
Key Points
- Enhanced Data Collection and Analysis – AI improves data collection in the Measure phase through IoT sensors and predictive analytics, ensuring accuracy and enabling real-time monitoring, which supports the entire DMAIC process with reliable, continuous data.
- Advanced Pattern Recognition and Insights Generation – In the Analyze phase, AI leverages machine learning to uncover hidden patterns, predict trends, and identify root causes within large datasets, helping teams pinpoint areas for improvement with greater precision.
- Automation and Control for Sustained Improvement – During the Improve and Control phases, AI-driven automation, such as predictive maintenance and process monitoring, streamline implementation and ensures long-term process stability, reducing human error and enhancing process control.
The DMAIC methodology (Define, Measure, Analyze, Improve, Control) is a structured, data-driven approach central to Six Sigma practices and quality improvement efforts across various industries. By clearly outlining steps to understand, address, and control quality issues, DMAIC drives process efficiency, product quality, and customer satisfaction. With artificial intelligence (AI) capabilities, DMAIC’s potential is significantly amplified, allowing teams to make faster, more informed decisions and leverage advanced data analysis, predictive capabilities, and automation. This article explores how AI can enhance each stage of the DMAIC process, along with practical examples to demonstrate its application.
1. Define: Establishing the Problem and Goals
The Define stage focuses on identifying the problem, understanding customer needs, and setting clear goals. AI assists this stage by collecting and analyzing extensive customer feedback, helping teams pinpoint issues more accurately and quickly.
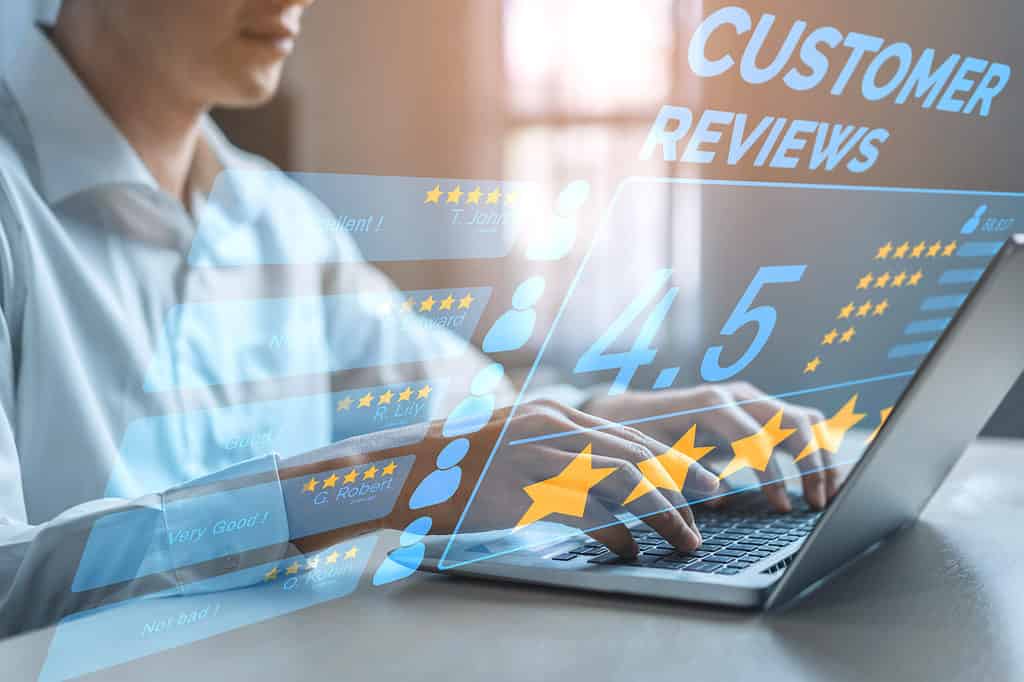
AI Applications in Define
- Customer Feedback Analysis: Natural language processing (NLP) tools powered by AI analyze large volumes of customer feedback from surveys, reviews, or social media, extracting recurring themes and sentiments. NLP identifies relevant pain points, allowing project teams to understand specific areas requiring improvement.
- Process Mapping with AI: AI-driven process mining tools can automatically map out the workflow by analyzing system logs. These tools show exactly how processes unfold, helping teams visualize bottlenecks and inefficient steps. This automated mapping helps the team define which areas of the process need improvement.
Example
A telecommunications company wants to improve customer service response times. Using an AI-driven sentiment analysis tool, the team scans thousands of customer reviews and social media comments. AI categorizes the most common complaints, helping the company clearly define areas where response times are slowest or where customer dissatisfaction is highest.
2. Measure: Gathering Reliable Data
In the Measure phase, relevant data is collected to establish baseline performance and identify key metrics for tracking progress. AI aids this phase by automating data collection and processing, which increases accuracy and decreases the time required for manual data analysis.
AI Applications in Measure
- IoT Devices for Real-Time Monitoring: AI-powered Internet of Things (IoT) sensors enable continuous data collection on machines or production lines, capturing information like temperature, pressure, or usage. AI algorithms analyze this data in real time, providing reliable, up-to-date performance metrics.
- Automated Data Quality Checks: AI can identify missing or inconsistent data in datasets, ensuring that only high-quality information is used. This capability reduces errors that could lead to faulty measurements and, ultimately, unreliable analysis.
Example
In a manufacturing plant, IoT sensors equipped with AI algorithms are installed on production line equipment. The sensors monitor variables such as temperature and vibration levels to detect signs of wear. By continuously collecting this data, the AI system helps the company measure equipment performance and establishes a baseline for improvement.
