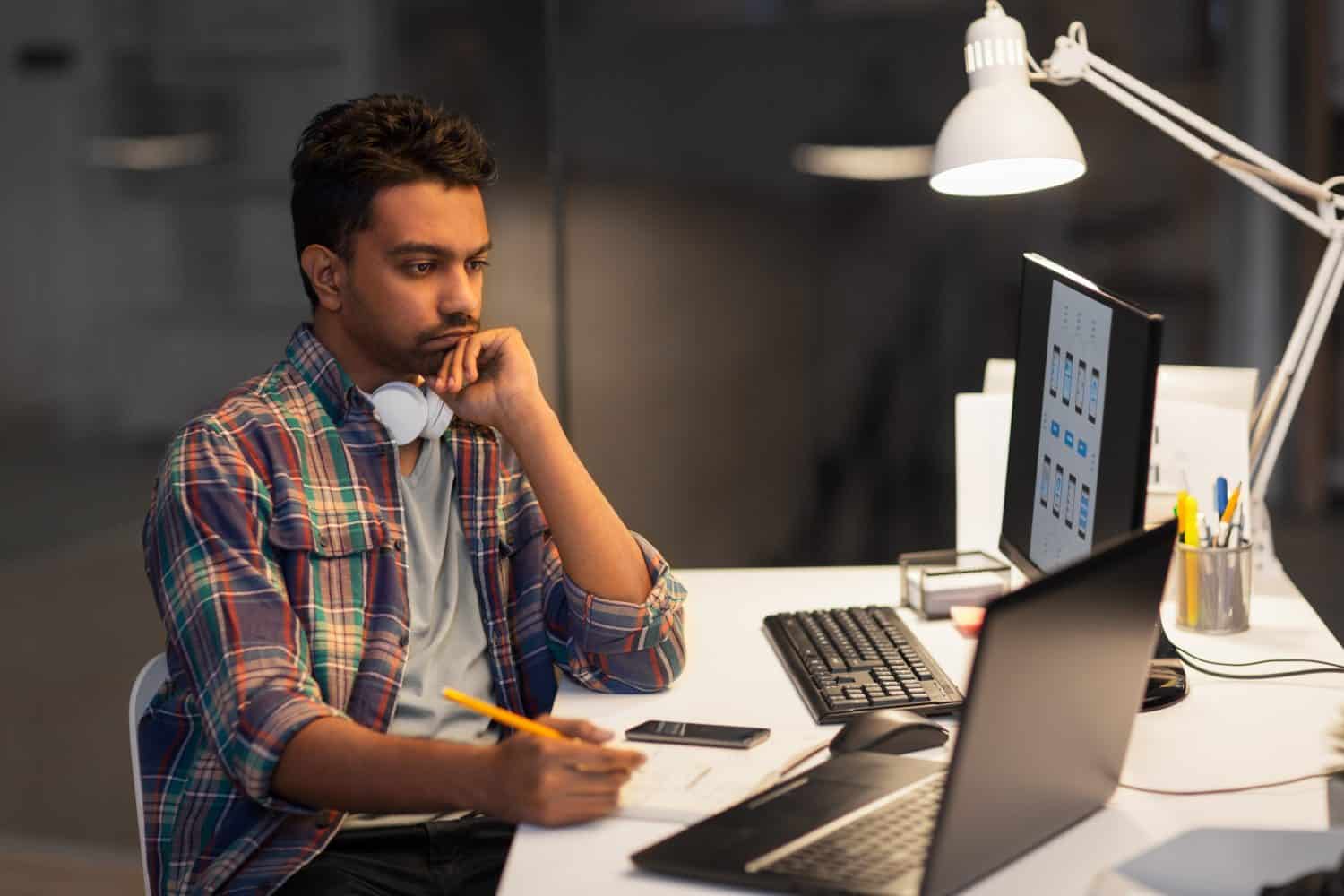
© Ground Picture/Shutterstock.com
How can the fractional factorial design of experiments benefit you?
A global beverage company faced a persistent issue in its production line: excessive foaming while bottling 1-liter apple juice bottles. The foaming caused significant inefficiencies, including improperly filled bottles, contamination risks, and increased rework due to rejected products. Despite attempts to address the problem through traditional trial-and-error methods, the results were inconsistent, leading to an annual loss of approximately $798,000 in wasted product and labor.
