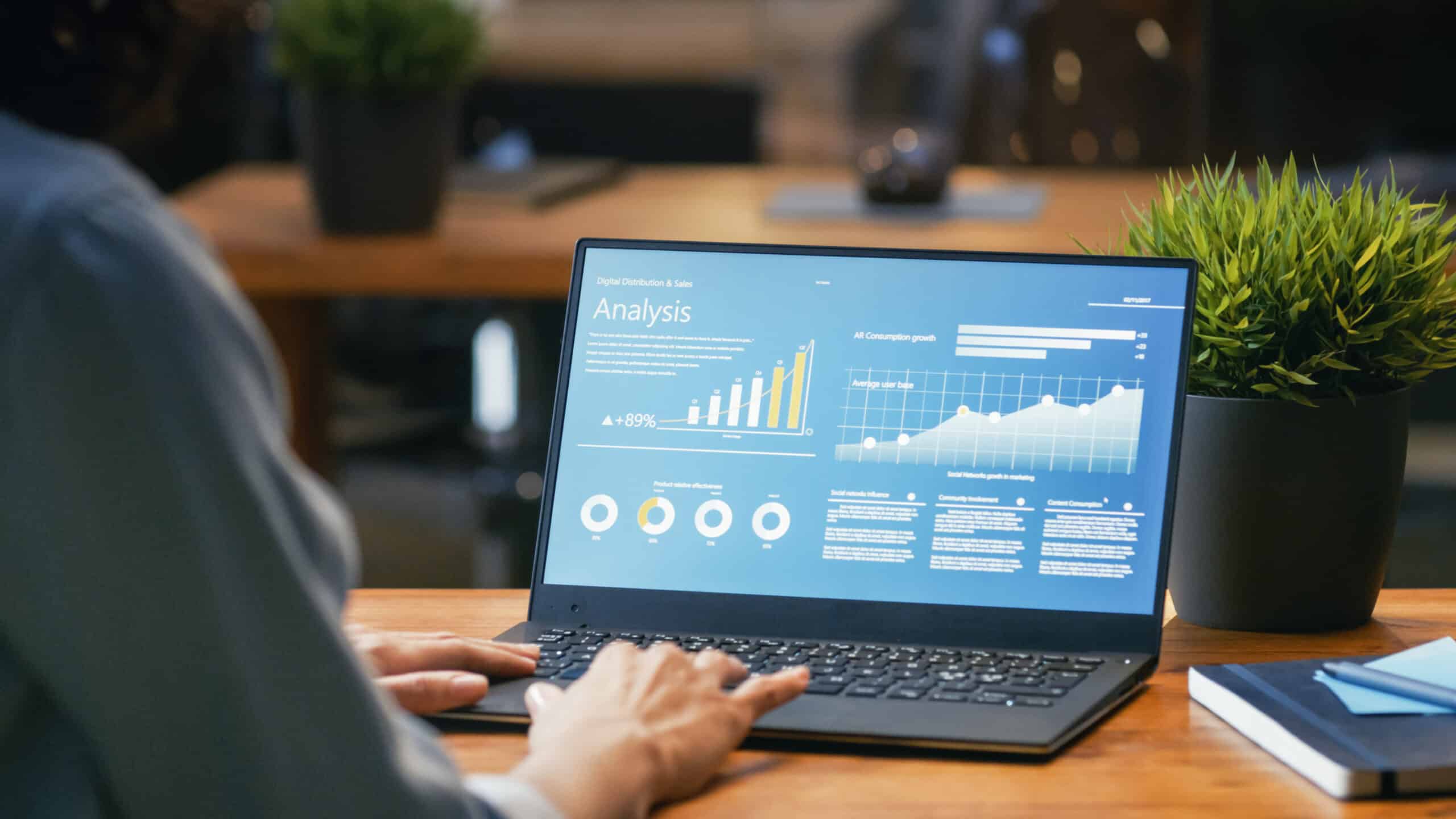
Key Points
- Kruskal-Wallis is a non-parametric test.
