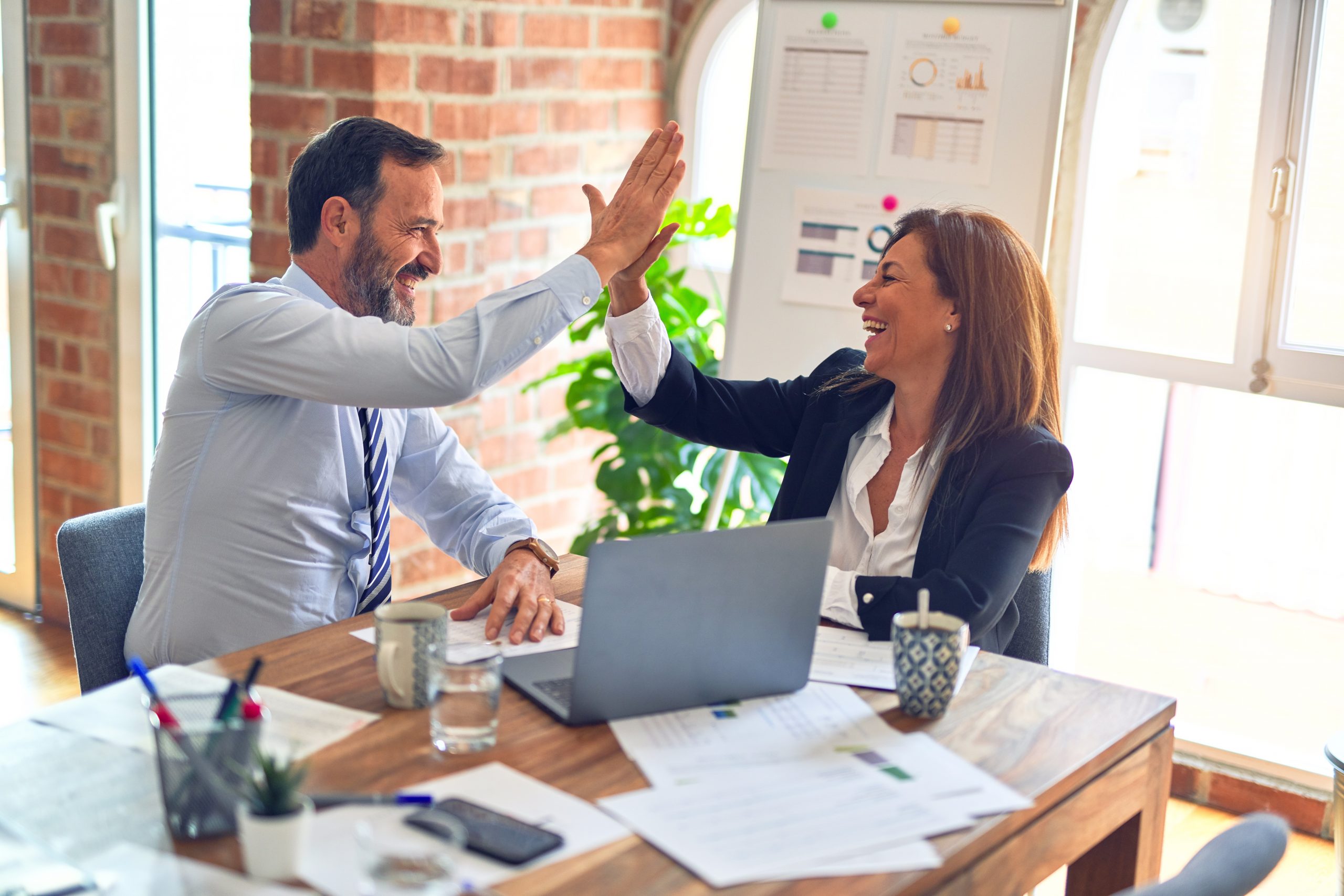
As the saying goes, if all you have is a hammer in your toolbox, everything looks like a nail. But if you had a torque wrench or a power drill with a box of bits, you are likely to be much more effective.
Design of experiments (DOE) is one of those specialized and sophisticated tools you should have in your toolbox. It is a technique to optimize any process or product better, faster and cheaper than other optimization methods, including A/B testing (also known as OFAT or one-factor-at-a-time) and “expert” guessing.
This interview starts at a high level, discussing what DOE is and how it is used, then dives into several examples (e.g., government, technology, human resources), and ends with a hands-on demonstration using software to analyze and find the critical factors in examples in healthcare (blood analysis) and a functional area (sales force).
Quick browse:
- What is DOE? (3:01)
- Why is DOE so important? (6:29)
- What makes DOE different from other testing techniques like one factor at a time, educated guesses or A/B testing? (11:26)
- Is DOE only applicable to complex and expensive systems, such as manufacturing environments or product design, or is it valuable for somebody like me who can change a webpage myself and the cost is basically zero to make all those changes? (16:33)
- Can you give me an example from a functional area at a Fortune 100 company, such as human resources or marketing, where design of experiments can be applied? (25:21)
- Case study 1: Aligning a sales force after two companies merge (33:18)
- Case study 2: Blood analysis (55:00)
Resources Mentioned in This Interview
- How Fleet Bank Fought Employee Flight, Harvard Business Review.
- DOE Pro software
About Mark Kiemele
Dr. Mark Kiemele is president and co-founder of Air Academy Associates. Dr. Kiemele has more than thirty years of teaching and consulting experience, and has trained, consulted, and mentored more than twenty-five thousand people from more than twenty countries, including companies such as Sony, Microsoft, GE, Raytheon, Lockheed Martin, and Samsung.
Dr. Kiemele has also co-authored or edited five books, including Design for Six Sigma: The Tool Guide for Practitioners and Reversing the Culture of Waste: 50 Best Practices for Achieving Process Excellence (see interview).
Follow Air Academy on Twitter
Follow iSixSigma on Twitter
Mark Kiemele Interview Raw (Non-Edited) Transcript
Mark Kiemele Interview Transcript in PDF Format (Right-click to Save As…) [View in Google Docs]
Watch the full video at:
https://www.isixsigma.com/tools-templates/design-of-experiments-doe/mark-kiemele-interview/
Michael Cyger: Hey everyone. My name is Michael Cyger, and I’m the founder and publisher of iSixSigma.com – the largest community of Lean and Six Sigma professionals in the world and the resource for learning to drive breakthrough improvement.
Here is what we do here. We bring on successful Lean and Six Sigma business leaders, we learn from their experiences, they share their strategies and tactics, and then, when you have a success to share, you can come on the show and give back, as today’s guest is going to do.
Anyone that visits my garage knows that I love tools. My father was an automotive mechanic, so I have every screwdriver of every size, every hammer type, every wrench. You name it, I have it in my toolbox. So, whenever a job comes my way, I know I have the right tools to get it done. Just like I would not use a small slotted screwdriver to take out a Phillips screw, I cringe at the idea of doing one factor at a time testing, or best guess and then test, to figure out the best solution to a problem that I am facing.
The same is true in business, yet it happens every day. If I read the latest technology startup blogs, they actively promote one factor at a time testing or AB testing. There has got to be a better way; and there is. Joining me today to help us understand DOE, or design of experiments, is Dr. Mark Kiemele, president and co-founder of Air Academy Associates. Mark has more than thirty years of teaching and consulting experience, and has trained, consulted, and mentored more than twenty-five thousand people from more than twenty countries, including people at Sony, Microsoft, GE, Raytheon, Lockheed Martin, and Samsung. He has also co-authored or edited 5+ books, including one that I was honored to have helped publish through iSixSigma’s parent company, CTQ Media, Design for Six Sigma: The Tool Guide for Practitioners. And we are going to talk a little bit about this book today.
Mark, welcome to the show.
Mark Kiemele: Thank you very much, Mike. It’s a real pleasure to be with you.
Michael: We are going to tackle the topic of DOE in two parts, Mark, as we discussed. The first part will be a conversation between you and me about design of experiment, so we can understand the concept and put everything into context. So, somebody who has never done design of experiments, but has heard of AB Testing, can understand why they are related, how they are different, and then we will move into sort of a phase two, which is a hands-on portion and we will run a couple of examples – one transactional, one more technical – so that the audience can see exactly how design of experiments are run. Sound good, Mark?
Mark: Sounds great, Mike.
Michael: All right. So, let’s start with an easy question, Mark. What is design of experiments?
