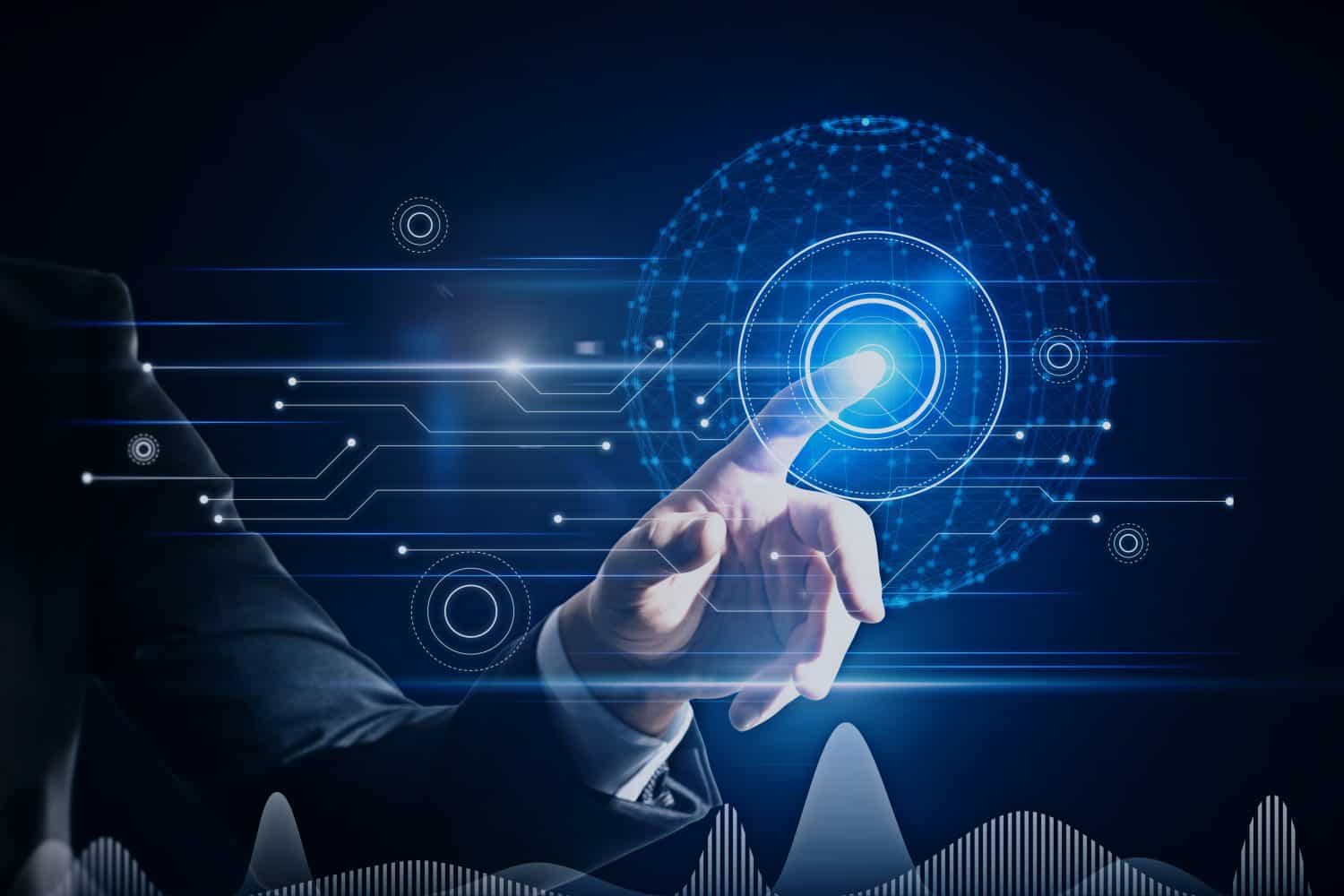
© Golden Dayz/Shutterstock.com
Key Points
- AI is primarily focused on enabling machines to perform tasks that typically require human intelligence, such as decision-making, language understanding, and pattern recognition. Continuous improvement emphasizes incremental enhancements in processes, products, or services over time. Big data is centered around the collection, storage, and analysis of vast volumes of data to uncover patterns and insights.
- AI uses algorithms and models (like machine learning or neural networks) to learn from data and make predictions or decisions. Continuous improvement relies on methodologies like Lean or Six Sigma to systematically identify inefficiencies and enhance operations. Big data involves statistical analysis and data processing techniques to analyze large datasets and derive insights, often serving as the foundation for AI applications.