- You can use the test with multiple samples at the same time.
- It is a flexible test applicable to any industry or business sector.
The ANOVA analysis of variance test is a fantastic tool when your data follows the normal distribution. Should your data fall outside of this category, you have the Kruskal-Wallis test.
Overview: What is Kruskal-Wallis?
The Kruskal-Wallis test is a nonparametric test that is an analog of the one-way analysis of variance. It is utilized when the measurement variable does not meet the assumptions of the ANOVA test or when a comparison is needed for the outcomes of three or more non-identical groups.
3 Benefits of Kruskal-Wallis
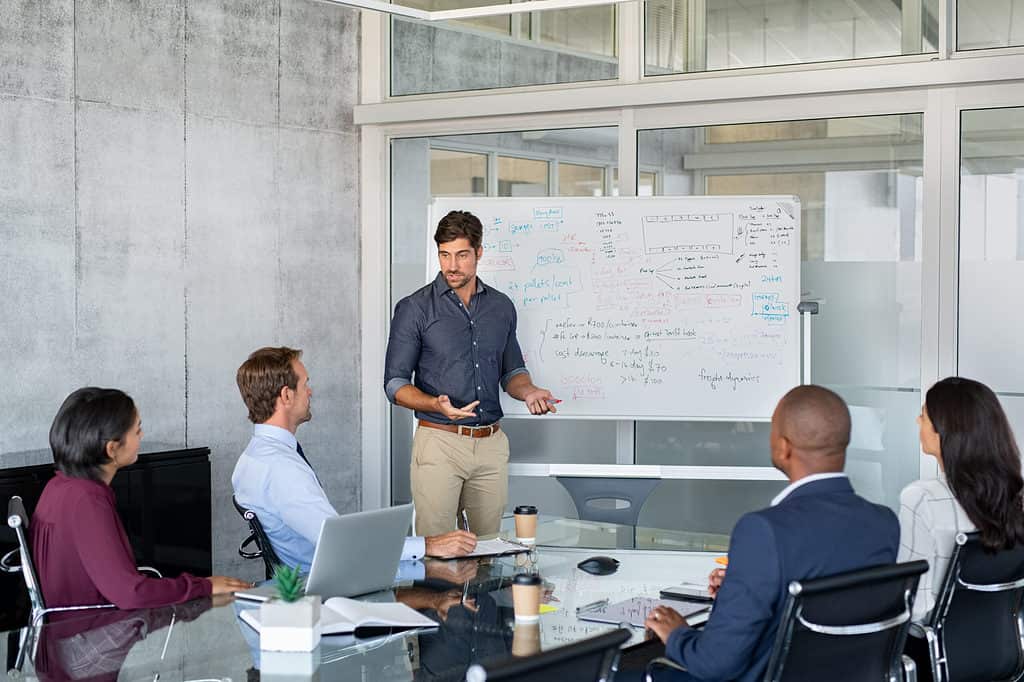
There are some key benefits to the Kruskal-Wallis test:
1. Nonparametric
One benefit of the Kruskal-Wallis test is that it is nonparametric, as data can be pulled from a sample that is not adherent to a specific distribution.
2. Multiple Groups
With the Kruskal-Wallis test, multiple group samples can be tested together.
3. Box-plot Comparison
The test has the advantage that the overall levels of samples can be compared with box-plots visually.
Choosing the Right Tool for the Job
Kruskal-Wallis is a useful test simply because of its flexibility and ease of implementation. Since it is non-parametric, it readily reflects your real-world data points. Knowing how to use an ANOVA test is great, especially for more involved analysis. However, there is a time and a place for the Kruskal-Wallis.
Why Is It Important to Understand?
Understanding a Kruskal-Wallis test is important for the following reasons:
Applicable to Any Industry
It is worth understanding the test in any industry since it applies to all whenever there is a need to understand the dependent variable should it have at least three independent groups.
ANOVA Is Not Always the Best Option
Understanding how to use the test is important because you cannot always rely on the ANOVA test. An example would be when the data is skewed.