Mark: Mike, let me give you my elevator speech for DOE, or design of experiments. DOE is the best data collection strategy that is out there today when our goal is to investigate relationships between inputs and outputs of a process. Now, let me explain inputs and outputs of a process. A process that most of us are very familiar with, Mike, is driving an automobile, or owning an automobile. And one of the performance measures, or responses, that we might be interested is gas mileage – miles per gallon. That is how we typically measure gas mileage. Now, there are other measures of performance or responses, like: “How long does is take to go from zero to sixty miles per hour?” But let me just talk about miles per gallon a second. That would be considered an output of the process – of this automobile process, if we want to call it. It is a performance measure. Sometimes we call it a response variable. It is also an output. Now, we can surmise what various inputs might be to that, that affect that output. Well, one might be, and our experience level with an automobile is going to tell us that, and one might be tire pressure. Maybe tire pressure would be the factor. Now, if we tested it at two different levels, like 25 psi versus 35 psi, tire pressure would be the factor, and the two levels at which we test it would be, say, 25 and 35 psi. Another factor that we could surmise might affect gas mileage is the type of fuel that we are using. Is it 85 octane? 91 octane? So, fuel type might be another factor. The two settings, or the two levels, that we might want to test fuel type at are maybe – I know, in Colorado, we have 85 octane. You may not have that in Washington, but we may go to a low setting, like 85 octane and a high setting at 91. So that gives the followers here a little bit of an idea of what a factor is or an input. Sometimes we call these factors inputs and outputs. But by and large, DOE is the best way to collect data when you want to find relationships between inputs and outputs, Mike.
Michael: Okay, that makes sense. And if I was an automobile manufacturer and I realized how important that miles-per-gallon rating was of the cars I was manufacturing with gas prices nearing five dollars a gallon here, in Washington State, I know that that is likely one of the top factors influencing whether somebody buys their car or buys some other car, so I need to optimize my miles per gallon on that car and I want to produce the best miles per gallon possible. So, what are the factors that go in there? Clearly I need to have something that is energy efficient, but there are a lot of factors that go in. Besides just the engine, it is the tire pressure, and it is exactly what you said earlier. And so that is what DOE allows you to do. Optimize an output variable based on a bunch of different input factors.
Mark: Exactly.
Michael: Okay. And so, why is DOE is so important to regular business people or to other people? I understand how it works in a manufacturing environment, and that is where I think DOE has the most classic examples. Why is it important to regular people or regular business users?
Mark: Well, it is important to everyone, whether they are a business leader, or practitioner, or whatever, because, first of all, it is going to save time and money, and time and money are our great resources for us. So, if you can save time and money, that is a very important part of it. Test and evaluation is in almost every organization, Mike, does testing. You test something. Whether it is a product or a service – whatever it is – you are testing a lot of the time. And a lot of folks do not know that DOE is the connectivity between test and evaluation. How you test, how you collect the data, what combinations you test of the different factors of the different levels is going to either make it easy or make it hard in the evaluation stage. So, DOE is the connecting link between test and evaluation. But besides time and money, a big thing is knowledge. Knowledge is critical. DOE is going to give the practitioner and the leader the knowledge that they need. What is important? Can you separate out? For gas mileage, is it tire pressure that is more important than the fuel type? How do you prioritize? How do you rank order the factors that could affect? Which ones are significant? Which ones are not? Knowing that kind of knowledge, of course, leads downstream to cost and time savings as well. But interaction effects. DOE is designed to get that interaction effects. Interaction effects are tough stuff. Tolstoy once said, and let me quote Tolstoy on this. He said, “The combination of events is beyond the comprehension of man.” Well, guess what? Combination of events is called, in DOE, interaction effects.
Michael: Okay.
Mark: I would like to extend Tolstoy’s quote to the combination of events is not beyond the comprehension of man using DOE. So, DOE allows us to get the requisite knowledge we need to make good decisions. I mean that is basically what it is. It is changing ‘I think’ to ‘I know.’
Michael: Yeah.
Mark: And the more knowledge we have, the better decisions we will make. And the better decisions we make, the more money and time we will save.
Michael: Hey Mark, in our pre-interview conversation, we discussed interaction effects on DOE, which we are going to go into more later on. So, if people are like: “Well, I do not understand interaction effects,” taking it to the off statistical world example – the combination effects from Tolstoy – you used a real world example of weather issues that happen as a result of combination effects that helped me understand interaction effects. Can you repeat that?
Mark: Well, I am not sure I used the weather one, but weather is an important factor; and that is something we typically cannot control. It is a noise variable. But weather interacts with a lot of factors. For example, in the gas mileage, you take a look at miles per gallon. The effect of weather combined with altitude, for example. I live at six thousand feet. Yesterday we had what one might call a blizzard. So, the weather, if I were driving yesterday, even at this altitude, the change in weather coupled with the altitude could give me a gross change in the gas mileage that I am expecting. Basically, if one factor, like weather. Altitude is another factor. When the effect of one factor is exacerbated by the change in another factor that is when we have an interaction effect.
Michael: Got it, okay. So, in my intro, I mentioned that a lot of companies in the startup world were focused on AB testing. There are a whole slew of service providers online, like Optimizely.com or VisualWebsiteOptimizer.com; even Google Analytics allows companies to do AB testing. So, if I have a signup page on iSixSigma.com, for example, I can test how many people come to the page and click and sign up with a green button versus an orange button on the side. So that is AB testing, or one factor at a time testing. I change one factor and I measure it. What makes DOE different from these other testing techniques like one factor at a time, or educated guesses, or AB testing?
Mark: That is a great question, Mike, because that is the heart and soul of DOE. Why would I want to use a DOE when I was taught, in high school, to do one factor at a time testing? When my chemistry teacher says, “When you want to see the effect of temperature on this experiment, you got to hold everything else the same and you just change that one thing, and then you will see what effect it has on your yield or whatever you are measuring for a response or an output.” How misinformed are we, because we are teaching people at the very young stages of their life to do this AB, or one factor at a time, testing? There is different criteria or, what I should say, attributes of DOE, like replication, randomization, which we will talk about a little bit later when we get into the examples, but the one characteristic of DOE that distinguishes it from everything else is the concept of orthogonality. Orthogonality allows, and that is how we distinguish DOE. A DOE is a testing technique that has an orthogonal design or nearly orthogonal design. Now, what orthogonality buys is the ability to evaluate these factors, their effects, and the interactions independently from one another. That keyword is independent evaluation. And what does independent evaluation buy us? Well, independent evaluation buys us the ability to get to root cause. That is cause and effect relationships. And cause and effect relationships – root cause analysis today is tough stuff, Mike. People make light of it. It is hard because of those doggone combination effects.
Michael: Right.
Mark: Those interaction effects. So, if you have orthogonal designs, orthogonality buys you the ability to evaluate the effects independently. Independence allows you to buy the ability to get to root cause analysis. And of course, if you can find root causes of problems, guess what? Your decision-making is enhanced and, of course, the financial aspect will also be enhanced. So that is the orthogonality aspect.
Michael: Okay. And so, that all makes sense to me, Mark. I think most of the people that watch this show are educated like you and I are, and they can understand that you are saying orthogonality is this concept that allows you to evaluate the factors independently. So you do not see the bias. You do not have results that are confounded; is another word that means the results you are seeing are actually affected by something else that you are not anticipating. So that makes sense, but then I just go back to my real world example, where I have got a signup page on iSixSigma.com, and the output variable from what you were telling me earlier was I want people to actually sign up. Maybe it is put in an email address and click the signup button. Right? And so, AB testing would tell me, or my fifth grade science teacher would tell me, “Start off with an orange button. Change it to a green button. See how much that affects it. Then go from whichever one is better. That is now your base. Now change it from a green button with a sans serif font to a serif font, and so it feels more like a typewriter. Maybe that will affect people.” Every industry and every group of people is different, and you might say that the fortune one hundred, which come to visit iSixSigma.com to learn about concepts like DOE would be more attuned to a font that looks like a typewriter, let’s say, and so they are going to get a higher click-through rate on that. Will DOE allow me to say: “My output is signups on this page; now I want to look at all the factors that are involved,” and just solve it once, so I am not doing AB testing for the rest of my life?