Recognizing the need for a systematic approach, the company turned to advanced statistical techniques. By leveraging a fractional factorial design of experiments (DOE), the team sought to identify and optimize the critical factors influencing foaming thereby reducing waste and improving production efficiency.
What is a Fractional Factorial Design of Experiments?
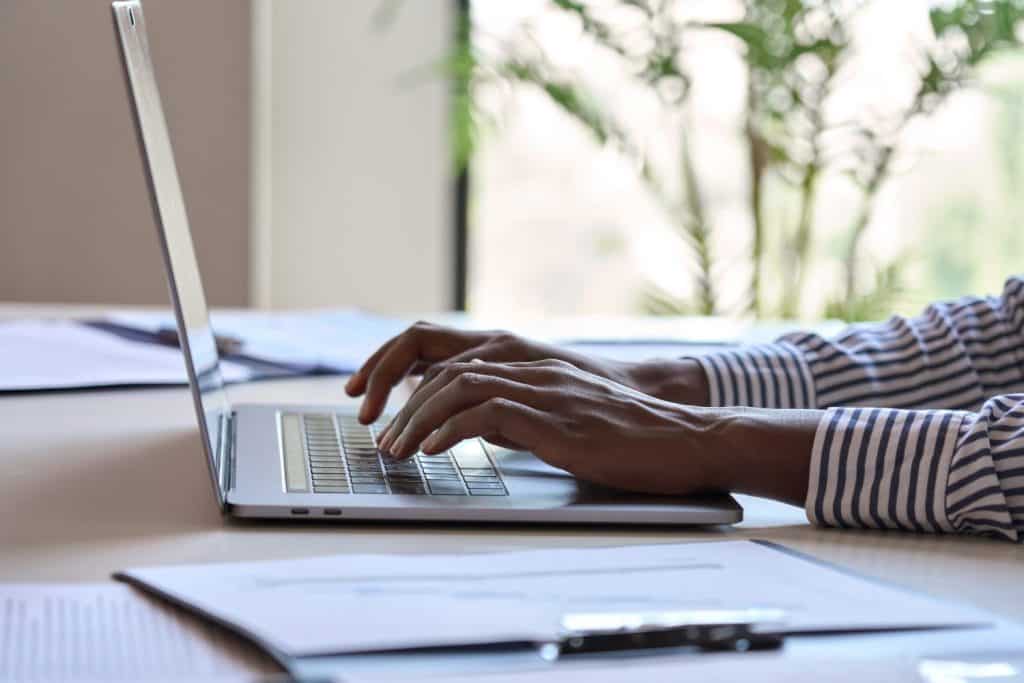
©Ground Picture/Shutterstock.com
A fractional factorial design of experiments is a statistical method used to study multiple factors simultaneously while minimizing the number of experimental runs. Unlike a full factorial design, which evaluates all possible combinations of factors and levels, a fractional factorial design focuses on a strategically chosen subset. This approach allows researchers to explore the most critical interactions between factors without the excessive resource requirements of a full factorial experiment.
Fractional factorial designs are particularly useful in industrial applications where numerous variables, such as temperature, pressure, flow rates, and ingredient proportions influence processes. By analyzing these variables systematically, companies can uncover the most significant contributors to a problem and make data-driven decisions.
What They Did
To tackle the foaming issue, the beverage company assembled a cross-functional team comprising production engineers, quality control specialists, and statistical experts. They followed a structured approach:
1. Define the Problem and Objectives
The team identified excessive foaming during bottling as the primary issue and set a clear objective: reduce the foaming-related rejection rate by at least 50% within six months. They also defined measurable outcomes, such as the percentage of rejected bottles and the associated cost savings.
2. Identify Potential Factors
A brainstorming session with production staff and engineers identified several factors potentially influencing foaming:
- Filling speed: Higher speeds might increase turbulence, causing more foam.
- Nozzle design: Different designs might affect the flow dynamics.
- Juice temperature: Cooler liquids tend to foam less.
- Tank pressure: Variations in pressure could impact flow uniformity.
- Degassing time: Insufficient degassing might leave excess air in the juice.
- Surfactant levels: Natural surfactants in apple juice might vary depending on the batch.