- AI aims for automation and intelligent decision-making, often reducing human intervention. Continuous improvement seeks gradual, sustained enhancements in efficiency and quality. Big data’s outcome is mainly to derive actionable insights from data, which can then inform AI models or drive continuous improvement initiatives.
- AI solutions can operate at various scales, from small-scale personalized recommendations to large-scale automation. Continuous improvement tends to focus on incremental, localized changes that can aggregate over time. Big data operates at the largest scale, handling immense datasets that require significant computational power for processing and analysis.

AI (Artificial Intelligence) involves creating systems and algorithms capable of mimicking human intelligence to perform tasks like decision-making, problem-solving, and pattern recognition. It leverages techniques such as machine learning and neural networks to improve through experience. Continuous improvement focuses on systematically enhancing processes, products, or services through incremental changes, using methods like Lean or Six Sigma to eliminate inefficiencies and boost quality over time. Big data refers to the collection, processing, and analysis of vast datasets, often too large for traditional methods, to derive valuable insights, patterns, and trends that support data-driven decisions and optimize performance.
Here is a table comparing the elements of Artificial Intelligence (AI), Continuous Improvement (CI), and Big Data:
Element | Artificial Intelligence (AI) | Continuous Improvement (CI) | Big Data |
---|---|---|---|
Definition | AI is the development of computer systems that can perform tasks typically requiring human intelligence, such as decision-making, problem-solving, and learning. | CI is the ongoing effort to enhance processes, products, or services incrementally over time. | Big Data refers to the large volume of data, structured or unstructured, that can be analyzed for patterns, trends, and insights. |
Objective | To simulate human intelligence for decision-making, automation, and prediction. | To continuously improve processes, reduce inefficiencies, and enhance quality over time. | To extract meaningful insights from large datasets to support data-driven decision-making. |
Scope | Wide-ranging: spans multiple industries (healthcare, finance, manufacturing) and tasks (automation, natural language processing, robotics). | Typically focused on improving internal processes within organizations (manufacturing, services, etc.). | Primarily used for decision-making and forecasting in various sectors (business intelligence, healthcare, marketing). |
Key Techniques | Machine learning, deep learning, neural networks, robotics, natural language processing. | Lean, Six Sigma, Kaizen, Total Quality Management (TQM), Plan-Do-Check-Act (PDCA) cycles. | Data mining, machine learning, predictive analytics, cloud computing, and real-time data processing. |
Input Requirements | Large amounts of data for training algorithms, computational resources, and software systems. | Feedback, process performance metrics, customer or employee suggestions, and continuous tracking of process data. | Vast amounts of raw data from various sources (social media, IoT, sensors, business transactions). |
Output | Automation tools, intelligent agents (e.g., virtual assistants), predictive models, enhanced decision-making systems. | Process improvements, cost reductions, enhanced quality, operational efficiency, employee engagement. | Actionable insights, predictive trends, data-driven business strategies, decision-support tools. |
Strengths | Ability to automate complex tasks, perform high-speed computations, and make predictions with accuracy. | Provides sustainable, long-term process improvements and builds a culture of innovation. | Handles vast amounts of data, identifies hidden patterns, and enhances decision-making with data-driven insights. |
Challenges | Ethical concerns (bias, transparency), data quality, high costs of implementation, and dependency on data availability. | Difficulty in maintaining momentum, resistance to change, time-consuming, and requires strong leadership support. | Privacy concerns, data security, challenges in processing unstructured data, and high computational resource needs. |
Human Involvement | Low to moderate: humans typically oversee, train, and adjust AI systems but the systems can operate autonomously. | High: human involvement is critical in identifying areas for improvement, implementing solutions, and sustaining momentum. | Variable: humans are involved in interpreting results, managing systems, and making final decisions. |
Applications | Autonomous vehicles, healthcare diagnostics, fraud detection, natural language processing, recommendation engines. | Manufacturing (quality control), customer service (process efficiency), project management, product development. | Marketing analytics, risk management, healthcare, business intelligence, predictive maintenance, IoT-based analysis. |
Challenges | Ethical concerns (bias, transparency), data quality, high costs of implementation, and dependency on data availability. | Difficulty in maintaining momentum, resistance to change, time-consuming, and requires strong leadership support. | Privacy concerns, data security, challenges in processing unstructured data, and high computational resource needs. |
Human Involvement | Low to moderate: humans typically oversee, train, and adjust AI systems but the systems can operate autonomously. | High: human involvement is critical in identifying areas for improvement, implementing solutions, and sustaining momentum. | Variable: humans are involved in interpreting results, managing systems, and making final decisions. |