Mark: Absolutely, Mike. That is the beauty and power of DOE. You can test multiple things simultaneously and still evaluate the effects independently. Orthogonality means balance in the design. That is – get back to your font and you color thing. You now home in on their orange or green, and then you start changing the fonts. There is a way to test those simultaneously so that you have balance in the design. And that balance will allow you to get that independent evaluation. We will talk a little bit about that in one of the examples that we do.
Michael: Great. All right.
Mark: Because all of our designs, all of our DOEs, all of our testing strategies are going to orthogonal or nearly orthogonal.
Michael: Okay. So, my next question was going to be when we talk about designing a car for optimum miles per gallon or doing a design of experiments on a missile system, for example. My question is: Is DOE only applicable to complex and expensive systems, such as manufacturing environments or product design, or is it valuable for somebody like me who can change a webpage myself and the cost is basically zero to make all those changes?
Mark: That is one of the myths about DOE, Mike, and you kind of hit on it there, saying, “Well, it is only for complex situations, complex products, services, and that.” DOE can be used in any area of life. For example, I go for shoulder therapy to my physical therapist. And I ask him. I said, “Okay, I have only got time today, in a day, to do four or five exercises, and you got to tell me what those four or five best ones are. And not only that. You got to tell me the order in which I am supposed to do them because obviously order might make a difference too.” And this young man – good young guy – looked at me and said, “Man, this guy must be out of his mind.” And I said, “We can help you with this. Just look out in the waiting room how many patients you have. Are you doing any testing? Are you recording any data? And we can show you how to collect that data to help you.” So, I do not care, Mike, if it is in the orthopedic therapy room, whether it is in finance, or whether it is in education. And education, I mean I remember of the days of the Air Force Academy where we would sit around the table and some people would say, “Well, we got to quiz daily. We got to give daily quizzing. That helps the learning.” And somebody else will say, “That does not do anything. Daily quizzing does not help anything.” How are you going to answer that question? You got to test it. Okay, same thing with computer-based learning. You have got those advocates of it. You have got the advocates that do not espouse to computer-based learning. You got to test it. I do not care, Mike, if it is in education, if it is finance, or if it sales and marketing. We will get into a little bit of that later, but if your budget for sales or for marketing is a certain level, how do you break that budget up into the various categories where you can spend your advertising dollars? What is the optimal mix? It can be used for anything. It can be used for any time you want to find what factors are the most important that effect some response or some value variable that is an output. This is the way to do it.
Michael: Yeah, all right.
Mark: It does not have to be hard. You can do it for one factor, two factors, and multiple factors. DOE is sometimes called multivariate testing because the beauty of it is you can test many things simultaneously.
Michael: Yeah. And so, in Part Two of this interview, Mark, we are going to go over an example from health care, which is really technical, and then we are going to go over an example from a transactional environment, as you just alluded to, in sales to show exactly how those design of experiments are completed. And we talked briefly about my application in the high-tech world. Companies that are growing completely online like an Amazon.com, or an Ebay.com, or a Google.com. How did they do their testing? How did they sell more? How did they make sure that the ads that are placed at the top are being clicked at to the highest degree? That can be done with design of experiments, as we just discussed. Can you give me a DOE example from government use?
Mark: Well, I could give you hundreds of those, Mike, that involves ships. Large systems like ship, subs, aircraft, ground vehicles, and also systems that are now being designed to be prevent successful cyber attacks. I could give you those, but the one I want to give you is one that I think we can all relate to, and that is AIDS. The spread of AIDS has been a big problem and the State Department, years ago, asked us at the Air Force Academy to investigate the key factors that influenced many, many response variables, one of which is the propagation of AIDS. And so it is a big problem. Lots of factors that are involved. And they had a model that was built by scientists at Los Alamos National Labs and also the Miriam Research Center at University of Illinois. And they had like 360 differential equations. They were deterministic differential equations that had (Unclear 21:08), and somehow we had to make sense of all of that. So, we got it down to one hundred and thirty-four factors. About one hundred and thirty factors that we wanted to investigate. Well, what kind of design do you have for evaluating one hundred and thirty-four factors simultaneously? Well, today it would be easy, because we have the software – the hardware to do this. But back in those days, Mike, we did not have that, so we had to generate a one hundred and thirty-six design, which is called a Plackett-Burman design. It does not matter what you call you it, but it is one hundred and thirty-six test cases or runs, as we would call them, and we did that and we were able to flesh out the most important factors. That was a screening design, where you screen out or separate out the vital few from the trivial many. So that was one of the largest designed experiments I was involved in some years ago. Now, with design of new automobiles and things like that, you are dealing with lots of factors like that again. Simulators have lots of factors. And that was essentially what this was. It was a simulator, but they were differential equations. Very complex stuff, and you had to fair it out – the most important factors. And it was interesting that the State Department folks that heard the last briefing and got the reports that it is really interesting now that we can prioritize these factors and we can now start looking at what we have to act on. Where do we spend the money now to, in fact, reduce this propagation of AIDS?
Michael: Got you. So that sounds like a great example. It is something that is very complex from a socio-economic perspective. One hundred and thirty factors. That is the kind of thing that would boggle my mind to try and solve. How do you solve AIDS? But you used a bunch of experts, you narrowed it down to one hundred and thirty factors, you then put it into a design of experiments using a specific design that you mentioned, and you screened it to find which factors were actually important to the output and which factors actually were not that important. Maybe there was some personal bias from a PhD who is an expert in some area of age or society, and you were actually able to use the data to then find the truths. And what happened from that study, Mark? Is there something that was measurable that the government was able to use in order to affect the spread of AIDS?
Mark: Absolutely. I cannot remember the top seven, but once we got the top seven or so, we were able to then build modeling designs, Mike, that would allow us to get at the interaction effects, because that is where the keys are. They keys are in those doggone combination effects or interaction effects that you have. And by golly, we could then, in fact, find some interaction effects. And that led the government to say, “Oh, well, this factor by itself is not as important as the other factor, but when you combine them together, their combined effect is much greater than each one individually.” So that allows them to home in on the factors, and then, of course, like you said, the socio-economic impact is huge and you have got to zero in on what can you do from a socio-economic point of view to minimize the impact of those factors. That is the real hard part. The DOE, Mike, is not hard. That is the point. The point is, is we think the easy stuff is hard and the hard stuff is easy. The hard stuff is once you know the factors; now what are you going to do about it? Where are you going to put your money? How are you going to impact those factors?
Michael: Right. Definitely. But somebody may be watching this interview right now, Mark, and say, “Well, I have worked in health care and I know that AIDS is a statistical issue, and one hundred and thirty factors. I just work in human resources, or I just work in marketing at my company. Like you are talking about stuff that is rocket science. I am just a human resource manager.” Can you give me an example from a functional area at a Fortune 100 company, such as human resources or marketing, where design of experiments can be applied?
Mark: Absolutely. The one that comes to mind, Mike, comes from Boston Fleet Bank, which is now part of Bank of America. This was done, I think, in 2004, maybe eight or nine years ago. Done by a young lady and her team in the HR department at Boston Fleet Bank. Their problem was turnover. Turnover was the response. That is the variable that was creating problems. When you have high turnover rates that is expensive. It costs money to bring people in – to hire people and to train them up to speed. And worse than that was sometimes an unmeasurable things like the high turnover rate was in areas where these folks were interfacing with the customers. Okay? And that is tough stuff.
Michael: Right.
Mark: I know DOE, but I am not an HR person, but these guys know HR. So, these guys said, “What are the factors that could be contributing to these high turnover rates?” Now, I probably would not have come up with stuff like this, but time since last promotion. Educational history. I might have gone to the educational history thing. Job stability history. What is the local unemployment rate at the time somebody left? What is the local employment alternative? What is the company’s market share? Then you have got the company’s policies, like what is the lateral upward mobility climate like? The layoff climate. There are all kinds of factors. All of those things are factors. Well, guess what? They investigated 16 or 17 factors, and they narrowed them down to two or three that were really critical that allowed them to change their policies on supervision. Supervisor stability. That is not their mental stability. That is how long they were engraved. That turned out to be a very, very important factor. So, it changed their policy that supervisors would stay in their positions longer. They would have more training for their supervisors. And one of the other factors that was important- statistically significant anyway – was how they recruited these people. Did they get them through an agency or were they hired based on internal recommendations? And the internal recommendations folks tended to stay longer. So those factors started coming out. And the beauty of the model that they developed was they got data. Every time there was somebody who would leave the company they got data, so they knew what the factor values were at the time somebody left, and they could affiliate that with that particular individual, and they rolled that back into their model. Continuously updating their model so they could predict and find what the factors, if there was any change in the factors, that are really affecting the output.