Determining if a Group Originates from Others
Having a working knowledge of the test provides you with a helpful tool that you can utilize when you need to determine if a group comes from a different origin.
An Industry Example
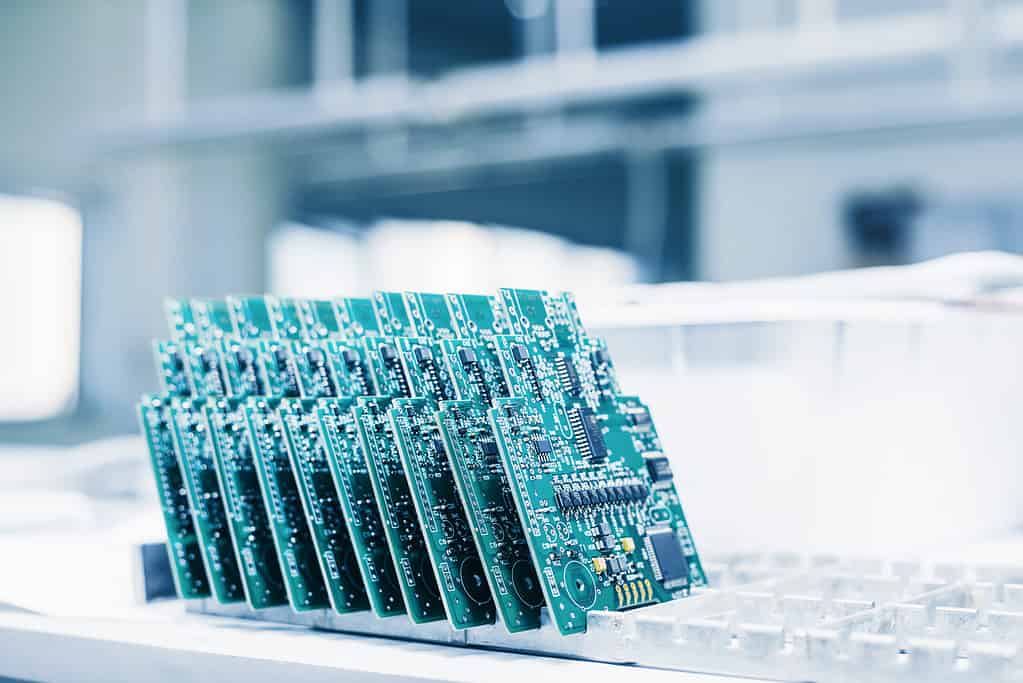
A manufacturing company has four different plants. Teams of operators from all four locations are given safety tests with a new machine that will be utilized at all four branches of the company. The hope is that the teaching methods are the same at all locations and that the scores will fall within the same range.
Once the scores come in, the Kruskal-Wallis test is utilized, and it is determined that there is likely some variance in testing scores and that there was some differentiation in the teaching method at at least one of the plants.
3 Best Practices
Here are some tips to keep in mind when working with the Kruskal-Wallis test:
1. Groups and sample size
It is typically used with at least three groups. It can, however, work with only two. There should be a sample size of 5 or more for each group.
2. Reject the null hypothesis
It can tell you that at least one group originates from a different distribution if there is a different median, but it is unable to tell you which of the groups are.
3. Failure to reject the null hypothesis
If all your groups have the same median, it is safe to say that all groups originate from the same distribution.
Other Useful Tools and Concepts
You’ve got plenty of tools at your disposal when it comes to statistical analysis. While the test we’ve mentioned applies to multiple scenarios, you might need more information on non-parametric testing. Our handy guide covers the how and why of these tests for your analysis.
Additionally, you might need to pay close attention to turnaround time. This measurement is useful for eliminating waste and meeting your customer expectations.
Having Kruskal-Wallis as one of your tools
Having the Kruskal-Wallis test as part of your toolbox is essential when the ANOVA test does not apply. After the Kruskal-Wallis test, you have several options when it comes to post hoc tests. The most common would be the Dunn test, the Pairwise Wilcoxon test, the Conover test, or the Nemenyi test.