3. Analyze: Discovering Insights from Data
The Analyze phase is where teams examine data to uncover the root causes of problems. This stage is vital for understanding what drives the issues identified in the Define stage. AI enables deeper, faster analysis, often uncovering insights that traditional methods might miss.
AI Applications in Analyze
- Predictive Analytics for Root Cause Analysis: Machine learning algorithms can analyze complex datasets to identify patterns and correlations that suggest potential root causes. Predictive models enable teams to see how various factors may contribute to process inefficiencies.
- Image Recognition for Quality Analysis: AI-powered image recognition software can assess visual data (e.g., photos from production lines) to detect defects. By analyzing production output images, AI can spot quality issues early, helping identify issues that may lead to product failures.
Example
An automotive manufacturer uses AI-based predictive analytics to examine why specific vehicles have higher-than-average warranty claims. By analyzing data from various sources (e.g., materials, assembly line metrics), AI algorithms reveal that certain materials used in specific parts tend to fail more frequently. This insight helps the team focus on using higher-quality materials for those parts, addressing the root cause of the problem.
4. Improve: Implementing Solutions and Enhancing Processes
In the Improve phase, solutions are implemented to address the root causes identified in the Analyze phase. AI can be highly effective in this stage, offering automation, process simulations, and optimization tools that help teams test and implement improvements more efficiently.
AI Applications in Improve
- Simulation and Optimization: AI-driven simulation tools can model process changes to assess their impact before implementation. These tools help teams test different solutions, predict potential outcomes, and select the most effective improvement strategies.
- Automated Process Adjustments: AI-powered robotic process automation (RPA) can take over repetitive tasks or adjust processes in real time based on collected data. For example, RPA can automate quality checks and adjust machine parameters as needed to ensure consistent quality.
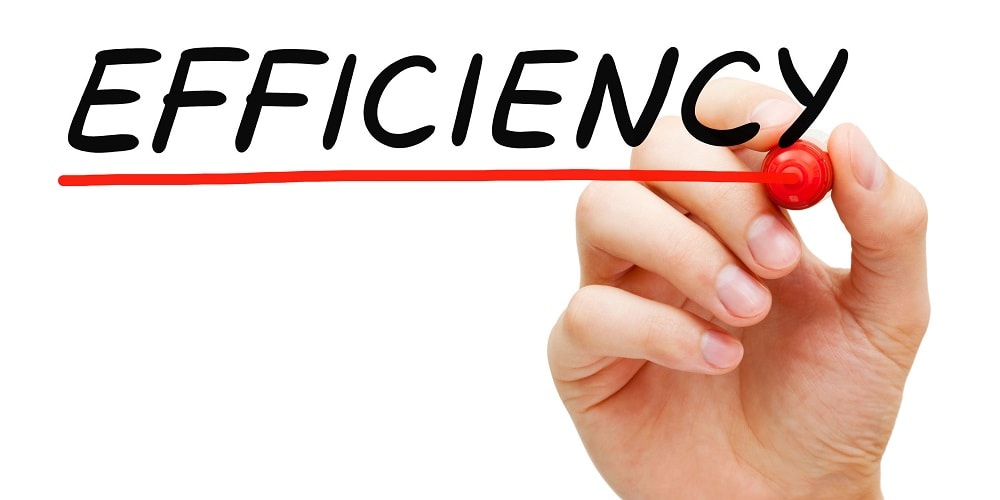
Example
In a pharmaceutical company, AI simulations are used to test process changes aimed at reducing production time for critical medication. The simulation identifies specific steps that can be adjusted to improve efficiency, helping the company implement changes with confidence. Additionally, RPA tools automate adjustments on the production line, ensuring a streamlined process with fewer interruptions.
5. Control: Ensuring Sustainable Improvements
The final phase of DMAIC, Control, focuses on maintaining improvements over the long term and ensuring that processes remain stable. AI tools assist in monitoring processes and implementing controls, minimizing the likelihood of recurrence of issues.
AI Applications in Control
- Automated Monitoring with AI Alerts: AI continuously monitors key metrics and generates alerts if performance deviates from established parameters. These alerts enable quick intervention, preventing issues from escalating or compromising the process.
- Predictive Maintenance: AI can use predictive maintenance algorithms to anticipate equipment failures, allowing for proactive repairs or maintenance. This predictive approach ensures process consistency and reduces downtime.
Example
A food processing company leverages AI for predictive maintenance on its packaging machines. By analyzing historical machine data, the AI system predicts when machines are likely to fail and alerts maintenance teams before any issues arise. This proactive approach ensures consistent production flow and prevents unplanned downtime, effectively controlling the improved process.
Similar Concepts
There is a lot of information being written on the integration of AI and the Lean Six Sigma DMAIC methodology. Here are two articles that may interest you.
- This article compares and contrasts the concepts of AI, Continuous Improvement and Big Data. You can see where the overlap occurs.
- How to adapt DMAIC training to incorporate AI concepts is the topic of this article.
Wrapping it up
Incorporating AI into the DMAIC process allows organizations to enhance every phase of this structured approach to process improvement. From defining problems with AI-driven customer sentiment analysis to measuring with IoT sensors, analyzing root causes through predictive models, implementing optimized solutions with RPA, and controlling improvements via automated monitoring, AI brings a level of precision, speed, and depth that traditional methods cannot match. As AI technology continues to evolve, its applications within DMAIC will only become more sophisticated, offering more valuable insights, greater efficiency, and stronger results for businesses seeking to improve their processes and deliver higher-quality products or services.
With AI at each DMAIC stage, organizations can drive continuous improvement, responding quickly to changing conditions and ensuring long-term success in an increasingly competitive market. AI doesn’t just streamline DMAIC; it transforms it, making process improvement more effective and adaptive than ever before.