Michael: Yeah.
Mark: So, actually that example, Mike, was so impressive that it was written up in Harvard Business Review. So people can go to HBR and they can read about that particular DOE.
Michael: Excellent. And I will put a link, if I can find it, to that HBR. And if people want to buy it, if it has a cost, or link. And if I forget, somebody from the audience, post a comment and ask me to remember to post a link to that and I will post a link. But that is an enormous cost – employee turnover. Having worked at a large corporation, I know how much of my time goes into training somebody that I hire as well as the entire company. I think one time we quantified it at GE and it was at least ten thousand dollars that goes into bringing on a new employee. And if you are at a startup company that maybe only had ten employees, imagine how much money goes into setting up their computer system, and getting them a desk, and hooking up their phone system, and changing it so it says their name. Everything. Setting them up with their 401K or benefits, or whatever they have. Like that has got to be at least a couple grand. So, small startups cannot afford that. They need to make the right decision to begin with. And large corporations that have a lot of employees, that can be an enormous cost per year. Hundred of thousands, millions of dollars. I look right across Puget Sound at Amazon.com. They hire thousands of people every quarter because they are growing so fast. Increasing or decreasing their turnover rate from – and I am just making up numbers, if it was – 5 percent down to 2 percent, or 1 percent, would be an enormous benefit. Hard tangible benefit, let alone the soft benefits of like: “Oh, they brought in another under-performer that does not fit the job type that is going to leave in two months because they do not like this culture,” or whatever the factors are. I completely see how that would be a great design of experiments to optimize.
Mark: Yeah, just reading that article can give HR departments ideas on how they can do this. And this was not a formal DOE. It was data collection that they orthogonalized data after the fact, and they were able to home in on the key factors.
Michael: Excellent. So, to somebody who is uninitiated to design of experiments, it seems like you need to be a mathematical genius or maybe a statistics expert to do DOE. Is that the case, Mark?
Mark: Absolutely not. That is another myth out there. I think people get confused over the fact that design of experiments is kind of a fancy statistically related term that blows people away. And we live in 2013 now, Mike. You do not have to be a mathematical wizard. The software does the crunching for us. What does have the happen – the hardest part of DOE is this, Mike. It is the factors and the levels. And the folks that are in the discipline, whether it is HR, finance, or IT – you gave good IT examples from your own business – it is the folks that are experienced in those areas that can determine what factors they should test. Like in the HR Department. I would have never come up with the layoff climate or the upward lateral mobility factor within an organization. I would not have come up with that, but they did. So, did they need me to help them with that? No. The hardest part of DOE is coming up with the factors and the levels. Once you know that, it is a piece of cake to setup the design. Now, randomization and replication – we have to talk about that too, but the orthogonality of the design – those designs are out there, Mike. We do not have to reinvent the wheel. We can be a good driver of an automobile without having to invent the engine, so to speak. So, we live in a society now, and we fully believe that the KISS approach – KISS means keep it simple statistically – is the approach you got to take with DOE, because we have got high school folks doing DOEs at some of our clients. I mean they do not have college degrees. These are high school graduates. They probably had a fairly good algebra background in high school, but they picked this stuff up like it is great. And we are not going to discriminate amongst the mathematical background of people because you can be a good tester – a good experimenter – using DOE without having a heavy-duty mathematical or statistical background.
Michael: Yeah.
Mark: Now, I am not saying that good education is not needed. When you combine – there is that combination effect. You combine process knowledge with education and the tools, like you have in your garage, you will become a much better practitioner and you will make better decisions. And that is what this is all about.
Michael: Okay, Mark, this part of the interview will be hands on. You are sharing your screen right now. The audience can see that you have a presentation document up. What is our first design of experiments example going to be?
Mark Kiemele: Good, Mike. We have two case studies, as Mike has said. The first one is going to be where two companies merged and the director of sales from GlaxoSmithKline. This is when Glaxo Wellcome merged with SmithKline Beecham. This is years ago. So, now they have to combine their sales forces. And this fellow had just taken the Six Sigma DOE portion of the Black Belt training and he says, “Well, I can apply this concept of evaluating these factors independently and looking for interaction effects to combining my sales forces,” so that is exactly what he did. He wanted to do it simple. Obviously there are more than three factors that affect sales, but what I am showing you on the screen right now is an IPO diagram, standing for input-process-output. The output here is sales. Keep in mind that sales is measured in dollars and more dollars is better. Bigger is better for the output. We just want to remember that so that, when we get into the graphs, we remember that bigger numbers are better.
And then the factors he wanted to evaluate are Product Types. He took two of the top product types they had, and then, of course, the Sales Backgrounds are from each of the two different companies. And the Customer Types I am not going to relate, but there are two different customer types. So, the factors that he wanted to investigate were product type, sales background, and customer type, and he has got two levels for each of those. And he wanted to take a look at his sales force, and he had a lot of sales reps, so the beauty of the design I am going to show you, which will turn out to be an eight-run full factorial design because you have two choices for product type, two choices for sales background, and two choices of customer type. So the design I am going to show you, or generate for you, or allow the software to generate for us, is going to end up being a full factorial design, which will allow us to evaluate all of the interaction effects amongst those three as well as the main effects.
Now, the beauty of this. He could have gotten this data, Mike, from just historical data. But the point I want to make is that he randomized his sales reps to each of the eight combinations that I am going to show you. So he had sixteen sales reps for each of the combinations that I am going to show you. So, replicating. He is replicating the design sixteen times, so he is getting data from sixteen different sales reps for each of the eight combinations. So let’s go into the software right now and let me just show you from scratch.
I am going to be using – let me use the sales data and sheet one here. I am going to start from scratch to demonstrate this program that I call DOE Pro. DOE Pro is a very KISS approach to DOE. It allows a practitioner to come in and create a design computer-aided. We let the computer select the design for us. The software will ask us how many levels do you want to test at. Well, the simplest is two levels. You got to test at least two levels. Three-level designs we will not get into today, but two levels, but we have three factors. There were three inputs on that IPO Diagram. They were product type, they were sales background, and then customer type. So we just press next, and then it comes up if you want to let the software. You want to put in the real life factors so you can say, “Oh, Factor A is not A, but it is product type.” So you type that in, you come over and give it the two levels – the low and the high setting – and then you go to Factor B, which is sales. I could just put background in there because background starts with the letter B, but we will just say sales background just to remember what it is. We have two levels there. And C, guess what, starts with customer. Customers starts with C. So customers is Factor C, and we have two different types of customers.
And so, all you have to do is enter the factor names. Their lows and highs. Now, in this case, if we had octane level, it would be 85 or 91. You would be 85 or 91 in there. For tire pressure you might put 25 psi and 35 for the high. So, in this case, it is pretty categorical. These factors here are categorical factors. Product type. They qualitative. They are not quantitative types of factors.
Michael: I understand. So, Mark, I believe you want the low on customer type to be a one?
Mark: Well, thank you very much.
Michael: You bet.
Michael: Okay, good. So we press next, and then it asks how many responses. DOE Pro will handle multiple responses. We only have one response here. It is sales. And he has sixteen reps. Actually these are sales reps, and guess what? Sales reps means replications in this case. So, reps is really replication. So we put this in. Response. We only have one response, and we are going to call that sales. This is measured in dollars of course. And here is your setup.
So the software comes back and says, “Those are your eight combinations you want to test.” This is your design matrix. Right here. It tells you that Test Case Number One, or row number or run number one, is Product Type One with Sales Background One with Customer Type One. And then, of course, you have 16 different reps, so we are going to have 16 responses. Let me just go and I am not going to take the type to put the data in because I have got this already setup, so let me go to the Sales Data Analysis here.
Now I have got the data in. Those are in thousands of dollars. So, when you see a 25 right in here that is to the nearest thousandths of dollars there. So, each of the Y’s – Y1, Y2 – represents a sales representative, which represents actually, in this designed experiment, a replication. So we are getting data from sixteen different reps, each of the combinations here. Okay? So, that is very simple. Actually, this eight-run design for three factors, each at two levels, Mike, is probably the most common design done out there in DOE today, because you do not have a lot of test cases. You have eight test cases. You could evaluate up to three factors in this guy. Each at two levels, and you will get all the interactions. Free of charge. Clean. No confounding. No aliasing. Completely independent evaluations. So that is what we have here.