Applications | Autonomous vehicles, healthcare diagnostics, fraud detection, natural language processing, recommendation engines. | Manufacturing (quality control), customer service (process efficiency), project management, product development. | Marketing analytics, risk management, healthcare, business intelligence, predictive maintenance, IoT-based analysis. |
Technological Dependency | Heavily reliant on algorithms, data availability, and computing infrastructure (hardware and software). | Dependent on management tools (dashboards, KPIs), organizational culture, and structured improvement frameworks. | Relies on data storage, high-speed processing, cloud computing, and advanced analytics tools. |
Evolutionary Trend | Moving towards more human-like decision-making, higher autonomy, and general intelligence capabilities. | Continues to evolve with new methodologies (Agile, Lean), tools, and technologies, adapting to modern business needs. | Rapid expansion with increasing sources of data (IoT, social media), advanced real-time analytics, and increased use of AI. |
Here is a table contrasting the elements of Artificial Intelligence (AI), Continuous Improvement (CI), and Big Data:
Concept | Artificial Intelligence (AI) | Continuous Improvement (CI) | Big Data |
---|---|---|---|
Definition | AI refers to the simulation of human intelligence in machines designed to think, learn, and adapt. | CI is the ongoing process of improving products, services, or processes through incremental improvements. | Big Data refers to extremely large datasets that are processed to reveal patterns, trends, and insights. |
Core Objective | To automate tasks, make decisions, and mimic cognitive functions. | To enhance efficiency, quality, and effectiveness through gradual, ongoing changes. | To derive meaningful insights and trends from vast amounts of data. |
Key Techniques | Machine learning, neural networks, robotics, natural language processing, automation. | Lean, Six Sigma, Kaizen, Plan-Do-Check-Act (PDCA) cycles. | Data mining, predictive analytics, machine learning, data visualization. |
Primary Inputs | Data (structured and unstructured), algorithms, models, and computational power. | Employee suggestions, performance metrics, process data, feedback. | Massive datasets, including structured, semi-structured, and unstructured data. |
Output | Intelligent systems (e.g., chatbots, autonomous vehicles, recommendation engines). | Improved processes, enhanced product quality, reduced waste. | Insights, trends, predictive models, and data-driven decision support. |
Strengths | Automation, high-speed processing, complex problem-solving, learning from data. | Incremental and sustainable changes, fosters a culture of continuous learning. | Ability to process vast amounts of data for actionable insights and trends. |
Challenges | Ethical issues (bias, privacy), data quality concerns, high computational cost. | Resistance to change, maintaining momentum, scope creep in improvements. | Data privacy, storage, real-time processing, managing unstructured data. |
Primary Applications | Autonomous systems, healthcare (diagnosis), finance (fraud detection), customer service. | Manufacturing, operations management, customer service, quality management systems. | Marketing, business intelligence, healthcare, finance, IoT (Internet of Things). |
Technological Dependency | Requires advanced algorithms, computing infrastructure, and access to large amounts of data. | Relies on organizational tools (dashboards, metrics), employee engagement, and data tracking. | Dependent on high-speed data processing, storage systems, and analytics platforms. |
Human Involvement | Minimal in execution (supervisory role in system training and monitoring). | High, with employees driving the improvement process through feedback and analysis. | Variable, often involves human oversight for interpreting data and making decisions. |
Evolutionary Path | Moving toward greater autonomy, more sophisticated learning, and better human-like reasoning. | Evolving with new methodologies (Agile, Lean) and technologies to facilitate improvement. | Expanding rapidly with more sources of data (IoT, social media) and advanced analytics capabilities. |
Similar Concepts
In the world of business, three very common terms we hear about are Artificial Intelligence (AI), Continuous Improvement (CI) and Big Data. Here are a couple of articles to help complement you understanding of these concepts:
- This first article discusses what CI needs to consider as organizations embrace AI.
- There are some ethical considerations when utilizing AI. This article discusses those.
Final Thoughts
AI, continuous improvement, and big data differ in focus but often complement each other. AI involves creating systems that perform tasks requiring human intelligence, such as learning and decision-making. Continuous improvement is a methodology focused on making gradual, systematic enhancements in processes or products over time. Big data deals with collecting, processing, and analyzing large datasets to uncover insights and patterns. AI uses big data to train models and improve decision-making, while continuous improvement can leverage both AI and big data to optimize performance. In contrast, continuous improvement emphasizes incremental changes, while AI seeks intelligent automation.
The image featured at the top of this post is ©Golden Dayz/Shutterstock.com.