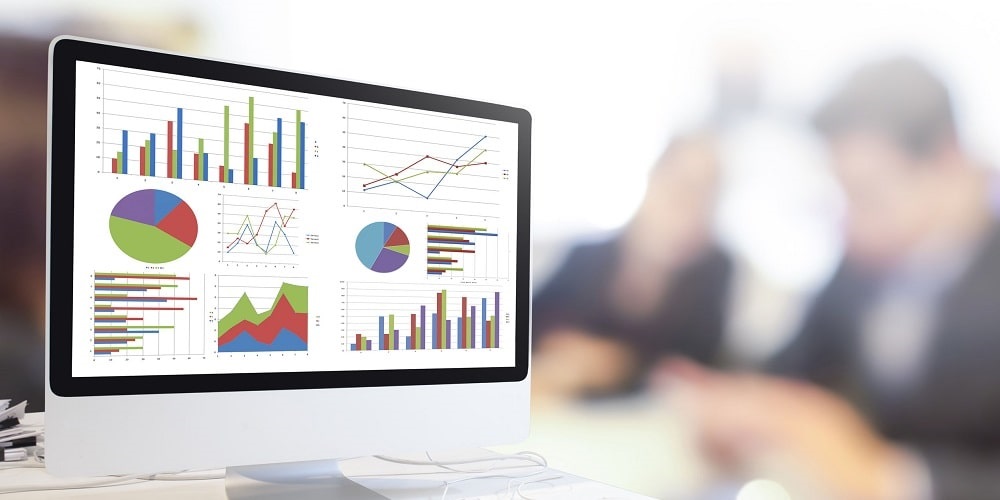
Background
In a highly competitive market, a plastic parts company leveraged Lean Six Sigma methodologies, particularly Process Capability Analysis (Cpk and Ppk), to analyze supplier performance and strategically improve their product offering. By selecting the most capable supplier and tightening component specifications, the company not only outperformed competitors but also created value for its customers, who were willing to pay a premium for higher quality and reduced variation.
This case study details how a Lean Six Sigma Master Black Belt (MBB) conducted the analysis and the strategic decisions that followed, providing sample data and visual insights.
The Challenge
The company relied on three suppliers to provide a key product component for a high-demand plastic part. However, variation in the component’s quality was causing challenges, including:
- Increased internal rework and scrap rates.
- Customer dissatisfaction due to inconsistent product performance.
- Loss of potential customers to competitors offering tighter specifications.
To address these issues, the company sought to:
- Analyze the process capability of all three suppliers.
- Select the most capable supplier based on Cpk and Ppk values.
- Negotiate tighter specifications with the chosen supplier to enhance customer satisfaction and differentiate their product in the market.
What They Did
Step 1: Data Collection — The MBB collected data on a critical dimension of the component from all three suppliers. A sample size of 100 measurements was taken from each supplier, ensuring consistency and statistical validity. The target dimension was 50 mm, with the following tolerances:
- Upper Specification Limit (USL): 50.20 mm
- Lower Specification Limit (LSL): 49.80 mm
Step 2: Check for normality of the data and do a control chart to test process stability. These are common underlying assumptions for doing process capability analysis.
Step 3: Process Capability Analysis — The collected data was analyzed using statistical tools to calculate the Cpk (process capability index) and Ppk (process performance index) for each supplier. These indices measure how well the process meets specification limits and accounts for both process variation and centering.
Step 4: Vendor Selection and Specification Tightening — The supplier with the highest Cpk and Ppk values was selected. The company then negotiated tighter tolerances to differentiate their product, ensuring the supplier could maintain capability under the new requirements.

©Ground Picture/Shutterstock.com
Sample Data
Below is a summary of the data collected:
Supplier | Mean (mm) | Standard Deviation (mm) | Cpk | Ppk |
Supplier A | 50.05 | 0.050 | 1.00 | 0.98 |
Supplier B | 50.02 | 0.020 | 2.67 | 2.60 |
Supplier C | 49.98 | 0.035 | 1.57 | 1.50 |
Observations
- Supplier B had the highest Cpk and Ppk values, indicating excellent process capability and performance.
- Supplier A barely met acceptable limits, while Supplier C showed moderate capability.
Outcomes
- Supplier Selection: Supplier B was selected as the primary supplier due to its superior process capability and consistent performance.
- Specification Tightening: The company negotiated tighter tolerances with Supplier B:
- New USL: 50.15 mm
- New LSL: 49.85 mm
Supplier B’s process still demonstrated a high Cpk (2.0) under the tighter specifications, ensuring reliable performance. Action was also taken to reduce the mean dimension of 50.05 to the nominal desired mean of 50.0 mm.
- Customer Value Creation: By reducing variation and tightening specifications, the company marketed their product as having higher precision and reliability. Customers valued this improvement and were willing to pay a 10% premium, increasing revenue and customer loyalty.
- Beating Competitors: Competitors struggled to meet the tighter specifications, giving the company a significant edge in the market.
How AI Can Enhance Vendor Process Capability
Artificial intelligence (AI) is transforming how companies assess and improve the process capability of their vendors. By leveraging AI-powered tools, organizations can gain deeper insights into vendor performance, optimize quality, and enhance overall supply chain reliability.
AI enables continuous monitoring and analysis of production data collected from vendors. Advanced algorithms can analyze key metrics, such as defect rates, process variation, and adherence to specifications, in real-time. By identifying patterns and anomalies, AI helps pinpoint the root causes of inefficiencies, enabling vendors to implement corrective actions swiftly.

©Golden Dayz/Shutterstock.com
Predictive analytics powered by AI can forecast potential deviations in vendor processes before they occur, minimizing the risk of non-conformance. Additionally, machine learning models can simulate process improvements and recommend optimal configurations to enhance process capability (Cpk) and performance (Ppk).
AI-driven quality control systems, such as computer vision, can inspect components for defects more accurately and consistently than manual methods, ensuring vendors meet stringent quality standards. Furthermore, AI facilitates transparent communication by generating detailed reports and visual dashboards, fostering collaboration between companies and their vendors.
By incorporating AI into vendor management, companies can ensure their suppliers deliver higher quality products, maintain consistent performance, and support the organization’s goals for operational excellence and customer satisfaction.
Final Thoughts
Through the strategic use of Process Capability Analysis and collaboration with a high-performing supplier, the plastic parts company:
- Improved product consistency and quality.
- Enhanced customer satisfaction and loyalty.
- Gained a competitive advantage by offering a superior product.
- Increased revenue because of premium pricing.
This case study demonstrates how Lean Six Sigma tools like Cpk and Ppk can drive business success by aligning process improvements with customer expectations and market demands.