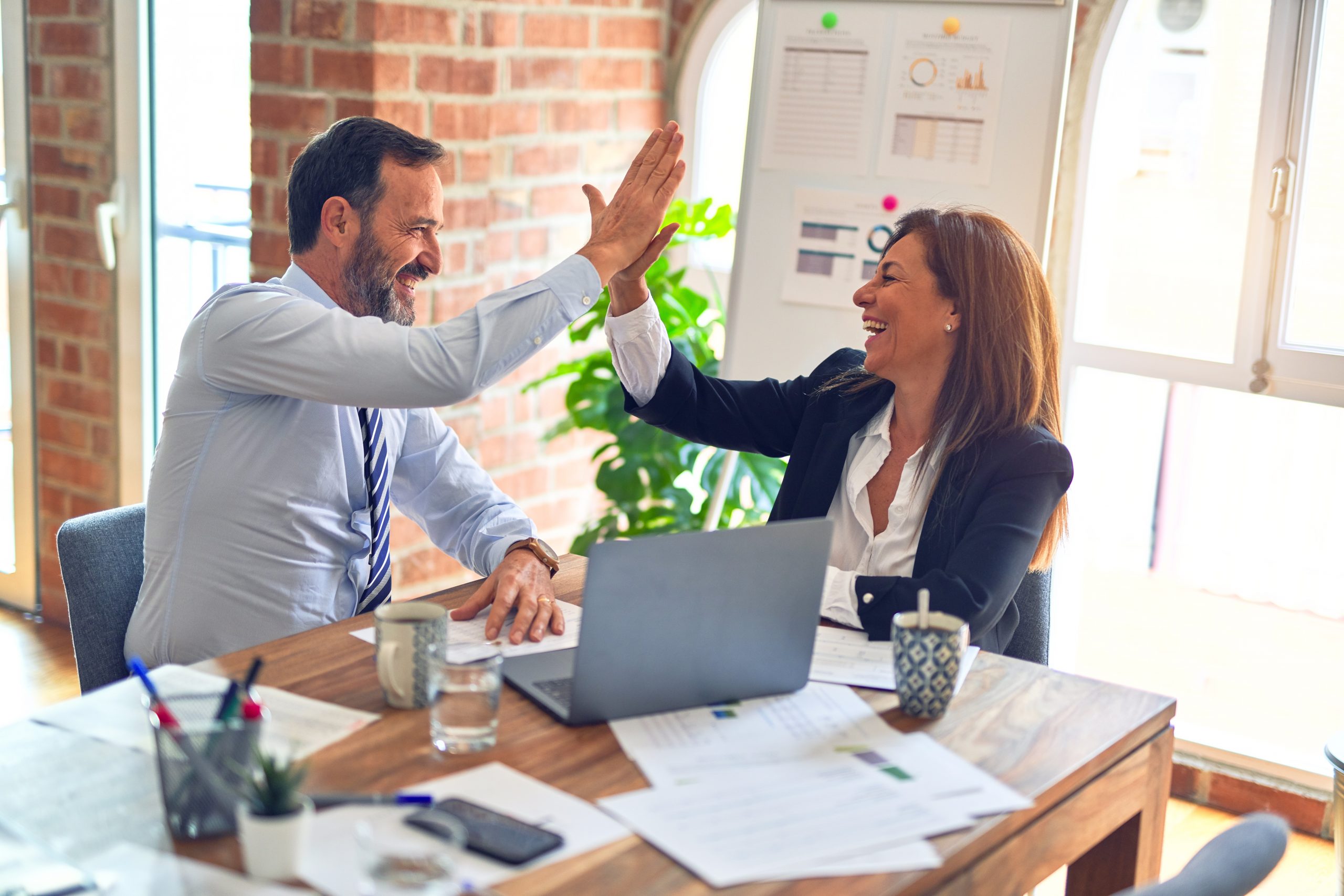
The various costs of an information technology (IT) project is forecast at the beginning of the fiscal year and usually re-forecast at the end of each month based on the actual spending pattern. Variation in the IT project forecasting process can result in either underspent or overspent budgets. As is true in most processes, variation is not good. A Six Sigma approach applied to IT project forecasting process minimizes forecast accuracy variance resulting in dollar savings for the business.
The importance of correct forecasting is easy to understand. In the case of underspending, new technology projects are not undertaken because funding did not appear to be available at the beginning of the fiscal year. In the case of overspending, the technology leadership team has to scramble to arrange for additional funds and the organization may have to shortchange other projects. Improving the capability of the IT project forecasting process so that the total project cost incurred is within specified limits of forecasts at post-Analyze sizing will improve technology’s overall revenue and recovery position.
IT Project Forecasting Process
The technology organization of a major financial institution based in the United States, in which project managers estimated costs in the project start up phase and re-estimated them at the end of each phase of the project life cycle, provides a good subject for a case study. Alignment between the business partners and project managers happened at the end of each month based on actual spending and the re-forecasts. The project managers updated the forecasts in the project management tool and baselined forecast accuracy variance at the end of each month using the re-forecast values.
