
© ESB Professional/Shutterstock.com
Background
When FreshSense, a mid-sized packaged goods company, decided to launch a new line of healthy snack bars, excitement buzzed through the office. The team knew the market was ripe for innovation, but the challenge lay in determining the perfect combination of attributes for their new product. Color theme, graphics, package size, graphic orientation, price, and shelf placement were all critical factors.
Traditionally, FreshSense would rely on their marketing team to select a few “best” combinations based on intuition and experience, and then test those in select markets. But this time, they opted for a different approach—a data-driven technique known as conjoint analysis.

©ESB Professional/Shutterstock.com
Conjoint analysis, a statistical method that helps determine how people value different features of a product, was chosen to guide the decision-making process. It promised to provide insights into customer preferences, uncover interactions between factors, and identify the optimal combination of attributes for the new snack bars. This story follows FreshSense’s journey through the conjoint analysis process and hypothesizes the results that helped shape their product launch strategy.
What They Did
Step 1: Defining the Problem and Attributes
FreshSense’s marketing team began by clearly outlining the problem: they needed to identify which combination of product attributes would resonate most with their target customers. They then defined the attributes and levels to test:
- Color Theme: Solid blue or mixed colors
- Graphics: Traditional or 3D
- Package Size: 8 bars or 16 bars
- Graphic Orientation: Horizontal or vertical
- Price: $3.99 or $6.99
- Location in Store: End cap or specialty shelf
The team recognized that each of these attributes could influence customer perception and purchase decisions, but they needed to understand their relative importance and any potential interactions.
Step 2: Designing the Conjoint Analysis
To execute the conjoint analysis, FreshSense’s data analytics team designed a six-factor, two-level fractional factorial design. This design would allow them to test a representative subset of all possible attribute combinations without needing to exhaustively test every one of the 64 (2^6) possibilities.
Using statistical software, the team generated a Design of Experiments (DOE) fractional factorial design that included 16 combinations of the attributes. This reduced design maintained a balance of all factors while minimizing the number of combinations to test, saving both time and resources. Each combination was presented as a hypothetical product profile that could be tested with potential customers.
Step 3: Collecting Data from Customers
The marketing team organized a study to collect feedback on the 16 product profiles. They recruited 200 participants who represented their target audience: health-conscious consumers aged 25-45. Each participant was shown the 16 profiles in random order and asked to rate their likelihood of purchasing each product on a scale of 1 to 10.
To avoid bias, the profiles were presented with a consistent layout, and participants were instructed to consider each product independently. The team ensured that all factors, from the use of color themes to the presentation of prices, were clear and comprehensible.
Step 4: Analyzing the Results
Once the data was collected, FreshSense’s analytics team ran the DOE analysis to quantify the importance of each attribute and its levels. The analysis revealed how each factor influenced customer preferences and whether there were any significant interactions between factors.
The regression coefficients were used to calculate part-worth utilities, numerical values that represent the relative preference for each level of an attribute. For example:
- Color Theme: Customers slightly preferred mixed colors over solid blue.
- Graphics: 3D graphics were significantly more appealing than traditional designs.
- Package Size: The larger 16 bar package size was favored, particularly by families.
- Graphic Orientation: Horizontal orientation slightly outperformed vertical.
- Price: As expected, $3.99 was much more appealing than $6.99, but certain premium combinations justified the higher price.
- Location in Store: Specialty shelf placement edged out end cap placement, as customers associated it with higher-quality products.