So, to do the analysis here, there is a couple of things we can do. We can go right to regression analysis, and that is what we are going to do here. That is one way to analyze the data. It is the most typical way. And see, the software does the crunching here. The beauty of getting the regression analysis – you say, “Mark, where are you looking on this complex output?”
Michael: Yeah.
Mark: Well, where I am looking is right here. And if I see red that means that is a significant factor, or a significant interaction. Red, in this case, means your p-value, or the probability of false detection, is very, very small. Less than 0.5. In the cases here, they are all 0.0000. One minus that p-value is your level of confidence. So I can be at least 99.99 percent confident that product type, because I am looking at this guy. Do not worry about the red up here for the constant. That is always going to be red.
Michael: Okay.
Mark: But it is these guys right in here that are significant or not significant. Product Type is. Sales Background by itself is not significant. Customer Type by itself is not significant, as given by the non-red values of the p-value. This guy is significant. AB – that is the interaction of Product Type and Sales Background – is significant. The AC interaction is not significant, but the BC and, believe it or not, there is a three-way interaction, which we rarely see in electromechanical. In people types of processes we will see more higher order interactions, and we are seeing that here on this Sales Process – an ABC interaction.
So, you can look at it this way. There are other diagnostics I can go into. One is this R-squared. R-squared just tells you of all the variation, in those 16 times 8 –what is that? One hundred and twenty-eight data points we saw back here on the Design Sheet. Of all of this data right in here, 90 percent of it is explained by these factors and interactions. That is what it is saying. So that is pretty good. I mean we have used three factors and their interactions to home in on what is important and what is not. So we have got some important interaction effects here, Mike, and another way to look at this is Pareto effects.
The Pareto diagram is always a nice guy to get. Let’s get it for both Y and S. Y means the average. Y-hat means average and S-hat means standard deviation. We do not see anything significant here affecting the standard deviation, which is represented by S. Those coefficients in the S-model were not significant, but if you look at the Y ones there are. If you look at those four, those were the four. Those four bars represent or correspond to the four factors or interaction effects that had significant or red p-values. P-values less than 0.05. And so, Product Type. That is your biggest.
See, the beauty of the Pareto, Mike, is it tells you the relative importance of these factors and interactions as well as the color-coding brings back is it statistically significant. So the Pareto itself gives you a relative indication of the importance of these factors and interactions, and the color-coding tells you then what is statistically significant and what is not. So, folks, do you have to be a mathematical wizard to figure out what is important here and what is not? I do not think so. The click of a button gave me this. The key thing is figuring out what are the factors and the effects, so this is very powerful stuff.
Michael: Yeah.
Mark: Now, what we want to do is we want to get rid of. Before we optimize or look at interaction effects, we want to get rid of the garbage in this model. This guy is garbage. AC. AC is not important. We are going to take it out of the model. All right? Why? Because its p-value is big. It is not significant. Now you say, “Mark, are you going to take out C and B as well?” The answer is no, and here is why. We have a little law called the Law of Hierarchy. I call that the Parental Law that says if an interaction, like AB, is important, you will keep the main effects, A and B, in the model. Okay? We can go into why that is important. You have a BC interaction here. You have an ABC interaction.
So we are keeping A, B and C – the parents of those interactions – whether they themselves or by themselves are significant or not. So, this is going to be our best model here. We saw nothing significant over in the S-hat model. This S-hat over here, so we are taking all this stuff out. It probably would not make any different if we took it out or left it in to be perfectly honest, because nothing is significant there anyway.
And then you re-regress and you get what we call, as George Box would say, the most parsimonious model. By parsimonious, we mean the simplest model that we can get. So, this Y-hat model predicts the center of our distribution of the performance, which is Sales. And S-hat represents the standard deviation, which is pretty constant at about five. That is in thousands of dollars, so that is about five thousand dollars standard deviation. But bottom line is we have got a good model.
Now, let’s look at the interaction plots. There is an analysis tool here called multiple plots that will get you all of the different interactions. Okay? On this sheet, okay? And where you see intersecting lines is where you have an interaction. Okay? Just to make the interpretation of this a little simpler. And the interaction effects between Product Type and Sales Background – this guy right here. These are your main effects right down here. The main diagonal of this tells you: “Look, Product Type was important. It has got a steep slope.” Where the slopes are the deepest that is where your most important effects are coming from.
So let’s home in on this guy. This is the AB interaction. This guy right here. Let’s make this a lot bigger, and then bring it down here so we can see it better. So, we are looking at, right now, the AB interaction. There we go. Now I am going to blow this guy up. Make it a little bigger so you can see it. There. That is a little bigger.
Michael: Yeah.
Mark: And this is called an Interaction Plot Between Product Type, which is on the horizontal axis, and Sales Background, which is the color-coded line. So you have two lines – a black line and a blue line. Black is for Sales Background 1. Blue is for Sales Background 2. Now, what is this intersecting line? What is this guy telling us? It is telling us, if we look at Product 1, what is this best Sales Background to use? Well, remember bigger is better. The blue option over the black is better. That Sales Background 2 for Product 1 is your best choice. That Sales Background 1 right there is a better choice than Sales Background 2 for Product 2.
So, here you have got what is called an interaction plot, where the effect of one factor – namely Product – is influenced by the level of another factor – in this case, Sales Background. So, Sales Background 1 is the better choice for Product 2 right there, and the bigger of the two points on the Product 1 is Sales Background 2. So, it is information like this, Mike, that allows the practitioner – in this case, the Director of Sales – to say, “Hey, we have got maybe an educational issue here on training. Training these different sales backgrounds are coming in from two different companies, and maybe we have got to train, but for right now we are getting the best bang for the buck, basically, by assigning Sales Background 2 to Product 1 and Sales Background 1 to Product 2.”
Michael: So, in a real world example, Mark, Sales Background 2 might have been they started off as a technical sales rep and they knew all the background of the pharmaceuticals before they became a sales rep, and so that allows them to sell Product 1, which is a very technical drug or pharmaceutical stint, let’s say, to doctors, whereas those sales people that did not come from a technical background would not be able to sell those as well.
Mark: Precisely. That is the whole thing. It gives you information and knowledge now of what the potential causes of your increased or decreased sales are; where they are really coming from.
Michael: Right.
Mark: And this is the nature of an interaction effect. These are critical. And with one factor at a time testing, AB Testing, Mike, you are not going to get this kind of information. Guaranteed.
Michael: So, what would the sales manager or director of sales do with this interaction chart, Mark? Might they say, “Okay, now I realize that there is an interaction and different sales people are able to sell more effectively, so I need to split my products up into two different sales forces rather than every sales person selling all of the drugs”?
Mark: Exactly.
Michael: I need to tackle it differently.
Mark: Exactly. That may be a partitioning of your sales force to say, for Product 2, we are only going to have Sales Background 1 sell that guy. For Product 1 we are only going to have Sales Background 2 sell that guy. That is exactly right.
Michael: Okay.
Mark: It allows you to partition and develop a strategy or a policy that allow you to maximize your revenue. That is the whole idea here.
Michael: Okay, that makes sense. So, back on the marginal means of product type, where we have a very steep slope over there, Mark. And it says Series One on the left. It is the upper left-hand graph. That is just a single factor.
Mark: That is a single factor. Just the marginal means of Product Type. Now, notice, Mike, that this guy is pretty steep, right?
Michael: Right.
Mark: And this guy right here, for Sales Background, not steep. It is pretty flat. That means the factors themselves are not significant. And you go back to the Regression Table. There is your Product Type. That was significant, but B and C by themselves – Sales Background and Customer Type – were not significant. Notice their coefficients are very small compared to Product Type. Product Type had the biggest coefficient. And Product Type there is a negative slope, and that is a negative number – the coefficient – and that is reflected in the negative slope of that.
Michael: I understand now. I understand how those are insignificant as single factors, but the interaction is now very significant, and that makes perfect sense in real world and statistical. Let me ask you this, Mark. The R-squared is ninety percent. You can see it .9034. What if my expert team of sales managers did not include the product type factor? They just forgot it and they included five other factors. What would the R-squared be? Might it be like twenty percent or ten percent, and that would indicate to me that like: “Hey, we are missing something here”?
