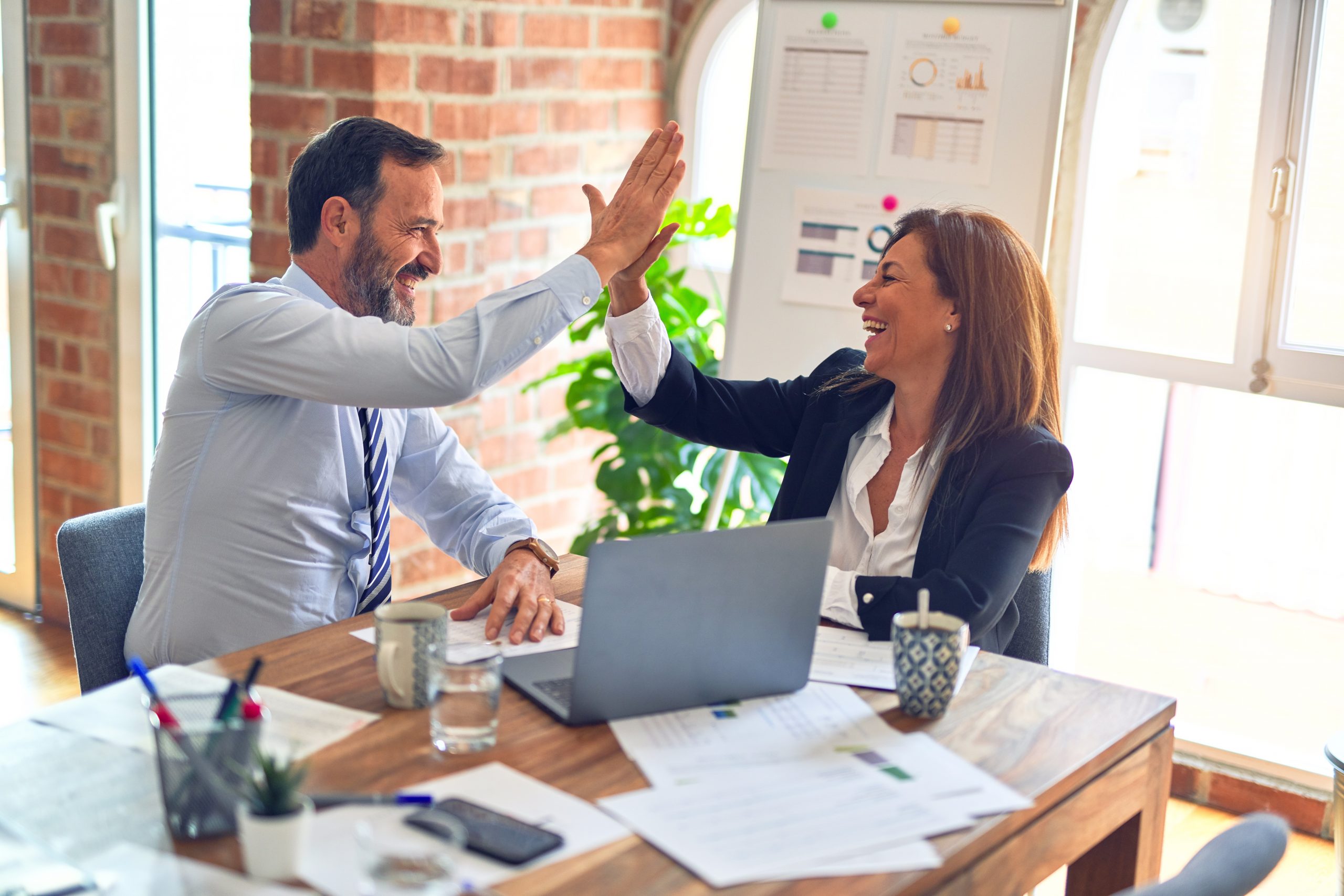
Where the idea of “process improvement” is concerned, it is now well understood that Six Sigma can play a most decisive role. Specifically, it can serve to significantly improve the process of market forecasting. For example, consider the instance where an organization attempts to deploy a certain type of ARIMA model from which to forecast future market conditions.
Without saying, the organization would naturally seek to reduce the amount of prediction error associated with the ARIMA model – prior to its deployment and implementation. To this end, a high-level Black Belt project could be formed to address the issue. Of course, the project would be structured to exploit the DMAIC approach to problem solving. In turn, such a project could serve as a “pattern” for improving other types and forms of business processes (no pun intended).
Of interest, we executed just such a project at Allied Signal in 1994 while deploying and implementing Six Sigma (at the corporate level). Clearly, the goal of this project was to refine an existing financial model. Although the forecasting model of interest was “home grown,” it was quite soundly based on the principles of polynomial regression. More specifically, this particular Six Sigma project was designed to appreciably reduce “corrupted data inputs.”
Related to the field of forecasting, we have the age-old saying “trash-in, trash-out.” It was just such wisdom that fueled our research project. In the final analysis, several sources of input error were discovered and effectively adjudicated. The net effect was a more confident set of model coefficients. In turn, the reduced “range” of prediction error provided management a more focused view of the future – but with far greater confidence.
From a purely Six Sigma point-of-view, the idea of “financial forecasting” would likely be considered an advanced topic. Certainly, it would be outside the general scope of most Black Belts. However, it would make a nice area-of-specialization for a Master Black Belt. For example, let us consider the idea of “present value.” Traditionally speaking, this concept can be defined as PV = F – R, where PV is the present value, F is the future returns (forecasted) and R is the risk. As would be expected, certain statistical methods must be employed so as to establish a confident value of F. The following is a partial listing of the procedures commonly invoked to realize the latter aim.
Simple Exponential Smoothing
Holt Winters Exponential Smoothing (Additive and Multiplicative)
Adaptive Exponential Smoothing
Linear Regression
Box Jenkins (ARIMA modeling)
Logistic Curve
Brown’s Double Exponential Smoothing
Moving Average
Brown’s Triple Exponential Smoothing
Polynomial Regression
Croston’s Intermittent Method
Probit Curve
Decomposition
Event / Promotional Modeling
Simple Regression
Gompertz Curve
Stepwise Regression with Dynamic Lagging
Holt’s Double Exponential Smoothing
Weighted Moving Average
Replenishment Statistics
Durbin – Watson
Ljung Box
Theil’s Statistic
Auto Correlation
Auto Covariance
Box Cox Method
Box Pierce Method
Thus, we have considered how Six Sigma could be used to improve the process of market forecasting. Indirectly, we have also alluded to how Six Sigma could be used as a basis for business planning. In addition, a partial listing of common tools have been prescribed.