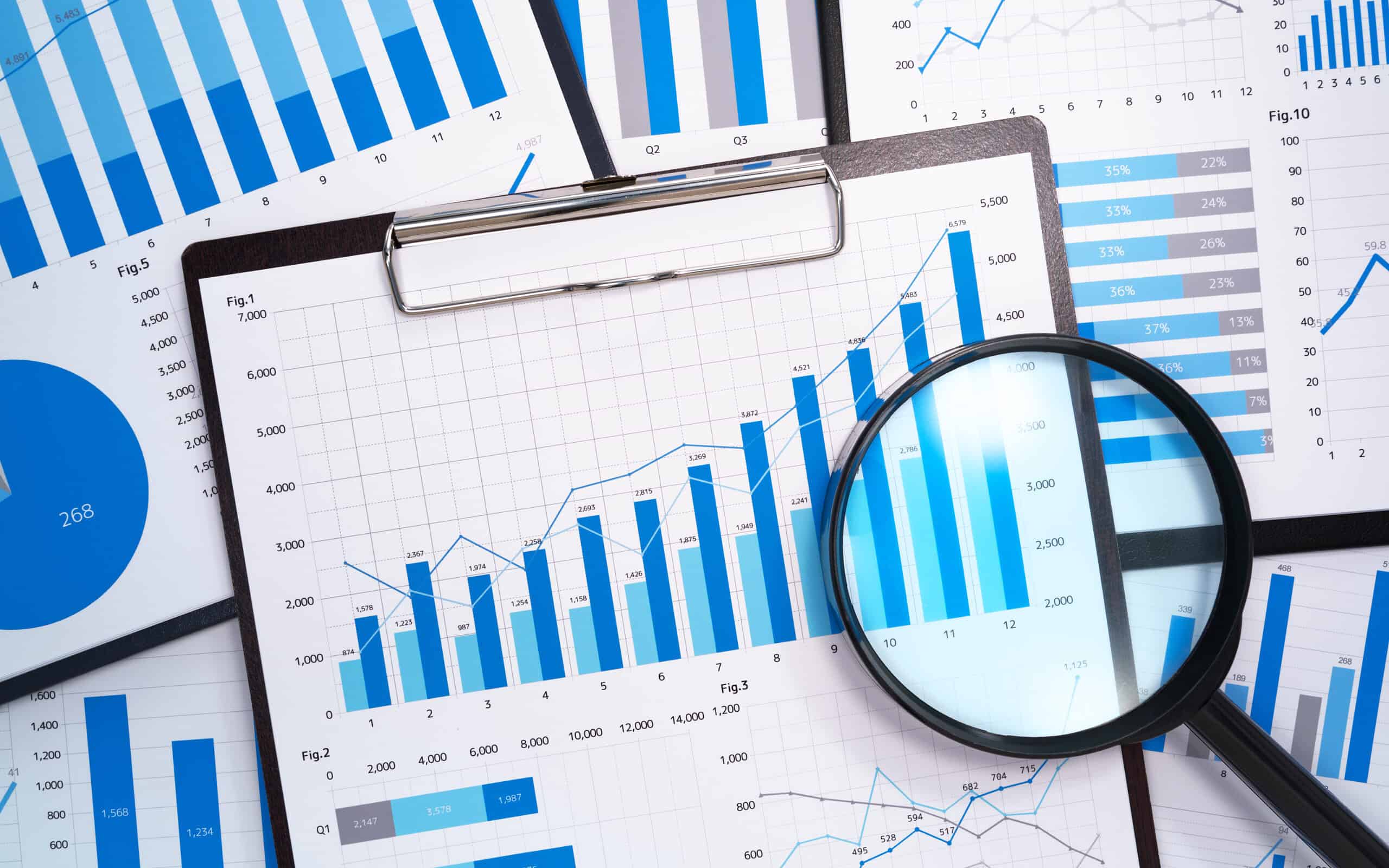
Hypothesis testing is a branch of statistics in which, using data from a sample, an inference is made about a population parameter or a population probability distribution.
First, a hypothesis statement and assumption is made about the population parameter or probability distribution. This initial statement is called the Null Hypothesis and is denoted by Ho.
An alternative or alternate hypothesis (denoted Ha) is then stated, which will be the opposite of the Null Hypothesis.
The hypothesis testing process and analysis involves using sample data to determine whether or not you can be statistically confident that you can reject or fail to reject the Ho. If the Ho is rejected, the statistical conclusion is that the alternative or alternate hypothesis Ha is true.
Overview: What is the Alternate Hypothesis (Ha)?
Hypothesis testing applies to all forms of statistical inquiry. For example, it can be used to determine whether there are differences between population parameters or an understanding about slopes of regression lines or equality of probability distributions.
In all cases, the first thing you do is state the Null Hypothesis. The word “null” in the context of hypothesis testing means “nothing” or “zero.”
If we wanted to test whether there was a difference in two population means based on the calculations from two samples, we would state the Null Hypothesis in the form of:
Ho: mu1 = mu2 or mu1 – mu2 = 0
In other words, there is no difference, or the difference is zero. Note that the notation is in the form of a population parameter, not a sample statistic.
The analysis of the Null Hypothesis is designed to test the Null, which will determine whether the Null should be rejected so that the Alternate Hypothesis is defaulted to and assumed to be true, or not to reject the Null so it is assumed to be the true condition.
A classic example is when you get the results back from your doctor after taking a blood test. The Null is written to state that there is no infection. Remember, the Null is always in the form of “nothingness.” The alternate hypothesis is that you have an infection. Once the test is done, the lab will determine whether the Null can be rejected or not. If the test shows an infection, the Null will be rejected, and the Alternate will be assumed to be true. If the test shows no infection, we cannot reject the Null.
