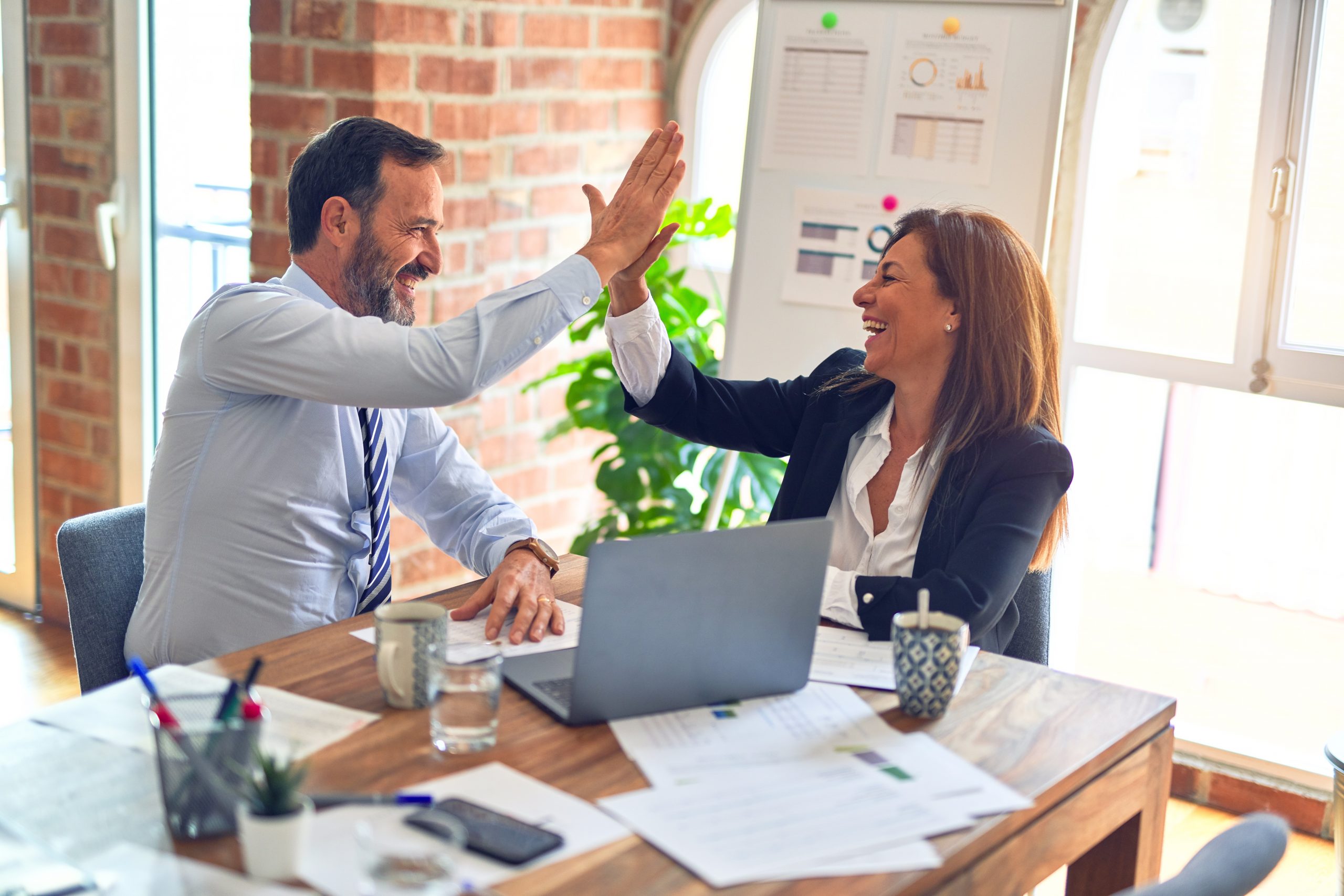
Blocking and randomization are terms used in Design of Experiments (DOE). This article will describe the process of blocking versus randomizing, when and why you will want to use blocking, and some best practices for using it.
Overview: What is blocking?
The purpose of a DOE is to determine what effect or impact your input variables (or factors) have on your output (or response) variable. The first step is to identify the possible factors you believe have a significant effect on your response variable. Using DOE, you will test those factors to understand which, if any, can cause your response to change.
To get the most out of your experiment, you want to minimize the noise or influence of other factors that may exist in your experimental environment but are not of interest to you.
For example, you want to determine the effect of machine speed, temperature, and pressure on the response variable of lamination integrity of a glass sheet. To minimize the influence of other factors than those three, you should take your measurements under a consistent experimental condition. Unfortunately, you need to do your experiment across the three shifts the plant operates.
Therefore, shift becomes another factor you would define as a nuisance factor.
A nuisance factor is a factor you believe has some effect on the response, but it’s of no interest to you right now. However, the variability it transmits to the response needs to be minimized. Typical nuisance factors include batches of raw material, operators, pieces of test equipment, and time (shifts, days, etc.).
Here are the conditions when you would use blocking versus randomization:
- If the nuisance factor is known and controllable, you will use blocking.
- If the nuisance factor is unknown and uncontrollable, you would call it a lurking variable. Here you would use randomization to try and balance out its impact across the experiment.