Mark: Well, let’s take it out. Let’s take it out and see. Yeah, your R-squared goes down. Like you take some of this stuff out. Let’s take these two guys out and re-regress and see what R-squared does here, Mike. And we can get that pretty easily and just do the (Unclear 51:28). Look at R-squared. It is down to sixteen percent. If you do not think Product was a significant factor, think again, because now, without it, you are down to sixteen percent.
Michael: Okay. So, when I am doing a modeling like this, I want to make sure that I have at least – what – ninety percent or eighty percent? What is the number that assures me that I have got the right factors in my model?
Mark: Typically, in systems like this, like in sales, electromechanical systems, you want your R-squared to be up there over .7.
Michael: Okay.
Mark: That means you have got about seventy percent of your variability accounted for. If you are a psychiatrist and you are trying to measure or predict the performance of an individual or the behavior of an individual, if you can get an R-squared of .4/.5 and explain forty to fifty percent of the variation in an individual’s behavior that is pretty doggone good.
Michael: Yeah.
Mark: So, it depends on the application, but typically we like to look at seventy percent or higher. And if you do not have ninety percent, like we have, it is an indication we have not homed in on the right factors. Exactly what you said. There are other factors out there that can explain this variation that we are not able to explain right now.
Michael: Yeah. Mark, this seems pretty A-to-Z roadmappy. I understand it. I got my experts to tell me the factors; I defined the two different values, and put it into the system. You software told me what to go collect data on. It ran the numbers. I know what R-squared I would look for. I can tell what the p-value is for the factors, what to eliminate, and then how to look at the interactions so that I can figure out how to change my business; how to setup my sales force so they are more effective, and then I can measure the revenue coming out after doing what my model said to do. The only question I have is around data collection. In this particular example, where we ran the different sixteen replications, do I need to then go into SalesForce.com and quantify all the deals with just one, and then bucketize them into Product Type, Sales Background and Customer Type in order to gather this data? Do I sort of do it retroactively?
Mark: Mike, you can always add new data coming in from your new process to this data and upgrade your model. That is exactly what the folks at Boston Fleet Bank did, and they were HR. They continued. They cut their turnover rate down from fifty percent down to about fifteen percent, but they still had people occasionally leaving the company. They would still get that new data. They would wrap that back in, and use that to build a new model or an updated model. We do the same thing here. As we change our policies and we say: “Okay, if you got Background 2, you guys are going to target Product Number 1. And Sales Background 1, you are going to target Product Number 2,” like we saw in that interaction plot. And now you add that data to this data – the sales data. It becomes more of a historical data analysis. It is not a pure DOE, but you can still update your basic model that you used with the original data.
Michael: Okay, but these are likely manual processes, right?
Mark: Absolutely. Yeah.
Michael: Okay.
Mark: Pretty much manual. And you want somebody who is familiar with the software to put the data in and update the model with the new data.
Michael: All right, fantastic example, Mark. I completely understand how this works and I know how I would take the data and then analyze it and make changes to my business. Let’s go into example two. What example do you have from the medical industry?
Mark: Okay. This is one is something that affects us all, and that is blood testing. Blood analysis. And we all go in, sooner or later, to have blood tests done. And you got to wonder if the results that come back are accurate – are they false positives, false negatives – if we go in. And this comes from Abbott Laboratories in (Unclear 55:26), their Japanese affiliate. So, this came, and now you can see here. They are interested. Their experts say,” Well, they have got a response. It is called a signal.” And this is a signal that they are targeting for sixteen hundred and twenty. They have a machine in this blood test that records the target, or the value of the signal. Their target is 1620 and their specs – they have specs. Now, what are the specs? The 1570 to the 1670 on this normal distribution at the bottom of the page. You will see the specs. The specs are right here, Mike, where the red starts on the left and where the red starts on the right.
Michael: Yeah.
Mark: Those are the specs – at 1570 and 1670. So you can see that you got a lot of red. You got a lot of auto spec, possible false positives, false negatives coming out of this test. That would not hack it, okay?
Michael: Yeah, that is not good. Your DPM – your defects per million – is 571,000. Basically half the test they are running are out of specification.
Mark: Yeah, you got 57 percent defect rate. And you can look at the other stats there, but that is the one I concentrate on; is 57 percent of the area under the curve is red. That is not good. They are not going to solve anything if this any of their blood tests. If this is drug testing, they may be vying for the major league baseball contract. Do you think they will be able to compete? Forget it. They may not be able to complete on this drug-testing contract.
So, they get down into the business and are saying, “Okay, what are our factors?” Now, if you were a subject matter expert that is where we need you. We need you to understand what are the factors that could impact that signal. Well, they came up with seven. Substrate Type, PH, range, and concentration. You can see them there on the left. Those are the factors. They wanted to do a screening design. Now, when you do seven factors, unlike the three we did in the sales example or the director of sales did, 23 is eight possible combinations. If you did that for seven, 27 would be 128 possible combinations. Way too expensive. Takes too long, so what we are going to do – a general rule of thumb is, is that if you have six or more factors, you probably want to screen first before you model.
So, screening is the first thing we will do here, and we can do that with a very simple twelve-run design, which is called an L12 Design. It happens to be a Taguchi 12-Run Design, but it does not matter. Let’s see where is our 12-Run Screen Design. I am going to, Mike, eliminate putting in the data. I am going to tell you the data that they already have.
Michael: Okay.
Mark: Here is the 12-Run Design, which is an excellent design for testing up to eleven. You have the ability to test up to eleven factors in a 12-Run Design. There are really eleven columns, but we are only showing seven because they only wanted to test seven factors. So they are doing twelve test cases. Each of those twelve test cases looks like this.
So, if you took Test Case 2, you are using Substrate Type 1 with a 4.5 PH. Range and concentration at two percent. Mixing time is one minute. Incubation time is also one. The incubation temperature is 120 degrees. And the blood temperature is 100. So that is the combination, and now they did four replications. That is the number of reps, Mike, to have a significant – that is a 95 percent – confidence level in your result. So, the number of replications. We had sixteen before because the director of sales had that number of sales reps to do it. Well, in this test you got to be efficient, but you still have to be effective, and so the number of replications is going to depend on the number of test cases. So we have twelve test cases and we have rules. The software will come up with this automatically and say, “You should be doing four replications.”
So that is what they did here to have 95 percent confidence in their results for both Y and for S – for standard deviation. So this is called a 12-Run Screening Design and its purpose in life is to screen out the main effects. In screening, you are not interested in interactions. Interactions come from modeling designs. This is a screening design. So a very simple, and you mentioned it in the last example. A very simple analysis technique, Mike, is the marginal means plot. You can get that from the raw data.
Let’s get it from both Y-hat and S-hat for the factors that affect standard deviation and the factors that affect the mean. Now, this is a marginal means plot, where all of the marginal means are on the same graph right now, Mike. And do you have to be a mathematical wizard to figure out where the longest lines are?
Michael: You do not have to be a math genius to figure out which one is different from the rest.
Mark: Exactly right. It is number one. It is way over here. It is this guy here. And you know what that happens to be? A qualitative variable called substrate. Substrate Type. These are two different substrates. They are coming from two different vendors. So, which is the better vendor? Which is the better substrate? It is this guy, because in standard deviation, smaller is always better. Smaller is better, so smaller dots are better. The stuff here. When we get the regression results, this guy is going to have a significant P-value, but relatively speaking it is not nearly as important as the first factor there, which is substrate type. The other guys are probably just noise in there. There is no substance there at all.
Now, when you look at the Y – that is for S. Now that is gold. When you discover a factor that shifts your standard deviation, Mike, that is like finding gold.
Michael: Yeah, so that may explain all of the variability of your process right there.
Mark: Exactly right.
Michael: Okay.
Mark: It turns out that it will, but we will get validation of that in the modeling design, okay? So, here is your Y. Now, this one is not so clear-cut. Again, you still do not have to be a mathematical wizard or a rocket scientist to figure what your top three are. That is number one there, and that is the third guy. That is your reagent concentration. Number two is your PH. And number three hitter, as far as length of line segments, is your incubation time.
Michael: Okay.
Mark: So those three factors are the guys that will affect the center or the mean of your output distribution. And the other guys. The S is here. That guy is a big hitter. You have got to control that guy at the setting where you get the smallest standard deviation. That is the bottom line. Now, we can also do, from the Design Sheet, we can get the regression analysis. And we just go in and do the analysis. Not the marginal means this time, but get the regression, and you are going to start seeing red and non-red here. And you can see over here, for the S, the two guys that are red are Substrate Type and Incubation Temp. Those were the two longest lines on the S marginal means plot. You can see, relatively speaking, thirty-one is a lot bigger than eight, but they are both statistically significant, so we got to keep that in mind as well. Not only relatively speaking, but statistically speaking.
