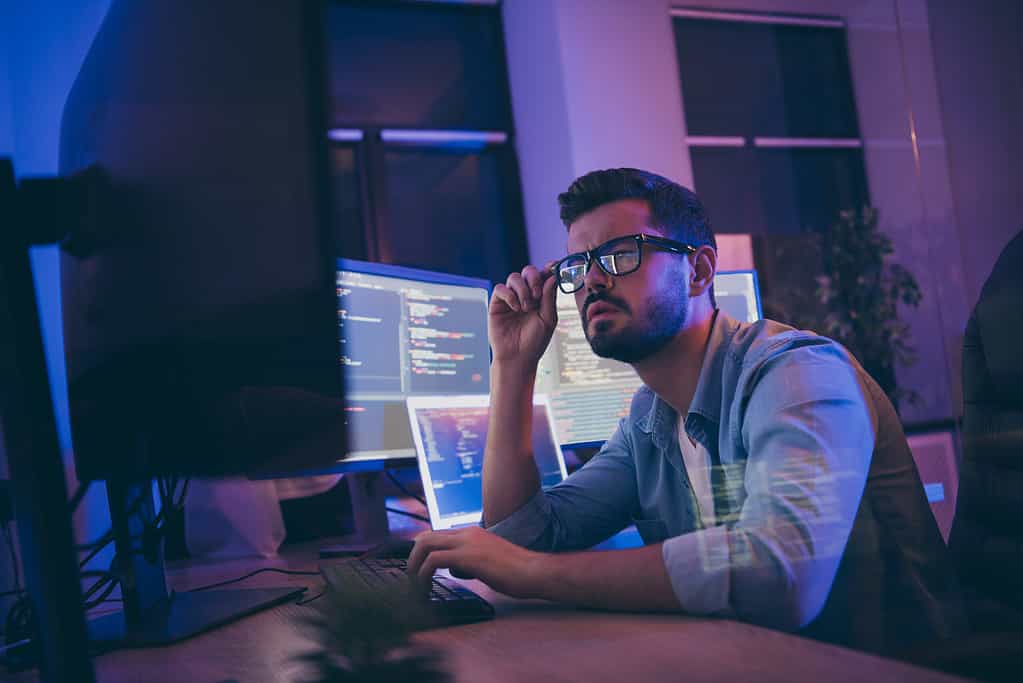
Statistical hypothesis testing is a formal process used to determine whether there is enough evidence in a sample of data to infer a certain condition about a population. At its core, it’s a method to test assumptions (hypotheses) and assess their validity based on observed data.
There are two primary hypotheses involved:
1. Null Hypothesis (H₀): Assumes no effect or no difference. It is the default assumption.
2. Alternative Hypothesis (H₁): Represents what the researcher wants to prove—typically that there is an effect or a difference.
The result of a hypothesis test determines whether the null hypothesis can be rejected in favor of the alternative hypothesis. This is achieved using statistical measures like the p-value, confidence intervals, and test statistics.
Key Steps in Hypothesis Testing
1. Define the Hypothesis: Clearly state the null and alternative hypotheses. For example, in a business context, the null hypothesis might be that a marketing campaign does not improve sales, while the alternative hypothesis concludes that it does.
2. Choose the Appropriate Test: Select a statistical test based on the type of data and hypothesis. Common tests include t-tests, chi-square tests, and ANOVA.
3. Set the Significance Level (α): The significance level represents the probability of rejecting the null hypothesis when it is true (Type I error). A common choice is 0.05.
4. Collect and Analyze Data: Gather data through experiments, surveys, or existing datasets and perform the chosen statistical test.
5. Interpret Results: Based on the p-value and the chosen test statistic, decide whether to reject or fail to reject the null hypothesis.