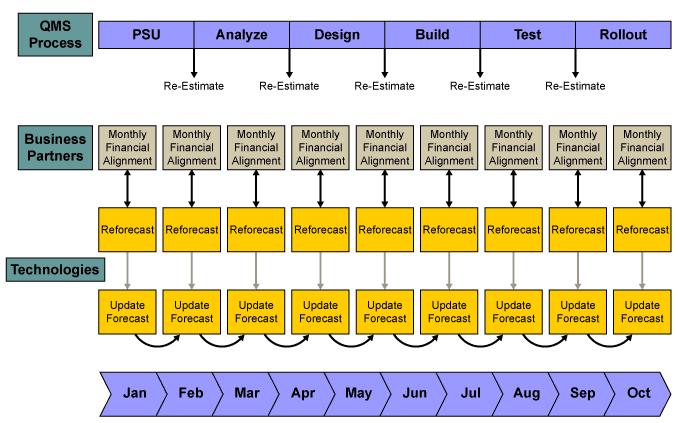
The IT project forecasting process improvement project was executed by a company technology team and consultant. They evaluated the IT project forecasting process performance and improved the same by leveraging Six Sigma expertise. Six Sigma DMAIC (Define, Measure, Analyze, Improve, Control) rigor was used in executing the project. The company team was responsible for implementing the Control plan/forecasting process improvement framework and verifying the success measures.
CTQs Are an Eye Opener
Voice of customer (VOC) was collected by conducting interviews and focus groups with the stakeholders from a key portfolio of the company’s technology organization. Level 2 and Level 3 as-is process maps were prepared for IT project forecasting process. Internal customer critical-to-quality characteristic (CTQ) was derived from the VOC data, and the project CTQ was determined using a CTQ drill-down tree. Customer and project CTQs were defined as:
Customer CTQ = Percentage post Analyze sizing accuracy = (Percentage variance of post-Analyze sizing and total project cost / the post-Analyze sizing)
Project CTQ = Percentage monthly average forecast accuracy = (Difference between monthly forecast and monthly actual spend in the post-Analyze phase / the monthly forecast)
With the current practice, project managers reported lower forecast accuracy variance to senior leadership team since the baseline was done using re-forecast values at the end of each month, which were accurate as compared to the forecasts at the post-Analyze sizing. The suggested CTQ baselines the variance using forecasts at the post-Analyze sizing uncovering the hidden variance. The new practice brought focus on the ability of the project managers to perform better forecasts at the post-Analyze sizing.
Analysis of Underspend and Overspend
Data analysis indicated that 57 percent of the projects were underspend cases while 28 percent were overspend cases when compared with post-Analyze sizing. Process capability of monthly forecast accuracy with a specification limit of +/- 5 percent was poor and the observed CPK was negative. Process capability of forecast accuracy with respect to post-Analyze sizing with +/- 5 percent specification limit also was negative. Median of forecast accuracy for projects with a dollar value less than $50,000 was smaller than that of projects with a dollar value greater than $50,000, indicating better accuracy in forecasting process costs of small-sized projects. The level of variability, however, was the same for both low- as well as high-dollar value projects.
The project costs included hardware, resource, third party software and miscellaneous costs. Detailed brainstorming sessions were conducted by the project team to determine root causes for inaccurate estimates, underspend and overspend situations for each project cost component. Trend analysis of the cost variance categories indicated underspend cause categories as 1) less effort realized, 2)unregistered billing for external receivables, 3) administrative issues, and 4) inaccurate forecasts registered. Similarly the overspend cause categories identified were 1) additional effort realized, 2) unplanned billing registered, 3) administrative issues, and 4) inaccurate forecasts registered.
Industry benchmark data indicated that 60 percent%†of the IT projects failed in terms of cost and schedule compliance, according to the book Information Technology Project Management by Kathy Schwalbe. Only one out of five IT projects were likely to be compliant on cost, scope, quality and schedule parameters. Also data indicated that larger the project size, the more likely was the failure on cost and schedule compliance. Based on industry benchmark reports, estimation standards, current performance baseline and agreement of the project team, the performance objectives were determined.
Monte Carlo Simulation and FMEA
Based on the current trend, the likenesses of different causes were analyzed. A probability distribution function for each cause category was determined based on the historical frequency data. Forecast accuracy results were then captured by running a Monte Carlo simulation for each of the cause categories. Factors related to less effort realized, unregistered billing and administrative issues were found to be vital Xs for the underspend variance situation. Simulation could not be performed for overspend variance due to lack of confidence in the probability distribution function determined for these cause categories. It was concluded that factors related to overspend situation were not vital since there were a fewer number of overspend cases reported.
Since there could be other unreported causes, a system and component a failure mode and effects analysis (FMEA) was performed to completely identify and prioritize causes of the project cost variance. Assessment of as-is risk was done using FMEA. Key causes with a risk priority number greater than 120 were identified. All the identified vital Xs impacting forecast accuracy variance were classified using a control impact matrix.
Action Plans and a Framework
Detailed future-state process maps were prepared. The high- and medium-impact action items were categorized based on the effort, then detailed action plans were developed for each stakeholder group including the business partners, the technology leadership team, project managers, tools group, hardware vendors and third party software vendors.
A forecasting process improvement framework with focus on requirements management, resource management, schedule management and administrative issues was suggested to eliminate sources of variation and achieve forecast accuracy variation within the +/- 5 percent specification limit.