Over here, this guy is not important, but the three big hitters are PH, Reagent Concentration, and Incubation Time. So, the screening allows us to understand what are the most important factors. Notice the screening design does not give us information on interactions. To get information on interactions we have to use a modeling design, and that is what we did next, Mike. We went in, picked those top three factors out, and we did a design. Now, this should look familiar to the listener. That is an 8-Run Design just like we did with the good old sales data.
Michael: Yeah.
Mark: The sales data was an 8-Run Full Factorial for Three Factors at Two Levels, and that is exactly what we have here. Three factors, each at two levels. Two times two times two. Eight possible combinations. The number of reps here was five. Remember, in the sales, he had enough reps to do sixteen reps. We are only going to do five reps, but five reps is enough here. Replications is enough to give us the ability to get at least 95 percent confidence in our resulting models. So, that is the design. We can now do the analysis on this guy. We can do the analyzed design. And now, from the modeling design, we get this guy right here.
Now, what do we know? And while we were doing this design, the substrate type and the incubation temperature were held constant at their prescribed or their best settings. So, when we were doing this experiment, the two guys that we found significant in the S-hat model primarily substrate had to be held constant during this experiment. Now, what do we know now that we did not know before? Well, we knew before that PH, Reagent Concentration, and Incubation Time were important. They are still important. The modeling design tells you they are important, so this design actually validates what we saw in the screening design for the mean effects, and it also discovers one other interaction effect. The interaction effect between A and B, which is PH and Reagent Concentration. That is a very strong interaction. These other three guys – this is also knowledge – are not important. The AC, BC, and ABC – the three-way interaction – are not important. So we found information about the interaction effects. Notice how your R-squared bumped up now.
Michael: Yeah.
Mark: We have got over 99 percent. Gosh, I will take that any day of the week we can get 99 percent R-squared. So, over here, nothing is significant. We can go to the marginal means to see that relatively speaking. So, if we go up here and get the marginal means or the Pareto effect, we can get the Pareto of both Y-hat and S-hat. And you do not see anything significant for the S-hat, but for Y you are going to see not only the reagent concentration, PH, and incubation time in the same order – relative order as they were from the screening design – but now you have got this additional information on the AB interaction and the other guys are just insignificant. Okay?
So, basically we have got that. So we have got a good regression model, but we have got to take the garbage out of here, so were are going to optimize. We got to find the setting for PH, Reagent Concentration, and Incubation Time that will get us to our target. So we are going to take garbage out. That is the insignificant terms over there. Nothing is important over here, so we are going to take these guys out as well. They do not tell us too much and there is no significance there. And we are going to regress again. I am just going to go in here and get the parsimonious model. There it is, and now it is going to be on this model that we are going to optimize.
We are going to use this model to find the critical settings that we need to hit a target of 1620. So, to do that, we go to graphs and optimization. We will use the optimizer here. And we do not have multiple responses. We only have one now. The software is now asking me to specify the low and high settings of each of the factors on which we want to allow the software to search for the optimal solution. Well, we are not going to go outside the range that we did when we did the DOE, which this is the low and the highs of a DOE that we did. Going outside would be what we call extrapolation, which can be a bit hazardous if you extrapolate too far. So we are just going to leave the lows and highs here and allow the software to search, in that three space, where is the best combination; can we hit 1620. We say, “Okay,” and we are going to just do a very simple optimization here of say, “Get my Y to 1620 and add that constraint,” and now we optimize, and here are your results right down here.
The results say, “You want to hit 1620, put your PH at 7.4, your Reagent Concentration at 5, and your Incubation Time at 4.96.” We will copy these settings to the worksheet and the software just copied them into our predictor, and now our prediction, under these settings – these experimental settings – is a target of 1620. We should hit 1619.96, which rounded to 1620. Your standard deviation will be that. And here is your 99 percent confidence or risk bounds. So, 99 percent of the time your results should go between 1514 and 1725, hitting a target there.
So we know, with three factors, PH, Reagent Concentration, and Incubation Time, we can put our response variable right on the target. And here are the combinations of the settings that will do that. Notice that all three of them are up towards the high end of the space, or the range, in which PH, Reagent Concentration, and Incubation Time were tested. It is up there close to 7.5 for PH. Close to 5. Exactly 5 for Reagent Concentration. Close to 5 for Incubation time. So, that is what it is. Okay? That will produce. Let me show you if we went in, and told you what it will be from a prediction point of view. The graph – I have that already in this scenario right here. This is what your new scenario would be at the bottom. After the DOE, at those optimal settings, Mike, you will get the Cpk or a prediction.
Now, it is still not the greatest.
Michael: Yeah, I would have thought, based on the DOE in this analysis that we did, that it would have six standard deviations in there. It would be a Six Sigma process, where you only have a few defects per million opportunities, but it is still showing 154,000 defects per million tests run.
Mark: That is right. You are down to a fifteen percent defect rate. Up here it was 57 percent. Down here, the proportion that is red, is fifteen percent. Still not good enough. One DOE that is concentrating on three factors with one factor mainly the substrate type being the variance reduction factor. But the bottom graph, Mike, shows you that we are right on target. The process is centered between the specs, where it is not up above. What is our objective now? We have got to remove more standard deviation.
Michael: Right.
Mark: We have got to make that curve taller and narrower. We have demonstrated with three factors, namely PH, Reagent Concentration, and Incubation Time, that we can put this process on target, but we have got to reduce the variation. Now, Substrate Type. Where do we go for this? Well, we go back to our fishbone diagram or wherever we are to what are some of the other factors that could impact standard deviation. And we have got to search those guys out. We have got to test those. We already have a hint. One of the hints – I do not know if you remember – was Substrate Type.
Michael: Right.
Mark: Now we can go into our vendor. Work with our vendor on that Substrate Type. Probably do a DOE at the vendor’s facility to find out how we can improve that Substrate even more than what it is doing now. We know whatever is in that Substrate Type is causing variance reduction. Can we exacerbate those particular factors and get more information? And this is very typical of DOE. One DOE, Mike, is going to lead to another.
Michael: Yeah. Hey Mark, can we have just said it looks like Substrate Type 2 is better than Substrate Type 1; let’s do a DOE with only Substrate Type 2 and these different factors, and see if it reduces the standard deviation? And then, if it does, then whoever is supplying Substrate Type 1 needs to go solve their own problem. That is not our issue. We fixed our process.
Mark: The second DOE, Mike, was done with Substrate Type 2 held constant at the better setting.
Michael: Okay.
Mark: So we did not find any other factors that reduced variation, so we are still hurting for the factors that are reducing variation. We still have to search those guys out. And actually, they did more screening designs and found variance shifting factors as well, but one of things was that Substrate Vendor Type 2, which we were getting small standard deviations, going back to them and saying, “Okay, what are the major ingredients of this substrate? What can we go and find out as to what might change our standard deviation even further?” Make it even less. So, they worked with that vendor, but they found some other factors as well that reduced the standard deviation even further.
Michael: Excellent. So they not only had it centered on the process, but then they reduced the standard deviation by going to the vendor and helping them analyze the factors that might effect their output of their process and DOEs.
Mark: The output of their process is an input to these guys’ process.
Michael: Right. Exactly.
Mark: That is exactly right, and that is where you get this cascading effect of the propagation of air or the propagation of variability, where the variation coming out of one process is input to the next process. And that is where going back earlier into the life cycle that is of the process – getting back to that vendor of substrate and finding out: “We have got to make this guy better. We already know that there is something in your substrate that is making the variation low. Can we take advantage of that further?” And they did that.
Michael: Excellent. All right, great example, Mark. I have got just a final couple wrap-up questions. People are now exposed to the power of design of experiments. Maybe I want to apply it to iSixSigma.com, which as a Six Sigma practitioner and I have worked in GE and CitiGroup doing Six Sigma in the past, I am a little embarrassed to say that I have not really applied it to iSixSigma.com. Maybe I want to go figure out how to convert more people who visit our marketplace to sell them more project examples or our research that we have done, or maybe I want to convert more visitors to newsletter subscribers. What are my options to learn more and take the next step?