Common Hypothesis Tests
Here is a comprehensive table of the most common statistical hypothesis tests, their uses, assumptions, and result interpretations:
Test Name | Use Case | Key Assumptions | Result Interpretation |
T-Test (One-Sample) | Test whether the mean of a single sample differs from a known or hypothesized value. | – Data is continuous. – Normally distributed sample. – Random sampling. – Independent observations. | If p-value < α, reject the null hypothesis; the sample mean differs significantly from the hypothesized value. |
T-Test (Two-Sample) | Compare means of two independent groups (e.g., male vs. female). | – Same as above, with the addition: – Variances are equal (for Student’s t-test) or can be unequal (Welch’s t-test). | If p-value < α, reject the null hypothesis; the two group means differ significantly. |
Paired T-Test | Compare means of paired samples (e.g., pre- and post-test scores). | – Differences between pairs are normally distributed. – Data is continuous. – Pairs are dependent but observations are independent. | If p-value < α, reject the null hypothesis; the mean difference between paired observations is significant. |
ANOVA (One-Way) | Compare means of three or more independent groups. | – Normally distributed groups. – Homogeneity of variances. – Observations are independent. | If p-value < α, reject the null hypothesis; at least one group mean differs significantly. |
ANOVA (Two-Way) | Evaluate effects of two independent variables on a dependent variable, including interaction effects. | – Same as One-Way ANOVA. – Interaction effects need proper interpretation. | If p-value < α for main or interaction effects, those factors significantly influence the dependent variable. |
Chi-Square Test | Test for associations between categorical variables. | – Observations are independent. – Expected frequencies in cells > 5 (in most cases). | If p-value < α, reject the null hypothesis; there is a significant association between variables. |
Mann-Whitney U Test | Compare medians of two independent groups (non-parametric). | – Data is ordinal or continuous. – Groups are independent. – Distribution-free. | If p-value < α, reject the null hypothesis; medians of the two groups differ significantly. |
Wilcoxon Signed-Rank Test | Compare medians of paired samples (non-parametric). | – Data is ordinal or continuous. – Pairs are dependent. – Distribution-free. | If p-value < α, reject the null hypothesis; median difference between paired observations is significant. |
Kruskal-Wallis Test | Compare medians of three or more independent groups (non-parametric). | – Data is ordinal or continuous. – Groups are independent. – Distribution-free. | If p-value < α, reject the null hypothesis; at least one group median differs significantly. |
Fisher’s Exact Test | Test for association between categorical variables in small samples. | – Data is categorical. – Observations are independent. – Small sample sizes. | If p-value < α, reject the null hypothesis; there is a significant association between variables. |
Applications of Hypothesis Testing in Business Decision-Making
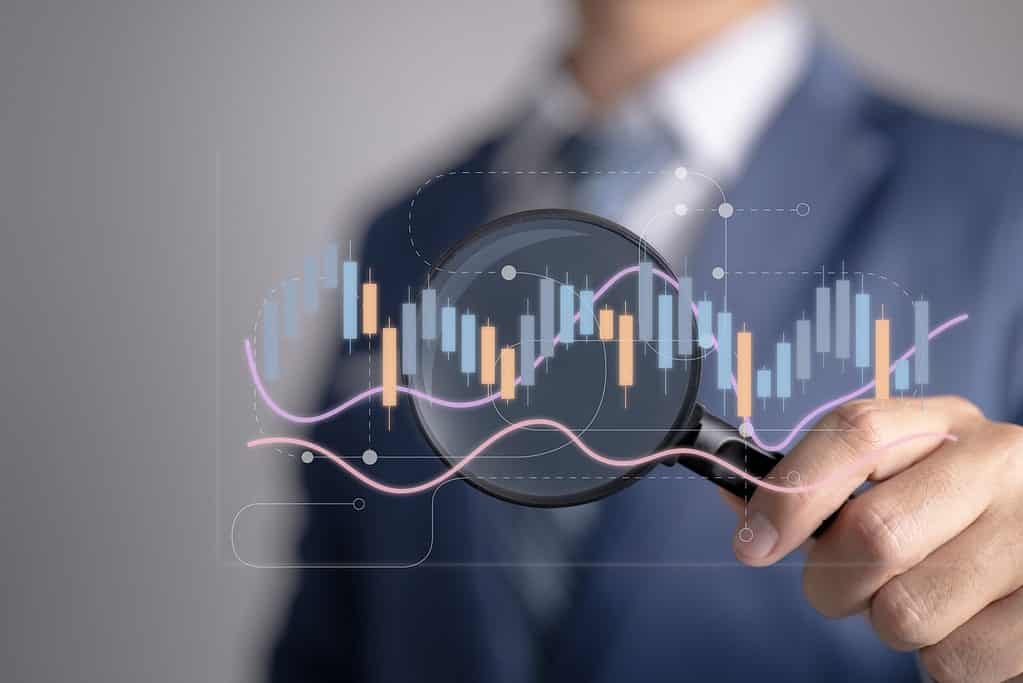
1. Marketing Campaigns
Businesses frequently launch marketing campaigns to attract new customers or increase sales. Hypothesis testing can evaluate the effectiveness of these campaigns.