While the Null can only be written in one form — “equal to” or “no difference” — the Alternate can be written for three conditions. For example, a marketing director wants to improve sales. She designs and launches a new social media campaign, collecting sample data for sales activity prior to the new campaign. After six months, sample sales data was collected to determine whether the campaign was successful. Hypothesis testing was used to statistically confirm whether the campaign was successful or not. The Null Hypothesis was written as: Ho: muBefore = muAfter, where the claim was that the population average sales before the campaign is the same as the population average sales after the campaign. In other words, the campaign had no effect on sales.
The Alternate Hypothesis can now be written in one of three forms:
- muBefore does not equal muAfter: The average of the population average sales before is not equal to the population average sales after, but you don’t know if it was more or less. You would have to look at the actual numbers to understand this result.
- muBefore less than muAfter: In this form, if you reject the Null, your conclusion will be that the sales after the campaign are greater than before the campaign. Put another way, the campaign increased average sales.
- muBefore greater than muAfter: Again, if you reject the Null, the alternate says that average sales before were greater than after the campaign. The campaign seems to have been a failure.
3 benefits of the Alternate Hypothesis
The stating and testing of the Null and the default to the Alternate hypothesis is the foundation of hypothesis testing. By doing so, you set the parameters for your statistical inference.
1. There can be a statistical assurance of determining differences between population parameters
Just looking at the mathematical difference between the means of two samples and making a decision is woefully inadequate. By statistically testing the Null hypothesis, you will have more confidence in any inferences you want to make about populations based on your samples. If you reject the Null, you’ll know which Alternate is most appropriate.
2. Statistically based estimation of the probability of a population distribution
Many statistical tests require assumptions of specific distributions. Many of these tests assume that the population follows the normal distribution. If it doesn’t, the test may be invalid. Rejecting the Null will establish whether the estimated distribution fulfills any test assumption or not.
3. You can assess the strength of your conclusions as to what to do with the Alternate hypothesis
Hypothesis testing calculations will provide some relative strength to your decisions as to whether you reject or fail to reject the Null hypothesis and, therefore, the Alternate.
Why is the Alternate Hypothesis important to understand?
It is the interpretation of the statistics relative to the Null and Alternate hypotheses that is important.
Properly write the Alternate hypothesis to capture what you are seeking to prove
The Alternate can take the form of “not equal,” “less than,” or “greater than.”
Frame your statement and select an appropriate alpha risk
You don’t want to place too big of a hurdle (or burde)n on your decision-making relative to action on the Null hypothesis by selecting an alpha value that is too high or too low. The Alternate can really reflect the true condition of the population, so failing to reject the Null too often can mask the truth.
Knowing there are decision errors when deciding how to respond to the Null Hypothesis
Since your decision relative to rejecting or not rejecting the Null impacts the Alternate Hypothesis, it’s important to understand how that decision works.
An industry example of using the Alternate Hypothesis
The director of manufacturing at a medium-size window manufacturer recently had an older machine retrofitted to increase run speed of the equipment. The supplier, after doing the retrofit, claimed that the machine was now running significantly faster. He showed a comparison of the sample average run speed before the retrofit and the sample average run speed after the retrofit. Looking at the two averages, it appeared that the supplier was correct and that the retrofit did indeed increase the speed.
However, having had some training in Lean Six Sigma, the director asked his local Black Belt for some help in doing a hypothesis test on the data to see if there was truly a statistically significant improvement of at least 100 rpm. The Null hypothesis was written as:
mu1-mu2= -100 rpms
That is the Before speed minus the After speed equaled -100 rpms. This was slightly different than the format he was used to seeing, where the Null would be reflective of “no difference” rather than a value of interest.
The Alternate hypothesis was written to reflect an increase of at least 100 rpms. That form was written as:
mu1 – mu2 greater -100
That would mean the After speed was more than 100 rpms faster than the Before speed. Fortunately, the results indicated that the Null hypothesis should be rejected and that in actuality, the difference was greater than the 100 rpms he wanted.
3 best practices when thinking about the Alternate Hypothesis
Using hypothesis testing to help make better data-driven decisions requires that you properly address the Null and Alternate Hypotheses.
1. Always use the proper nomenclature when stating the Alternate Hypothesis
The Alternate should be in the form of “not equal to,” “greater than,” “less than,” or “equal to some value of interest.”
2. Be sure that the Alternate statement reflects what you want to learn about the process characteristics
The writing of the Alternate Hypothesis can vary, so be sure you understand exactly what condition you are testing against.
3. Pick a reasonable alpha risk so you are not always failing to reject the Null Hypothesis
Being too cautious will lead you to not reject the Null enough so you will never learn anything about your population data.
Frequently Asked Questions (FAQ) about the Alternate Hypothesis
What form should the Alternate Hypothesis be written in?
The Alternate Hypothesis should be in the form of “not equal to,” “greater than,” “less than,” or “equal to some value of interest.”
When do you default to the Alternate Hypothesis?
If your analysis of the sample data suggests that you should reject the Null Hypothesis, you will default to the statement of the Alternate Hypothesis.
Should I be disappointed if I default to the Alternate Hypothesis?
Usually no. Typically, you want the actions you took to have had some impact or effect. Rejecting the Null and defaulting to the Alternate signals that something did, in fact, happen — and that might be a good thing.
Using an Alternate Hypothesis (Ha)
The Alternate Hypothesis is the default should you reject the Null. It is an indication that something has happened that is significant. Often, that is what you want to see if you’re comparing a before and after situation.
While the form of the Null Hypothesis is usually written in a single format, the format of the Alternate can be written a number of different ways. This provides more sensitivity to your interpretation of the population data and will therefore provide a richer insight for decision-making.