Mark: Well, as you say, Mike, there has got to be a next step. I would recommend education. What I have shown you today is just a couple of examples. One, like you said, from the service or the transactional area in sales; another from the more scientific area – blood analysis. But there are a lot of things that go into this, like randomization, like replications. I said, “We have got to use five reps in this 8-Run Design.” Where did that come from? I mean how many replications do we have to do with a 16-Run Design or with a 32-Run Design? And what if I want to screen fifty-five factors? A little bit of education helps. We have books out there. We have three books. The one that you showed at the beginning of our session – the DFSS: The Tool Guide for Practitioners. The one we did working with you guys. That is the best book as far as learning how to use the software because each of the software steps – what I showed you here today – is described in great detail in the DOE Section of that book. So, if you want to link software to the application that is the best book to have.
We have a couple of other books. The Basic Stats Book that we have and the Understanding Industrial Designed Experiments book. They both have a lot of case studies, like the UIDE. The DOE book has the AIDS case study in it that I mentioned before. They have a lot of case studies that people can, not only the methodology of DOE. Like when you have eight factors, what should you be testing? When you have eight factors at three levels, what design should you be using? Now, you can allow the software to pick the design for you, which it will, but you would want some underlying understanding of why it is picking that design rather that some other design.
So, we have the books, Mike. We have our website. I would encourage anybody to go out and see some of these case studies that are on our website. Many of them are DOE-related. Some are not. Some are success stories without DOE. But DOE is critical. Of all the tools, the methods, Mike, that we have seen in Lean Six Sigma and designed for Six Sigma over the years, DOE brings the greatest return on investment. And that is kind of the point I would like to make. We have the books. We have the software. We entirely recommend that you use a KISS approach. Keep it simple statistically. Use a software package that you can put down for two/three months and pick it up without missing a beat. You need something simple to start with.
Michael: Yeah.
Mark: And I would just say, from a business perspective, look at what your critical performance measures are, and then start looking at the factors that impact those. And I will bet money right now you may be already collecting data on some of those factors. If not, setup a DOE, and do the replicates, and get involved in analyzing the results to find those critical factors.
Michael: All right, Mark, if people want to buy the Basic Stats book or buy the Design for Six Sigma book; not that they have to, but I want to provide the resources to people that want to take the next step and want to learn more, they can do that on your website. And what is that URL?
Mark: www.AirAcad.com.
Michael: Great. And then they can click on products. Now, the software that you used in the example looked very easy to use. It was Excel-based I noticed. It was probably a plugin or an add-on. It is called DOE Pro. Is that correct?
Mark: That is correct. DOE Pro. It is a Microsoft Excel add-in, and it is extremely easy to use. And it uses our rules of thumb to select the design for you and it is extremely easy to use.
Michael: Are you the developer of that software, Mark?
Mark: We are the co-developer. Air Academy Associates and Six Sigma Zone are the co-developers of this software.
Michael: Okay, and they can go to www.AirAcad.com in order to purchase that software as well?
Mark: That is right. They can go there. They can go to that website and then look on software, and that will take you to our Six Sigma Products Groups; is where you would order the software from.
Michael: Okay, here is my question, Mark. I have an attorney that handles my intellectual property issues. If I want to file a trademark with the United States Patent and Trademark Office, I will go to my attorney and I will pay him three hundred bucks an hour to handle that and do it right, because I know he is an expert on that. Does it make sense for me, if I want to optimize my sales process, to hire an expert like yourself or somebody that is an expert in design of experiments for four hours to help me setup the experiment, identify the factors, tell me what data to go collect, and then I go collect the data and then bring it back, and we analyze it together, so I make sure that I am not screwing up, looking at a Y-hat when it should have been an R-hat, or whatever? Does that make sense to do?
Mark: Oh, we do that all the time. We are doing it with one company who has got a problem with one of their products, and they have got millions of dollars of inventory sitting there, waiting to be sold. But they have got a problem they have got to resolve with their customer first. So, we are in there, try to design an experiment right now that will get to root cause. And we have got to find the cause of that. And we will set them up. They will run the test, and then they will bring us back in or call us to help them with the analysis. We will do that, but we recommend that DOE does not have to be that hard. That you develop an expertise within your own company over time so you can do the fishing, so to speak, yourself, and we do not have to do that for you. And DOE does not have to be that complicated. Like I said, you can develop practitioners through a little bit of training to develop that capability internally. Now, will you ever? Even if you are a practitioner of DOE, which I am, I still have to go to the Doc. Over the counter statistics sometimes is not going to do it. When I do a DOE and it is a critical guy, very expensive – 200,000 bucks a shot – I am going to get somebody else to look at that design to see is there something I am missing. Have we missed anything here in the planning stage of this design that I should be considering that I am not considering right now? So, you always need a lifeline. Everybody needs a lifeline, and we can provide that lifeline without training or we can provide that lifeline with training to reduce the dependency on the lifeline.
Michael: Okay. So I can go AirAcad.com and I can use the contact form and say, “I need coaching or mentoring for doing design of experiments in my own company,” and I can hire you guys on an hourly basis to do that.
Mark: Absolutely.
Michael: But I can also sign up for open enrollment training, it sounds like you have, looking at your calendar on your website, or I believe that we have them on our events calendar on iSixSigma.com as well. The listing of courses. You do offer a one-week course on design of experiments, where you can teach people how to fish themselves, so that you do not have to give them the fish. They are not paying you for the fish. You are teaching them how to use this tool themselves.
Mark: Absolutely. We think it takes about five days to show them not only two-level designs we talked about, but three-level and then also mixed-level. Talk about historical data analysis from a DOE context. It takes about five days to get them up to be a practitioner. And that is about what it is in our Lean Six Sigma and DFSS curriculum too. It is about a weeklong class really just focusing on design of experiments, because one DOE, Mike, can save the cost you cannot believe of all of the waste that is generated by a particular process. Just finding an interaction effect or a factor that reduces variation. At GE Corporate R&D, I know you worked at GE, but maybe not in Corporate R&D. When they saw these screening designs, like that 12-Run Design, those PhD chemists said, “Hey man, I did not realize we can test that many factors, looking for these factors that shift the standard deviation.” Yeah, you can do it, and you can do it quite effectively and efficiently with a few number of runs. It does not take that many test cases.
Michael: Yeah, all right. And so, regardless of how people learn, if they already have a background in statistics and they watched us run through these examples, and they want to pay forty dollars or whatever it is for the book to have an example to go through, they can buy the software, they can maybe buy the book, and they can go off and do it. For people that need a little bit more assistance, they can hire you as consultants. The people that want to learn how to fish themselves can go to a five-day training course and learn all the tools and the full educational background themselves. So, we are offering a whole different variety of options. We are not saying that any one is correct for anybody watching this show. But if people have a follow-up question, you can go ahead and post it in the comments below the video and we will ask Mark to come back and answer as many as he can. Air Academy Associates is on Twitter of course. Their Twitter handle is @AirAcademyAssoc. I will have a link to their Twitter account just below the video as well. Mark, if someone wants to contact you directly to ask a question, maybe they are too embarrassed to ask it in a comment or they just want to reach out to you, how can they do that? Is there a preferred email address?
Mark: Yes, email address. You can send it to [email protected]. That is the email address and that will get to one of our DOE practitioners if I am not there. So, [email protected], or they could call us at (719) 531-0777.
Michael: Great, and we will have that in the transcript below as well. I am going to urge the audience right now. If you received value out of this interview, please take a moment to say thank you to Mark. This is as easy as posting a comment below the show, following Air Academy on Twitter, sending a tweet out, saying thanks Mark, and there will be a link just below the video saying I got a lot of value out of this. Tell your friends. Tell your colleagues. Mark has taken a couple hours plus in preparation out of his time to give back to the community, and I think that he has given enough to get a lot of people moving in the right direction so that they are not wasting time and money in their business; that they are optimizing their processes. So, thank you, Mark, for doing that.
Mark: Thank you, Mike. Thanks for having me.
Michael: You bet.
Dr. Mark Kiemele, president and co-founder of Air Academy Associates. Thank you from iSixSigma for coming on the show, sharing your knowledge of optimization and design of experiments, and helping others become successful change agents and business leaders
Mark: Thank you, Mike. It has been a pleasure.
Michael: Thank you all for watching. We’ll see you next time.
Watch the full video at:
https://www.isixsigma.com/tools-templates/design-of-experiments-doe/mark-kiemele-interview/