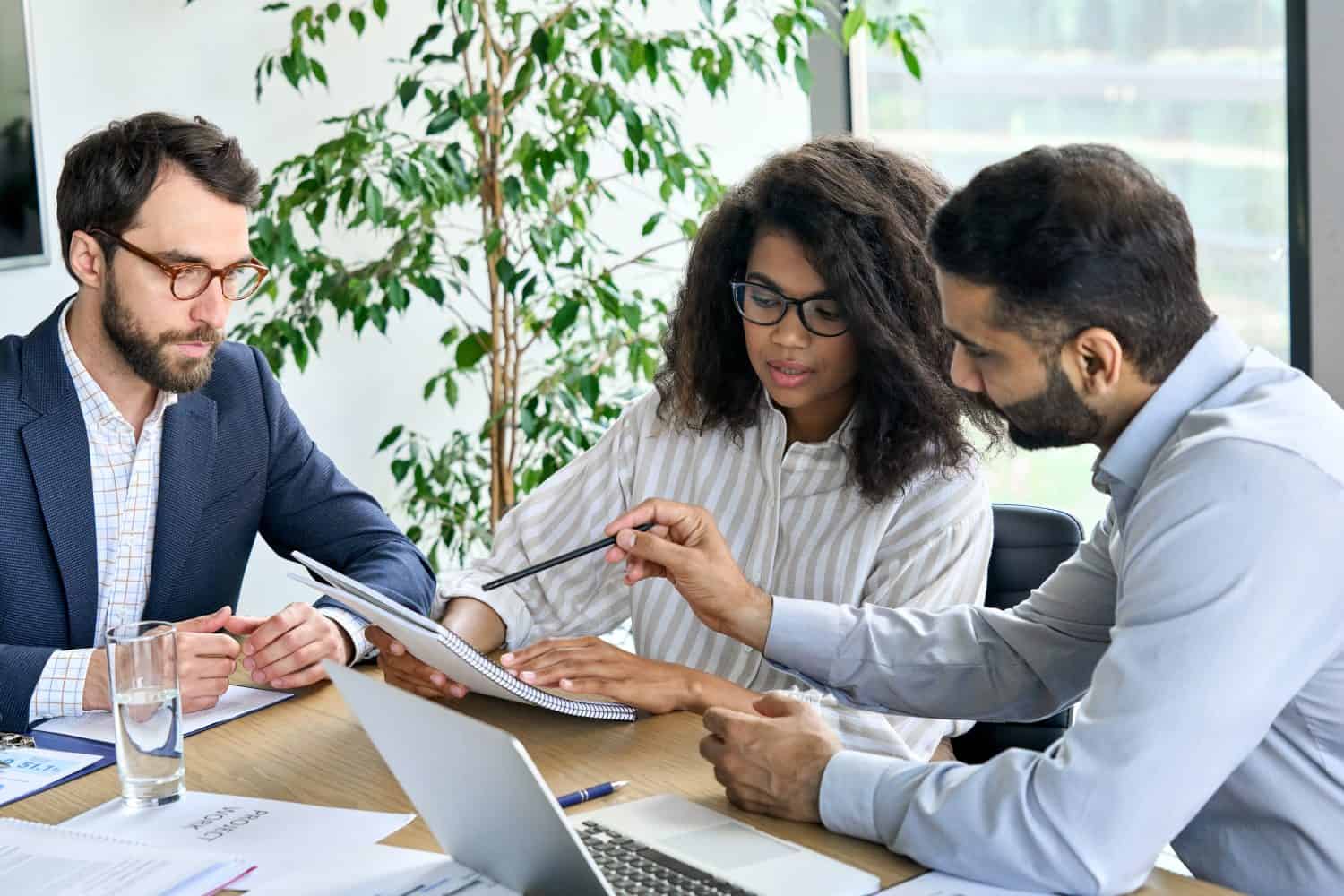
©Ground Picture/Shutterstock.com
Step 5: Visualizing the Insights
The team visualized the findings through bar charts and interaction plots to better understand the data. For example, they discovered that the preference for mixed colors was even stronger when combined with 3D graphics, indicating a synergistic effect between these two factors. Similarly, customers were more willing to accept a higher price if the product was located on a specialty shelf and paired with premium packaging.
Outcomes
Based on the insights from the conjoint analysis, FreshSense determined that the optimal product would have the following attributes:
- Color Theme: Mixed Colors
- Graphics: 3D
- Package Size: 16 bars
- Graphic Orientation: Horizontal
- Price: $3.99 (but $6.99 could work for premium placements)
- Location in Store: Specialty Shelf
This combination balanced cost-effectiveness with customer appeal, maximizing the likelihood of success in the marketplace. The conjoint analysis revealed several important findings:
- Customer Preferences Were Multifaceted: While price was a significant factor, it wasn’t the sole determinant of customer preferences. Visual appeal and shelf placement also played critical roles.
- Interactions Between Factors Mattered: The analysis highlighted that certain combinations of attributes amplified customer interest, such as the synergy between mixed colors and 3D graphics.
- Premium Segments Could Be Targeted: Although $3.99 was the preferred price point for most customers, a subset of participants showed a willingness to pay $6.99 for products with premium attributes like larger sizes and specialty shelf placement.
These insights empowered FreshSense to tailor its product strategy to different customer segments, enhancing its chances of a successful launch.
Piloting
With a clear hypothesis in hand, FreshSense developed prototypes of their optimal product and conducted a test market campaign. They launched snack bars in three different regions, each with a slightly different combination of attributes to validate the conjoint analysis findings. Over a three-month period, they tracked sales, gathered customer feedback, and monitored competitor activity. The results confirmed that the combination predicted by the conjoint analysis outperformed others, validating the effectiveness of the data-driven approach.

©Chatham172/Shutterstock.com
Conclusion
FreshSense’s journey illustrates how companies can use conjoint analysis to navigate the complex process of product development. By focusing on customer preferences and understanding the interactions between product attributes, they were able to make informed decisions that maximize their product’s appeal.
The success of this approach not only sets a new standard for future product launches at FreshSense but also demonstrates the power of data-driven decision-making in modern marketing. Conjoint analysis provided a roadmap to uncover hidden preferences, challenge assumptions, and create a product that truly resonated with customers.
The image featured at the top of this post is ©ESB Professional/Shutterstock.com.