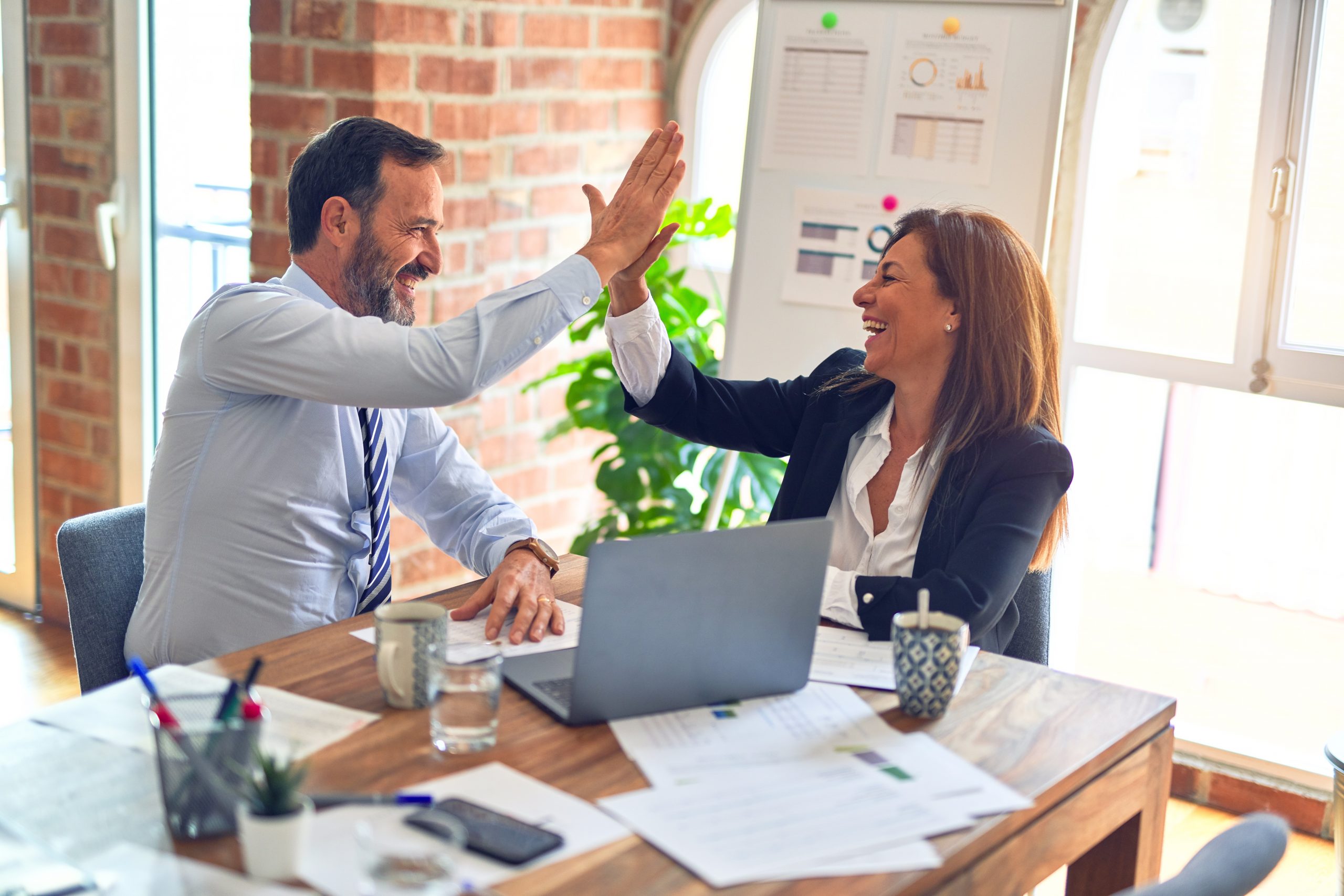
While reading about type I & II errors and specifically beta-risk I realised that although I was happy with alpha-risk I didn’t recall seeing beta-risk in any of our sampling or hypothesis equations. Being curious, I wondered why I was only accounting for type I errors in my work?
Just to review, we need to be aware of and manage our risks when running a hypothesis test. We term the potential errors as Type I & II and they can be quite insidious:
- Type I – False Positive – You call a difference when there isn’t one
On March 23rd 1998, Professors Fleischmann & Pons announced they had achieved sustained thermonuclear reactions in a test tube. They believed they had created Cold Fusion. Unfortunately it was not to be. - Type II – False Negative – You do not call a difference when there is one
The effects here can be devastating, such as releasing harmful new drugs or missing production defects in car-tyres. Imagine a control chart showing no issues while bad product is being pumped-out?
Our (abbreviated) process for hypotheses testing being:
a. Define Ho
b. Set the alpha-risk (normally 95%)
c. Calculate p-value for the hypothesis
d. Accept or reject Ho
No mention of beta-risk here. I reviewed assorted materials to get up to speed and discovered all about beta-risk. Here is the concept (not the math), big disclaimer, I might be wrong or have missed something.
Imagine you draw a sample from a process and get an estimate for the mean and confidence interval. You decide the process needs a touch of improvement. You draw a sample from the new process and get the mean and confidence interval. Where the beta-risk comes into play is the when looking at the potential degree of overlap of the second sample with the first.
In the first picture (below) the red & green lines show the respective samples 95% confidence intervals. The two sample distributions are miles apart. Not much beta-risk here.
In the second picture (below) the two distributions overlap. So for any given sample from B there is a probability it will be in the zone to the left of the red-line, the 95% confidence interval of sample A, hence accepting Ho when the two means are different.
The shapes of the sampling distributions are governed by the sample size, alpha and standard deviation and have not given you the resolution to see the change in mean. The distributions are too wide. So the beta-risk is the probability of drawing a samplefrom Bin the region left of the red-line, within the 95% interval of sample A.
You can find the beta-risk for any given hypothesis test in Minitab under Stats–>Power & Sample Size. If you find you have a low score, The Power of the Test, you may wish to change your key parameters, sample size, and alpha, to improve the sampling resolution.
My conclusion on why beta-risk does not appear more prominent in our manuals is because we look for “big improvements”. If our process improvement has shifted the mean by a small-margin (so that beta-risk applies) then its not much of an improvement. Bit of a generalisation and am sure there must be exceptions.