The general rule is to block what you can, and randomize what you can’t. Said another way, blocking should always be used to account for the effects of nuisance factors if it is not possible to hold the nuisance factor at a constant level through all of the experimental runs.
Randomization should be used within each block to counter the effects of any unknown variability that may still be present. You can also just randomize the runs of your desired factors to spread out the effect of the lurking variable.
A block is a categorical factor that explains variation in the response variable not caused by the factors you are interested in learning about. To distinguish between any block effect (incidental differences between shifts) and effects because of the experimental factors (machine speed, temperature, and pressure), you must include the block (shift) in the designed experiment.
3 benefits of blocking
Your DOE can be sensitive to nuisance and lurking variables. Blocking will give you these benefits.
1. Identifies the effect of the nuisance/lurking variable
If your block does not show statistical significance, you can be confident it has little effect on your response.
2. Eliminates contamination
The experimental noise and contamination of nuisance variables can be minimized by using blocks.
3. Increases significance of factors on your response
Allows for the true effect and variation of your factors of interest to be known by reducing the variation attributable to nuisance variables.
Why is blocking important to understand?
Nuisance variables may be part of the natural experimental environment. It is important to know how to mitigate their impact on the results of your DOE.
Not always possible to eliminate nuisance variables
There will be factors in your experimental environment you can’t eliminate. Some will have more impact than others. Use blocking to understand the most important nuisance variables.
Significance of the factors you are interested in
Nuisance variables will cause additional variation in the interpretation of your experiment. By blocking and mitigating the unwanted variation, you will be able to distinguish which of your factors of interest are truly significant.
Blocking versus randomization
You need to understand when to block and when to randomize. The key is whether the nuisance variable is known and controllable or not.
An industry example of blocking
Hartford Biogenics conducted an experiment to test a new vaccine against the common cold. To test the vaccine, Hartford recruited 1,000 volunteers equally split between 500 males and 500 females. The participants ranged in age from 21 to 70.
At first, the researcher suggested doing a randomized design in which the participants would be randomly assigned to receive the vaccine or a placebo. A completely randomized design is shown below.
In this design, the researcher randomly assigned participants to receive either the placebo or the vaccine. The same number of participants (500) were assigned to each treatment condition. The response variable was the number of colds reported over 6 months. If the vaccine was effective, participants should have reported significantly fewer colds than those who got the placebo.
But the researcher had second thoughts because he knew there were physiological differences between men and women. He didn’t know whether to consider that in his experiment and whether to call it a lurking or nuisance variable. His randomized design allowed for the control of the effects of the possible lurking variable, gender. By randomly assigning subjects to treatments, the researcher assumed the effect of the lurking variable would be mitigated and spread across the experiment.
Upon further reflection, the researcher decided it would be better to go with a randomized block design where he divided participants into subgroups called blocks. The participants within each block were then randomly assigned to the two treatment conditions. Because this design reduces variability and potential confounding, it would result in a better estimate of treatment effects.
The table below shows a randomized block design.
Participants were assigned to blocks based on gender. Then, within each block, participants were randomly assigned to treatments. For this design, 250 men get the placebo, 250 men get the vaccine, 250 women get the placebo, and 250 women get the vaccine.
As a result, differences between treatment conditions would not be attributed to gender. This randomized block design removed gender as a potential source of variability and as a potential confounding variable. Unfortunately, the results from the vaccine were not significantly different from the placebo, so the study was discontinued — and we still catch colds.
3 best practices when thinking about blocking
The lack of blocking is a common flaw in using designed experiments. These tips should help you get better results from your DOE.
1. Don’t block everything
Only block the most important nuisance variables, otherwise your experiment will get long and more complex.
2. Set up your experiment to eliminate contamination and noise
During your initial design, try to create homogeneous conditions so you don’t have to worry about the effect of nuisance variables.
3. Use blocking in your screening experiment
A screening DOE is used to narrow down the possible factors by using a limited amount of runs. By also using blocks, you may be able to eliminate nuisance variables that might not be significant.
Frequently Asked Questions (FAQ) about [primary keyword]
1. Can blocking be used for fractional factorial experiments?
Yes. Blocking can be used for full factorial and fractional factorial experiments.
2. Can I use center points with blocking?
Yes. Center points are used with numeric factors and are represented as the midpoint between a high and low numeric level. It is used to determine if the relationship is linear when going from the low to high setting in your experiment.
If the design includes more than 1 block, you can add the number of center points that you specified to each block. For example, if you specify 2 center points per block and 2 blocks to your design, and the factors are numeric, this will result in 2 center points in block 1 and 2 center points in block 2.
3. When do I use blocking versus randomization in my DOE?
Blocking is used when your nuisance variable is known and controllable. Randomization is used when your nuisance variable is unknown and uncontrollable. As the saying goes, block what you can, and randomize what you can’t.
Blocking summary
Blocking is a technique in DOE used to mitigate the impact nuisance variables could have on the interpretation of the effects of the factors you are interested in. If your unwanted nuisance variables are known and controllable, you will use blocking. If they’re unknown and uncontrollable, you will use randomization of your runs and hope this spreads out the impact of the lurking variables.