3. Select a Fractional Factorial Design
Given the large number of factors, a full factorial design would have required dozens of experiments, consuming time and resources. Instead, the team opted for a fractional factorial design, choosing a 26−2 design, which required only 16 experimental runs instead of 64. This design allowed them to study the main effects and some two-factor interactions.
4. Conduct Experiments
The team systematically altered the levels of the six factors according to the experimental design. Each run involved bottling a set quantity of apple juice, measuring foaming levels, and recording the rejection rate. To ensure consistency, they controlled for environmental variables, such as humidity and room temperature.
5. Analyze the Data
Using statistical software, the team analyzed the results to identify the most significant factors and interactions. The analysis revealed three critical insights:
- Filling speed and juice temperature were the most significant individual contributors to foaming.
- An interaction between tank pressure and nozzle design also played a significant role.
- Other factors, such as degassing time and surfactant levels, had minimal impact within the tested ranges.
6. Implement and Validate Changes
Based on these findings, the team implemented changes to the production line:
- Reduced the filling speed by 15%.
- Adjusted juice temperature to a consistent 5°C.
- Optimized nozzle design to minimize turbulence.
- Standardized tank pressure settings to complement the new nozzle configuration.
After implementing these changes, the team monitored the production line for three months to validate the improvements.
Outcomes
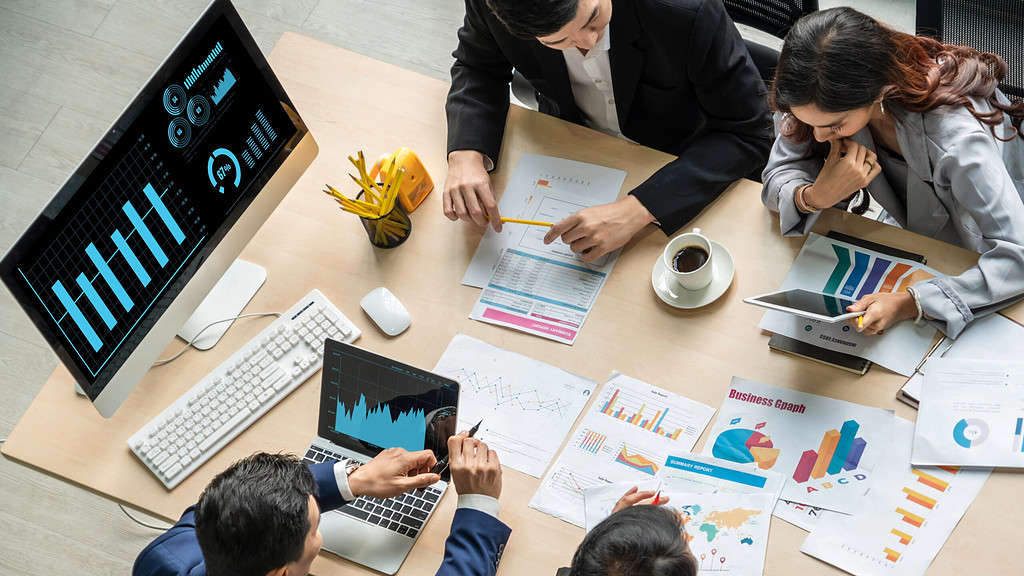
The application of fractional factorial design of experiments led to remarkable results. The rejection rate due to foaming dropped by 85%, far exceeding the initial target of 50%. This improvement translated into several tangible benefits:
- Reduced Waste: Annual product waste decreased significantly, resulting in cost savings of $798,000.
- Improved Efficiency: Fewer rejected bottles reduced the need for rework and allowed the production line to operate more efficiently.
- Consistent Quality: Bottles were filled uniformly, enhancing product quality and customer satisfaction.
- Scalable Insights: The knowledge gained from the experiment was documented and shared across other production lines and facilities, enabling similar optimizations for different beverages.
The success of this initiative also reinforced the value of using statistical methods to solve complex industrial problems. By embracing a data-driven approach, the company demonstrated its commitment to innovation and operational excellence.
Conclusion
The use of fractional factorial design of experiments proved to be a game-changer for the company. It allowed the team to systematically identify and address the root causes of foaming, leading to substantial cost savings and operational improvements. Beyond solving the immediate problem, the project underscored the importance of statistical thinking in modern manufacturing, setting a precedent for future optimization efforts.
The image featured at the top of this post is ©Ground Picture/Shutterstock.com.