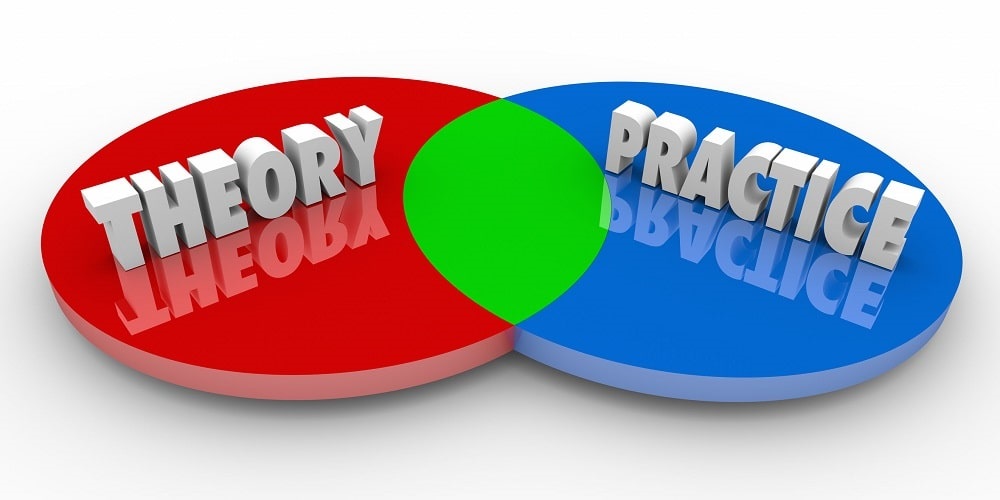
When I was a teenager, I took a SCUBA diving certification course. For the first few lessons, I sat in a classroom with the other students and learned a lot about critical concepts like nitrogen in the bloodstream, proper equipment usage and emergency procedures. We eventually progressed to a shallow swimming pool where we tried the basics of breathing underwater and how to handle hazardous situations that may arise while diving, most of which involved variations on not having air available to breathe.
Finally, we proceeded to the open water test of our skills. In one of my first demonstrations of applying this knowledge, I was asked to take off my mask and then remove my regulator from my mouth and toss it behind me. Next, I had to successfully recover both – while remaining under 40 feet of water. In my first attempt, I managed to get my removed mask tangled with the regulator and snagged on another piece of equipment behind me. In the classroom, I was trained to reach for my second regulator or signal to my diving buddy that I needed assistance.
In practice, I attempted to bolt for the surface and had to be held down by the instructor to save me from a dangerous consequence of rapid ascent.
Classroom Versus Reality
Learning statistical tools in a Lean Six Sigma (LSS) course more often than not follows the same fate: what seemed perfectly reasonable and logical in the classroom fails to account for real-world complexities. Learning those tools has little value if the student is inclined to immediately abandon them when the situations they face do not look like those the instructor showed. As a result, Belts gravitate to simpler tools within their comfort zone that often fail to deliver breakthrough results on complicated problems.
Critical to Statistical Analysis
To bridge this gap, consider the elements necessary for effective statistical analysis as shown in Figure 1.
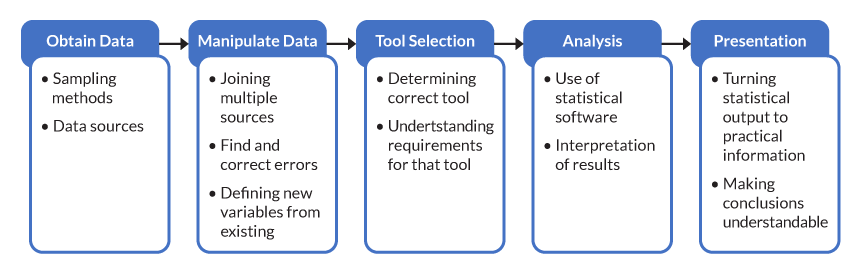
This is not an exhaustive list, but I consider these five categories to be fundamental to doing things correctly. Now consider where the majority of time is spent during Belt training: tool selection and analysis. With little-to-no formal training in collecting and preparing data for analysis or presenting it to an audience once completed, is it any wonder Belts don’t feel confident?
Statistical Tool Training Requirements
To make training more effective, I recommend several practices as part of training in statistical tools.
1. Use real data. Clean training datasets that avoid the problems of real-world data may make it easy to focus on the tool being taught, but they avoid the realities of using that tool in practice. By using real data sets in class, data manipulation tools and quality checks are incorporated into the analysis. Instructors can further gain enormous credibility for the power of the tools by allowing students to bring their own data and then working through it “live fire” in front of the class.
2. Formal training in data collection and presentation. You are probably aware of the catastrophic results of engineers working on the Challenger space shuttle not being able to clearly communicate the results of their data analysis. Converting technical information to understandable graphical presentation and verbal communication is a skill; if Belts are to be successful, they need to be taught – and have the opportunity to practice – that skill. The same goes for data collection: understanding how databases work and best practices for manual data collection is a hard skill that should be taught extensively – not glossed over.
3. Test with open-ended problems. An exam, especially at the Black Belt level, should reflect expected competency following the course. In that spirit, questions asked should not direct the student to the appropriate tool to use or ask specific questions with obvious answers. Rather, providing a dataset (preferably real data) or situation and requiring the candidate to determine the best course of action will best determine whether they can apply knowledge outside of strict tool usage.
4. Practical application. Incorporating an actual problem to solve in the course provides context and an opportunity to go through the end-to-end process of using the tool. This is additional work for the instructor, who must identify an opportunity ahead of time and line up resources and backing to attack the problem as part of class. But the potential value to students, who now work together through all five elements of statistical analysis to achieve a real result, is potentially the most significant of all activities in the course.
As an example, consider using these practices as part of learning design of experiments (DOE). The five elements, learned and applied to a real designed experiment performed as a class, might look like what is shown in Figure 2.

The Value of Real-World Experience
The questions posed in Figure 2 are not comprehensive but provide an idea of the kinds of problems and considerations a student should learn to respond to when they are executing their own project. By teaching mostly tool selection and analysis in class, instructors aren’t preparing students for what to expect after class and how to handle it. Thus, the student ends up lacking confidence when their project doesn’t seem to produce “clean” data analysis opportunities as were presented in class. The students then gravitate away from using them.
Excuses for why statistical analysis was not appropriate for a project include statements like “my data was not normal,” “the process was not in control” and “there wasn’t even data on this problem.” These are followed by face-saving statements intended to make the practitioner sound like they did the right things by not using data, such as “this was more of a process-oriented project” and “the company isn’t really ready for those types of tools and is more Lean-focused right now.” Of course the company isn’t ready to be using these tools – that’s why they trained only specific individuals in those skills! And you can be sure that the organization is in full support of problem-solvers using the tools that get the best results.
My dive training experience taught me valuable lessons that I have taken with me on more than 50 dives, including one in which another diver knocked my mask off in 80 feet of water. Having undergone a real-world test on my training, I was able to stay calm and take appropriate action that resulted in not only retrieving my mask but continuing the dive.
There is no replacement for real-world experience, but these practices begin to incorporate that experience in the learning process. By ensuring better balance across all elements of statistical analysis, instructors can better prepare students to stay calm in the face of unexpected obstacles and not “bolt for the surface.”