Example: A retailer launches an email marketing campaign and wants to know if it significantly increases website traffic. Using hypothesis testing, the retailer compares the average daily website visits before and after the campaign. If the p-value is less than the significance level, the retailer can conclude the campaign had a measurable impact.
2. Product Development
Before launching a new product, companies need to assess its potential success. Hypothesis testing can validate assumptions about customer preferences or product features.
Example: A tech company tests two different designs of a mobile app interface. By comparing user engagement metrics, the company determines whether one design performs better than the other.
3. Quality Control
In manufacturing, ensuring product quality is crucial. Hypothesis testing helps identify defects or variations in production processes.
Example: A factory producing light bulbs tests whether the average lifespan of bulbs meets the advertised duration. If the test reveals significant deviation, the company can adjust its production process.
4. Customer Satisfaction
Measuring and improving customer satisfaction is critical for long-term success. Hypothesis testing helps analyze feedback and identify areas for improvement.
Example: A hotel chain implements a new customer service training program and tests its effect on guest satisfaction scores. By comparing scores before and after the program, the chain assesses its effectiveness.
5. Financial Analysis
Hypothesis testing aids in evaluating investment strategies, budget decisions, and financial performance.
Example: A financial analyst tests whether a new investment strategy yields higher returns than the existing approach. If the test results support the alternative hypothesis, the firm can adopt the new strategy.
Case Study
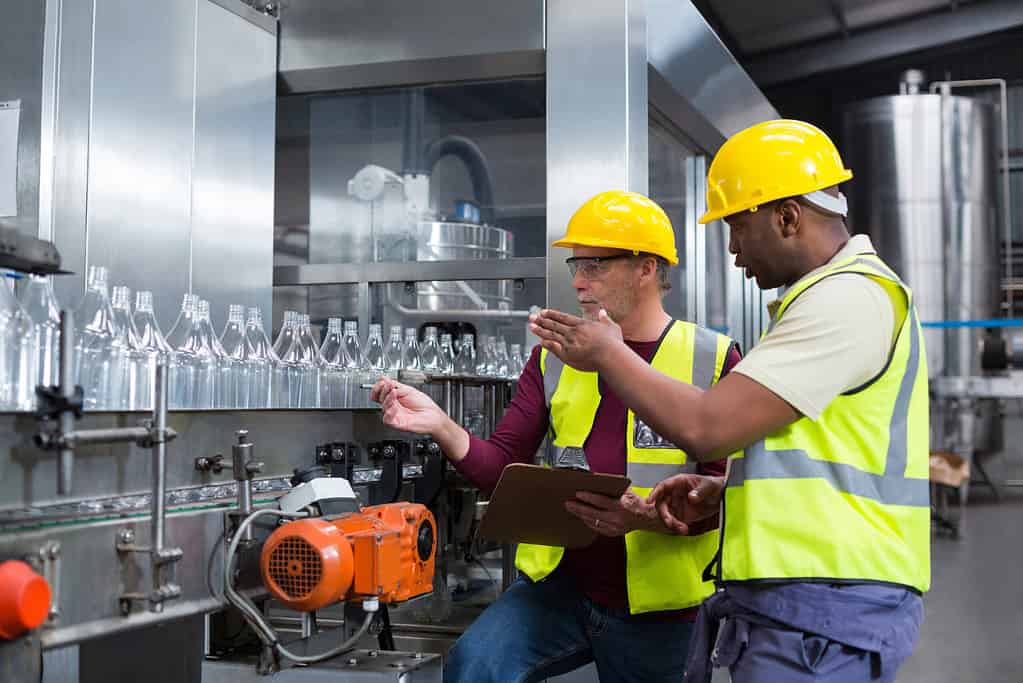
A manufacturing company operates four production lines (A, B, C, and D) to produce their electrical components. Management noticed variations in the output of the lines and decided to investigate whether the differences were statistically significant. Their goal was to identify the best-performing line and assess if adjustments could enhance overall productivity.
