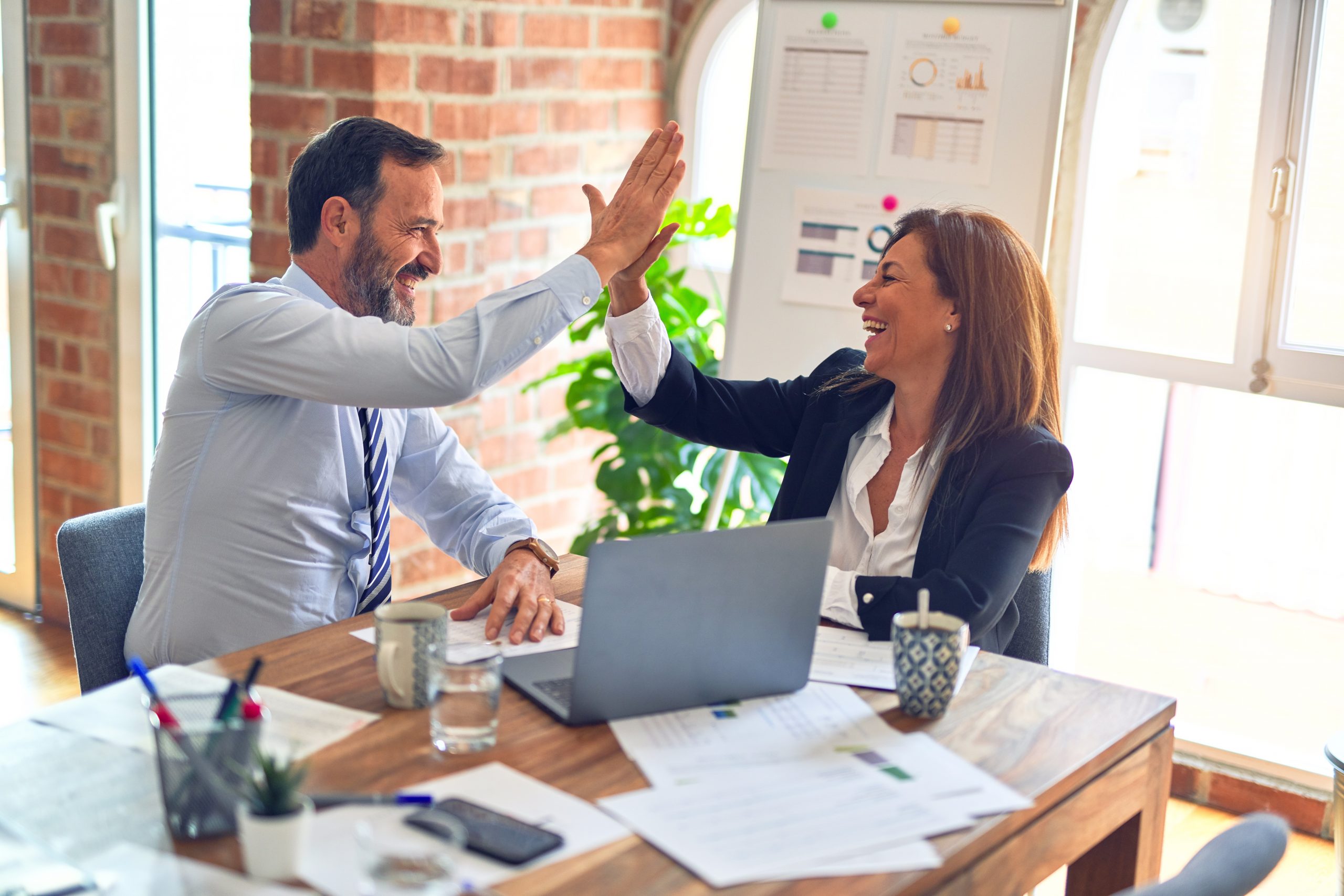
I recently read through a thread on this site’s discussion forum which started with the question “Sample Size…Why 30?” You can read it for yourself here.
It’s a question I hear a lot, except that 30 is sometimes another number. Like 2. Or 8. Or 156. Or 2068. The thread was intriguing in a number of ways, not least because it has remained active since mid-2002! Clearly there is a lot of interest in this topic.
How to sample a process is a favorite topic of mine as well, but for different reasons: in my experience it is one of the most abused topics in the Six Sigma canon. The “why 30 samples” question is, in my view, symptomatic of a root cause that involves a misunderstanding of enumerative versus analytical statistics.
(Fair warning: I am not a statistician.) Enumerative statistical techniques concentrate on understanding population data via various statistics. For example, we might want to estimate the average height of a 42 year old male in England, and to do this we could sample the population through a census. Enumerative statistics would help us understand how many people we’d need to survey from the population (and maybe something about how to choose them) to get an “accurate” estimate of the true average value at some level of statistical confidence. Techniques such as Chi Square and other tests of significance, confidence intervals, etc, are very powerful tools in the enumerative world for these types of problems. And questions like “how many samples do I need for…?” have well-defined answers.
Analytical statistics, on the other hand, concentrate on predicting the future. Which means the techniques vary considerably from those of the enumerative world. And in my view it should go without saying that the point of any Six Sigma project should be to predict the future in some way. What does Y=f(x) symbolize if not the ability to predict? Yet in my anecdotal estimation, about 95% of the Six Sigma projects I see at conferences, on the web, and in the open literature rely solely on enumerative techniques. Which is probably why there is such intense interest in questions of sample size. This is highly misguided in my opinion. Also in Dr. Deming’s, who has the following to say regarding enumerative techniques for continuous improvement in “Out of the Crisis”:
“Incidentally, Chi Square and other tests of significance, taught in some statistical courses, have no application here or anywhere.”
And later on:
“But a confidence interval has no operational meaning for prediction, hence provides no degree of belief for planning.”
(Note: I copied these quotations from an interesting exchange on this subject here.)
From an analytical statistics point of view, the relevant question to ask is not “how many samples are needed for…?” but rather “how can a representative sample be chosen such that we can predict the future behavior of the system at a useful level of accuracy?” And the crucial point is this: that second question is not a statistical question. I’ll repeat: the question of how to sample appropriately such that you can accurately predict the future behavior of the system is not a statistical question. It is entirely dependent on your knowledge of how the system behaves, and no piece of software or statistical textbook will help you answer it. You have to study the system itself, and let it be your guide.
The advice we should give in answer to the query “how many samples do I need for…?” is to turn around and ask how much knowledge of process variation/behavior we have, and then discuss how to sample that variation/behavior in such a way that we feel confident that the results will predict the future variation/behavior. In the world of analytic statistics there is no magic number that answers the question of how many samples are needed – it depends wholly on knowledge of the process.
For the same reason, I almost never recommend random sampling. To me random sampling as a strategy is a tacit admission that variation is not understood and cannot be sampled purposefully. I would rather sample in a very structured, non-random way and gain insights into process variation than sample randomly and mathematically remove the effect of “noise”. Random sampling might get rid of “noise” in the mathematical sense (and hence make enumerative calculations look better), but that noise is still there in the process. Better to sample it, study it, and deal with it than ignore it.
So when I am asked, as I often am, “how many samples do I need for this study”, my answer is always the same: “what is your sampling plan to capture the variation you are interested in seeing?” There is no single, easy answer to this analytic question – it depends completely and entirely on the specific situation.