Data Collection
Over 20 days, the company recorded daily production volumes (in units) for each line:
- Line A: 102, 108, 110, 104, 106, 107, 109, 111, 105, 108, 112, 110, 107, 109, 113, 114, 108, 110, 115, 117
- Line B: 95, 92, 94, 96, 97, 98, 93, 95, 94, 94, 96, 92, 91, 94, 93, 95, 97, 94, 92, 93, 94
- Line C: 120, 122, 119, 121, 123, 125, 124, 122, 120, 123, 124, 126, 125, 122, 121, 120, 123, 124, 122, 125
- Line D: 88, 85, 87, 86, 89, 90, 88, 86, 87, 89, 88, 90, 85, 86, 88, 89, 87, 85, 86, 88
Hypothesis Testing
The company performed an ANOVA test using statistical software to determine whether the average production volumes of the four lines were statistically different:
- Null Hypothesis (H₀): All production lines have equal average production volumes.
- Alternative Hypothesis (H₁): At least one production line has a different average production volume.
Output
1. Assumption 1 – All data groups are not different than normal. This was tested and confirmed.
2. Assumption 2 – All data groups have equal variances. This was tested and confirmed.
3. Ran an ANOVA to test for difference and a Tukey’s Test to see which lines are different. The conclusion was that there were differences between the four production lines. Below are the results for the ANOVA and Tukey Test.
Interpretation of Results
Since the p-value is 0.000, the null hypothesis is rejected. There is strong evidence to conclude that not all production lines have the same average production volume. From the output, Line C has the highest production output, followed by Line A, Line B, and then Line D.
Business Decisions and Implications
The results helped the company identify Line C as the most efficient production line. Management used this insight to make the following decisions:
- Analyze Best Practices: Investigate the processes, machinery, and workforce efficiency on Line C to replicate its success across other lines.
- Optimize Resources: Allocate more raw materials to Line C to maximize output while addressing inefficiencies in Line D to improve its performance.
- Targeted Improvements: Focus on equipment maintenance and worker training for Lines B and D to bring their performance closer to that of Line C.
- Production Planning: Redesign production schedules to prioritize the most efficient lines during peak demand periods.
By leveraging ANOVA, the company made informed decisions that improved production efficiency and increased overall output, leading to better resource utilization and higher profitability.
Advantages of Using Statistical Hypothesis Testing
1. Data-Driven Decision-Making: Decisions based on statistical evidence are more reliable and less prone to biases.
2. Risk Mitigation: By testing hypotheses, businesses can avoid costly errors and reduce uncertainty.
3. Efficiency: Hypothesis testing streamlines decision-making processes by providing clear guidelines based on statistical evidence.
4. Scalability: The method is applicable across various business functions and industries, making it a versatile tool.
Challenges and Limitations
While statistical hypothesis testing is a powerful tool, it has limitations:
1. Dependence on Data Quality: Poor data collection methods can lead to misleading results.
2. Misinterpretation of Results: Misunderstanding p-values or test statistics can result in incorrect conclusions.
3. Overemphasis on Significance: Focusing solely on statistical significance without considering practical significance may lead to suboptimal decisions.
4. Sample Size Issues: Small sample sizes may lack the power to detect meaningful effects, while excessively large samples can lead to trivial findings being statistically significant.
Best Practices for Organizations
1. Ensure that employees understand the principles of hypothesis testing and can interpret results correctly.
2. Work with data professionals to design robust tests and analyze results.
3. Use hypothesis testing alongside other analytical techniques, such as machine learning or predictive modeling, for comprehensive insights.
4. Consider the real-world impact of statistical findings when making decisions.
Similar Concepts
Hypothesis testing is a core tool for organizations to better understand their data and processes. Below are some additional articles on how hypothesis testing can be valuable in helping make better business decisions.
- This article discusses how to optimize the value of hypothesis testing.
- Mitigating Type 1 Error is the topic of this interesting article.
- Selecting the right statistical test for your data explores hypothesis testing and other techniques.
Conclusion
Statistical hypothesis testing empowers organizations to make informed, evidence-based decisions. By systematically evaluating assumptions and analyzing data, businesses can enhance marketing effectiveness, improve product quality, and optimize operations. While there are challenges, adopting best practices and maintaining a clear focus on both statistical and practical significance can maximize its benefits.
As organizations continue to navigate an increasingly complex business landscape, statistical hypothesis testing remains an indispensable tool for driving success and fostering innovation.
The image featured at the top of this post is ©Ground Picture/Shutterstock.com.